In Situ Framework for Coupling Simulation and Machine Learning with Application to CFD
CoRR(2023)
摘要
Recent years have seen many successful applications of machine learning (ML) to facilitate fluid dynamic computations. As simulations grow, generating new training datasets for traditional offline learning creates I/O and storage bottlenecks. Additionally, performing inference at runtime requires non-trivial coupling of ML framework libraries with simulation codes. This work offers a solution to both limitations by simplifying this coupling and enabling in situ training and inference workflows on heterogeneous clusters. Leveraging SmartSim, the presented framework deploys a database to store data and ML models in memory, thus circumventing the file system. On the Polaris supercomputer, we demonstrate perfect scaling efficiency to the full machine size of the data transfer and inference costs thanks to a novel co-located deployment of the database. Moreover, we train an autoencoder in situ from a turbulent flow simulation, showing that the framework overhead is negligible relative to a solver time step and training epoch.
更多查看译文
关键词
coupling simulation,situ framework,machine learning
AI 理解论文
溯源树
样例
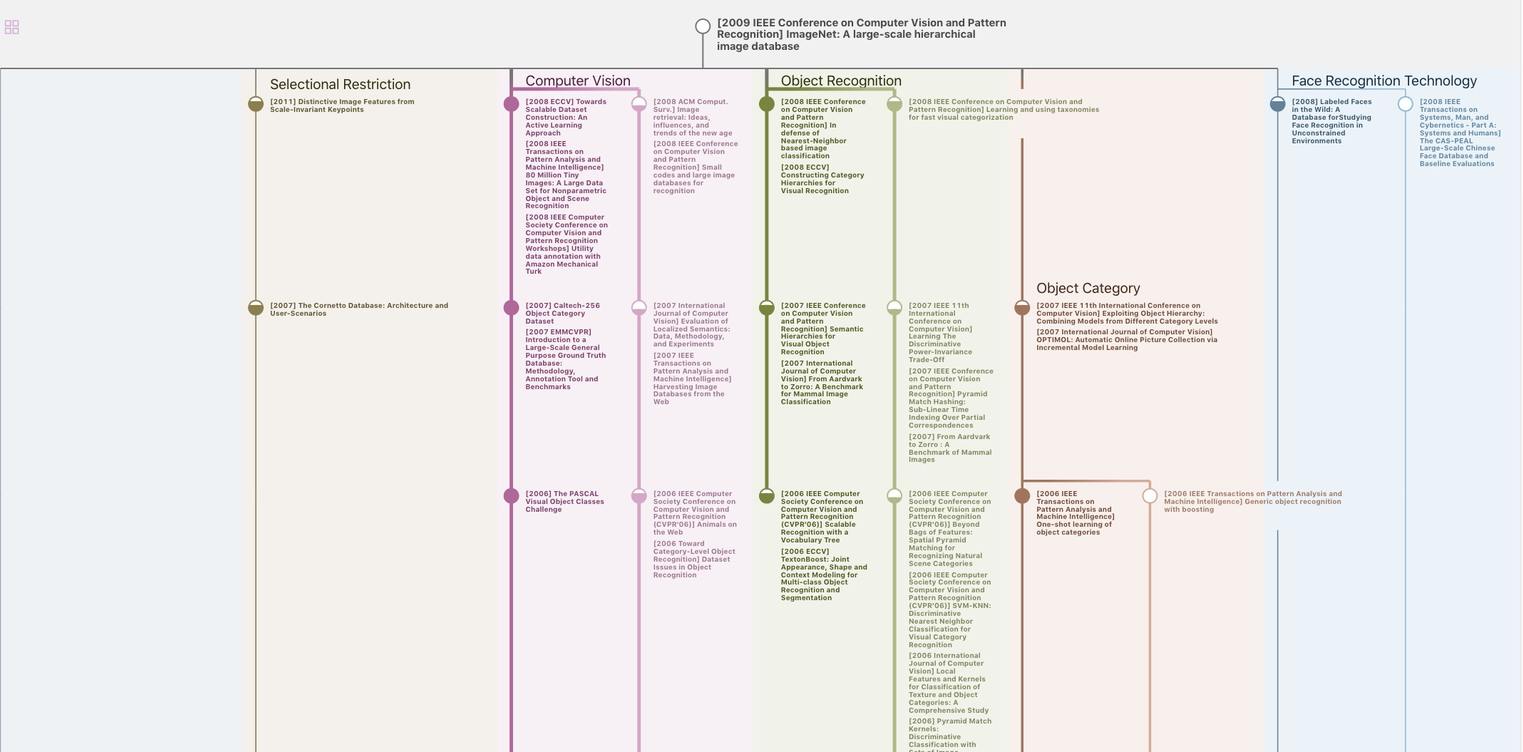
生成溯源树,研究论文发展脉络
Chat Paper
正在生成论文摘要