Interpretability for reliable, efficient, and self-cognitive DNNs: From theories to applications
Neurocomputing(2023)
摘要
In recent years, remarkable achievements have been made in artificial intelligence tasks and applications based on deep neural networks (DNNs), especially in the fields of vision, speech, text, and multimodal analysis. The learning of DNNs is not only the process of abstracting essential laws from data but also the result of nonlinear fitting from massive high-dimensional data. However, the architecture, operation mode, and learning ability of DNNs are still far from those of human brain neurons, and the calculation and reasoning are extremely complex, making the model's analysis and interpretation crucial. To free DNNs from their dependence on complex structures and massive data, a lot of related works toward the interpretability of DNNs have been proposed. In this review, we elaborate on the definition of model interpretability from the three perspectives of model reliability, feature efficiency, and self-cognition. The interpretability theory of DNNs is summarized from four aspects: model adversarial attack and defense, feature representations, information and geometry, and causal counterfactual. In addition, we categorize the interpretable methods involved according to typical application scenarios. Finally, we discuss the research goals that have not yet been achieved. We sincerely hope that our work will benefit the field and attract more researchers to devote their energy to the interpretability of DNNs, thereby pushing for-ward the long-term development of artificial neural networks and artificial intelligence. (c) 2023 Elsevier B.V. All rights reserved.
更多查看译文
关键词
Deep Neural Networks,Interpretability,Explainability,Adversarial attacks,Attribution,Information geometry,Causality
AI 理解论文
溯源树
样例
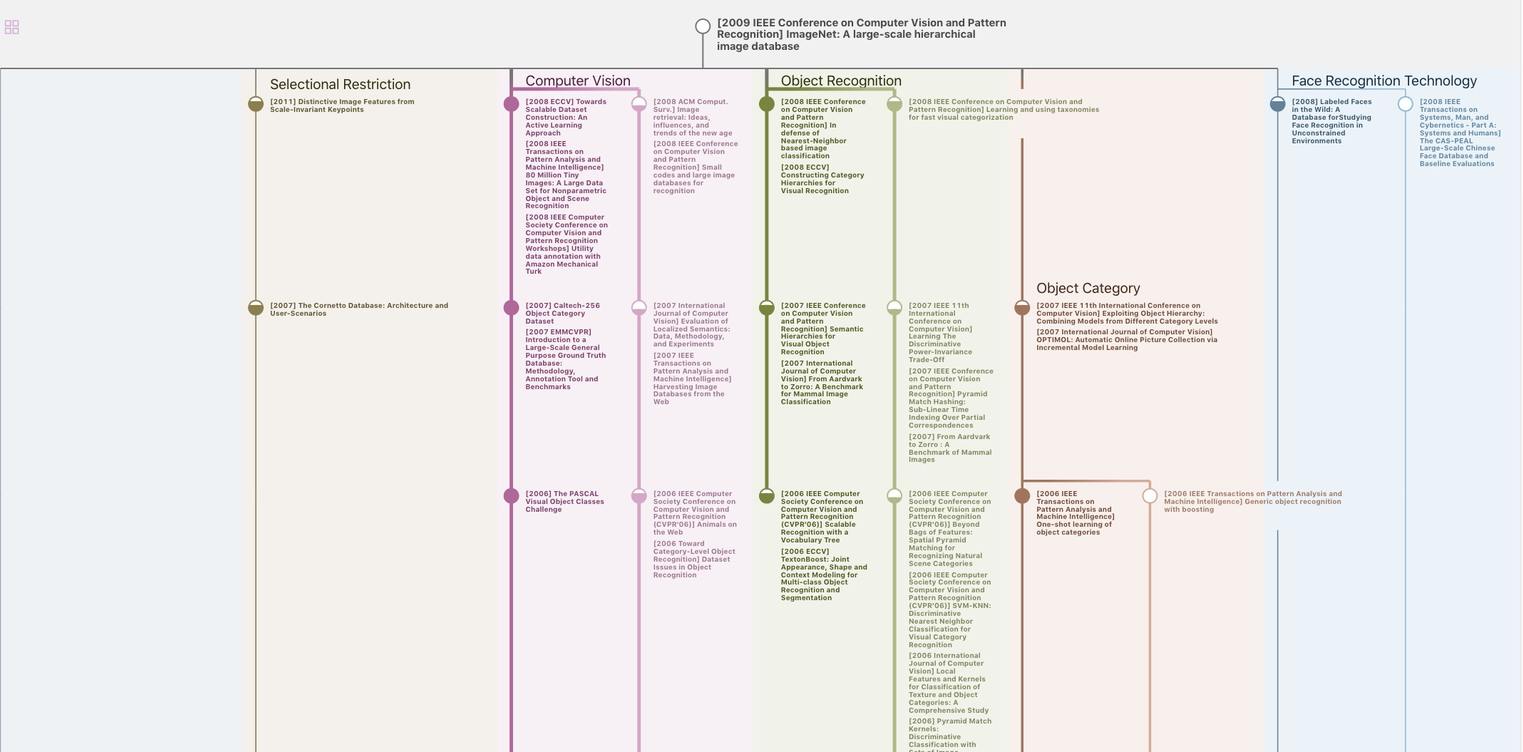
生成溯源树,研究论文发展脉络
Chat Paper
正在生成论文摘要