Deep Reinforcement Learning with Task-Adaptive Retrieval via Hypernetwork
CoRR(2023)
摘要
Deep reinforcement learning algorithms are usually impeded by sampling
inefficiency, heavily depending on multiple interactions with the environment
to acquire accurate decision-making capabilities. In contrast, humans rely on
their hippocampus to retrieve relevant information from past experiences of
relevant tasks, which guides their decision-making when learning a new task,
rather than exclusively depending on environmental interactions. Nevertheless,
designing a hippocampus-like module for an agent to incorporate past
experiences into established reinforcement learning algorithms presents two
challenges. The first challenge involves selecting the most relevant past
experiences for the current task, and the second challenge is integrating such
experiences into the decision network. To address these challenges, we propose
a novel method that utilizes a retrieval network based on task-conditioned
hypernetwork, which adapts the retrieval network's parameters depending on the
task. At the same time, a dynamic modification mechanism enhances the
collaborative efforts between the retrieval and decision networks. We evaluate
the proposed method across various tasks within a multitask scenario in the
Minigrid environment. The experimental results demonstrate that our proposed
method significantly outperforms strong baselines.
更多查看译文
关键词
multitask episodic memory,deep reinforcement learning,hypernetwork,task-conditioned
AI 理解论文
溯源树
样例
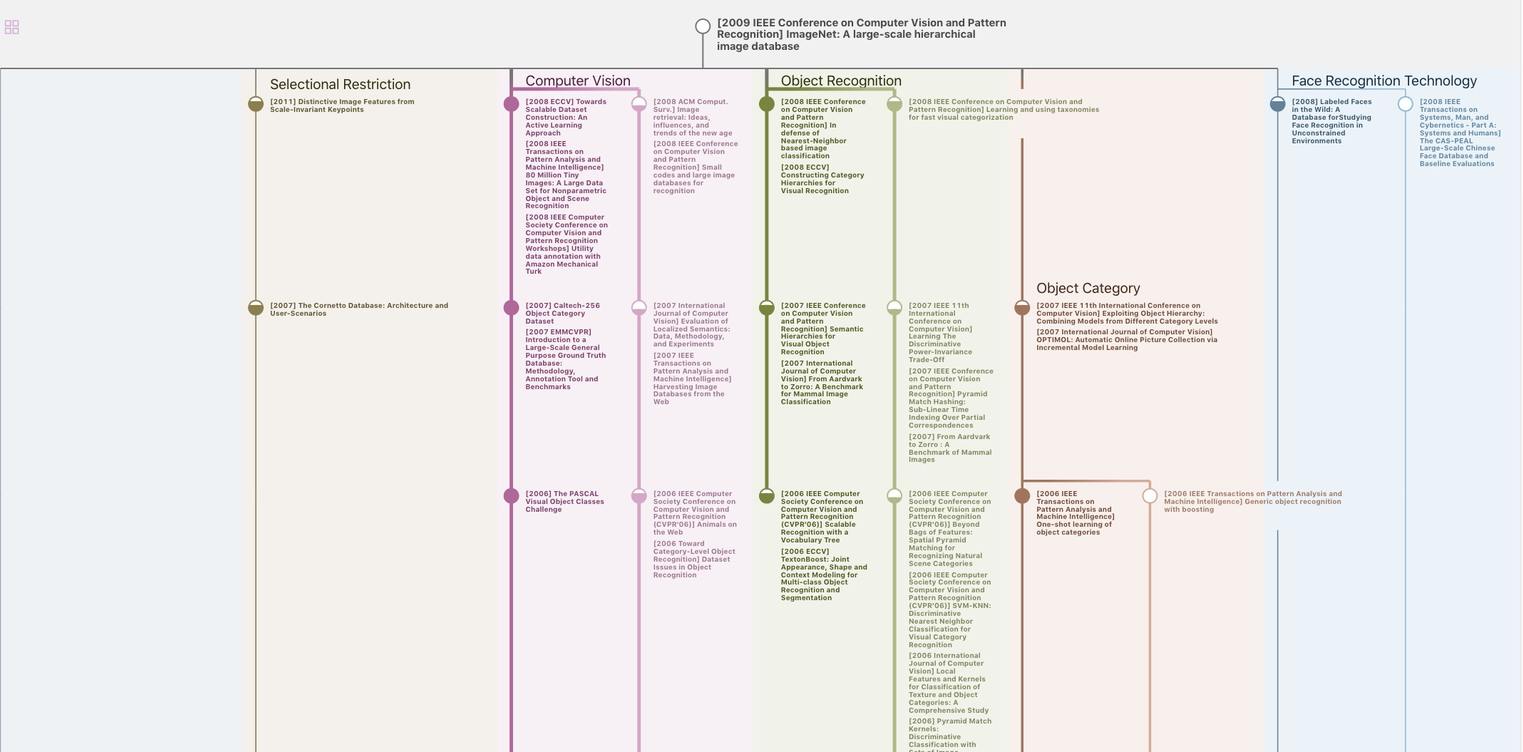
生成溯源树,研究论文发展脉络
Chat Paper
正在生成论文摘要