The Multiscale Maximum Change Algorithm for Subsurface Characterization
Computational Science and Its Applications – ICCSA 2023 Workshops(2023)
摘要
The characterization of subsurface formations is a formidable task due to the high dimension of the stochastic space involved in the solution of inverse problems. To make the task computationally manageable, one needs to apply a dimensional reduction technique. In this paper we are considering the Karhunen Loève expansion (KLE) as the aforementioned technique. Considering the subsurface properties of interest, such as permeability and porosity, it may be suitable to localize the sampling method so that it can better accommodate the large variation in rock properties. In a Bayesian framework we investigate the solution of an inverse problem involving an elliptic partial differential equation for porous media flows. We propose a new multiscale sampling algorithm in which the prior distribution is expressed in terms of local KL expansions in non-overlapping subdomains of the domain of the inverse problem. We solve the inverse problem using multiple Markov Chain Monte Carlo (MCMC) simulations performed on a multi-GPU cluster. The simulation results indicate that the proposed algorithm significantly improves the convergence of a preconditioned MCMC method.
更多查看译文
关键词
Preconditioned MCMC, MCMC convergence, Inverse Problem, Multiscale Sampling, Domain Decomposition
AI 理解论文
溯源树
样例
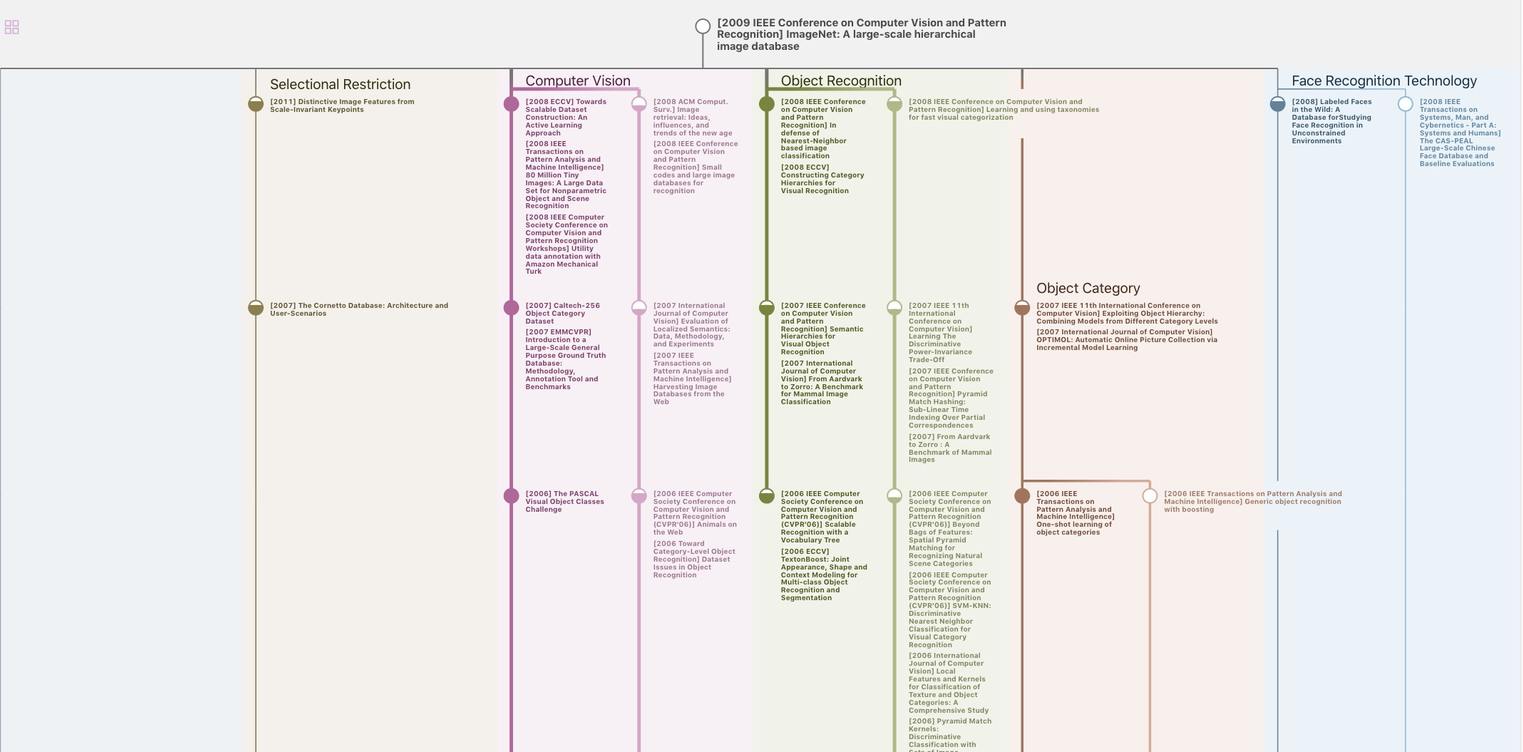
生成溯源树,研究论文发展脉络
Chat Paper
正在生成论文摘要