Utilizing Machine Learning Algorithms for Effectively Detection IoT DDoS Attacks
Proceedings of the 2023 International Conference on Advances in Computing Research (ACR’23)(2023)
摘要
One of the most critical features of the Internet of Things (IoT) is that it allows a massive number of devices to communicate with each other at the same time, for example (cars, smoke alarms, watches, glasses, web cameras, etc.). Given that they can connect to the Internet, the huge demand to convert systems into smart systems that can be controlled remotely increases the chances of cyber-attacks that threaten security and availability becoming more frequent, severe, and in a different form. Distributed Denial of Service (DDoS) attacks have become the most common threats in IoT. These attacks make IoT systems unreliable because complex DDoS attacks cannot be detected by traditional security countermeasures such as firewalls and intrusion detection systems (IDS). In this research, we proposed a model dedicated to work on prediction and detection of DDoS attacks on networks IoT, where we run this model on a NF-BoT-IoT-v2 dataset, which contains 37,763,497 records divided into five categories (Benign, Reconnaissance, DDoS, DoS, and Theft), and then we process our dataset to be more business friendly so it only has two categories (100,000 records broken down into 23,906 benign attacks and 76,094 DDoS attacks). This study used WEKA and MATLAB tools to perform the classification efficiently and find the most important features through feature selection. We used a decision tree (J48) machine learning classifier, a Naive Bayes (NB) classifier, and a random forest (RF) classifier. However, the results were as expected and improved accuracy and efficiency.
更多查看译文
关键词
Internet of Things (IoT), Cyber Security, DDoS Attack, Machine Learning (ML), Attack Detection
AI 理解论文
溯源树
样例
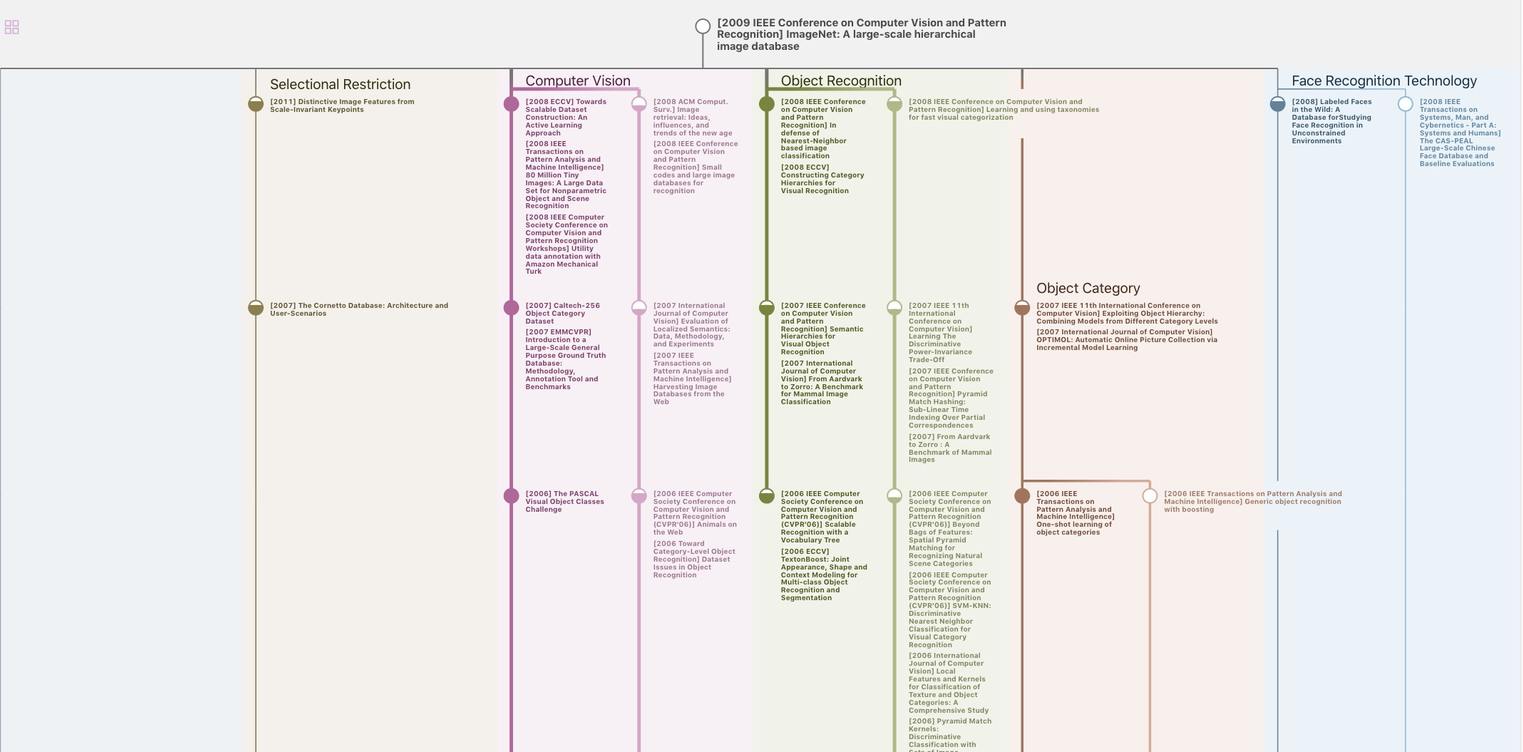
生成溯源树,研究论文发展脉络
Chat Paper
正在生成论文摘要