Harmony: Heterogeneous Multi-Modal Federated Learning through Disentangled Model Training.
MobiSys(2023)
摘要
Multi-modal sensing systems are increasingly prevalent in real-world applications such as health monitoring and autonomous driving. Most multi-modal learning approaches need to access users' raw data, which poses significant concerns to users' privacy. Federated learning (FL) provides a privacy-aware distributed learning framework. However, current FL approaches have not addressed the unique challenges of heterogeneous multi-modal FL systems, such as modality heterogeneity and significantly longer training delay. In this paper, we propose Harmony, a new system for heterogeneous multi-modal federated learning. Harmony disentangles the multi-modal network training in a novel two-stage framework, namely modality-wise federated learning and federated fusion learning. By integrating a novel balance-aware resource allocation mechanism in modality-wise FL and exploiting modality biases in federated fusion learning, Harmony improves the model accuracy under non-i.i.d. data distributions and speeds up system convergence. We implemented Harmony on a real-world multi-modal sensor testbed deployed in the homes of 16 elderly subjects for Alzheimer's Disease monitoring. Our evaluation on the testbed and three large-scale public datasets of different applications show that, Harmony outperforms by up to 46.35% accuracy over state-of-the-art baselines and saves up to 30% training delay.
更多查看译文
AI 理解论文
溯源树
样例
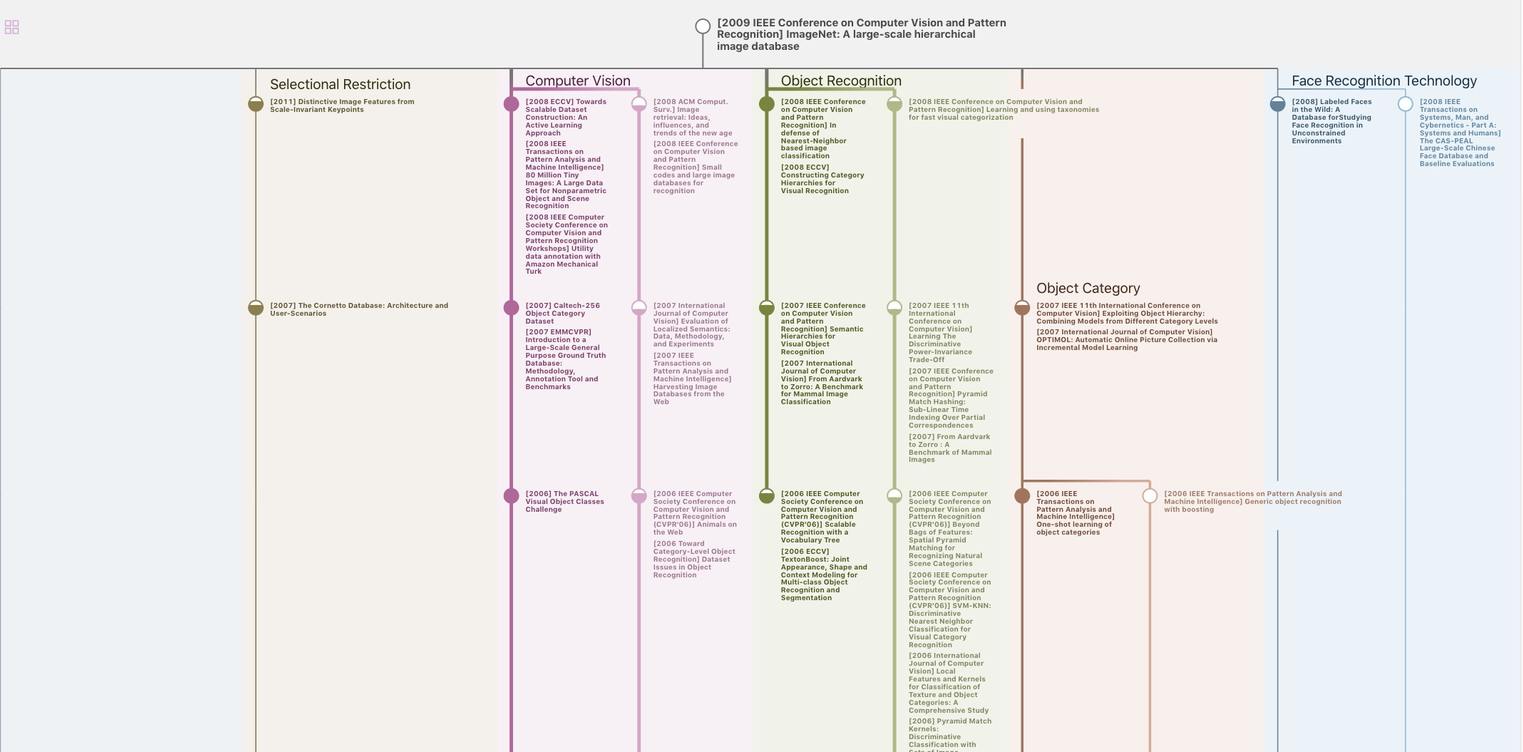
生成溯源树,研究论文发展脉络
Chat Paper
正在生成论文摘要