Knowledge Transfer for Dynamic Multi-objective Optimization with a Changing Number of Objectives
CoRR(2023)
摘要
Different from most other dynamic multi-objective optimization problems (DMOPs), DMOPs with a changing number of objectives usually result in expansion or contraction of the Pareto front or Pareto set manifold. Knowledge transfer has been used for solving DMOPs, since it can transfer useful information from solving one problem instance to solve another related problem instance. However, we show that the state-of-the-art transfer algorithm for DMOPs with a changing number of objectives lacks sufficient diversity when the fitness landscape and Pareto front shape present nonseparability, deceptiveness or other challenging features. Therefore, we propose a knowledge transfer dynamic multi-objective evolutionary algorithm (KTDMOEA) to enhance population diversity after changes by expanding/contracting the Pareto set in response to an increase/decrease in the number of objectives. This enables a solution set with good convergence and diversity to be obtained after optimization. Comprehensive studies using 13 DMOP benchmarks with a changing number of objectives demonstrate that our proposed KTDMOEA is successful in enhancing population diversity compared to state-of-the-art algorithms, improving optimization especially in fast changing environments.
更多查看译文
关键词
optimization,knowledge,dynamic,transfer,multi-objective
AI 理解论文
溯源树
样例
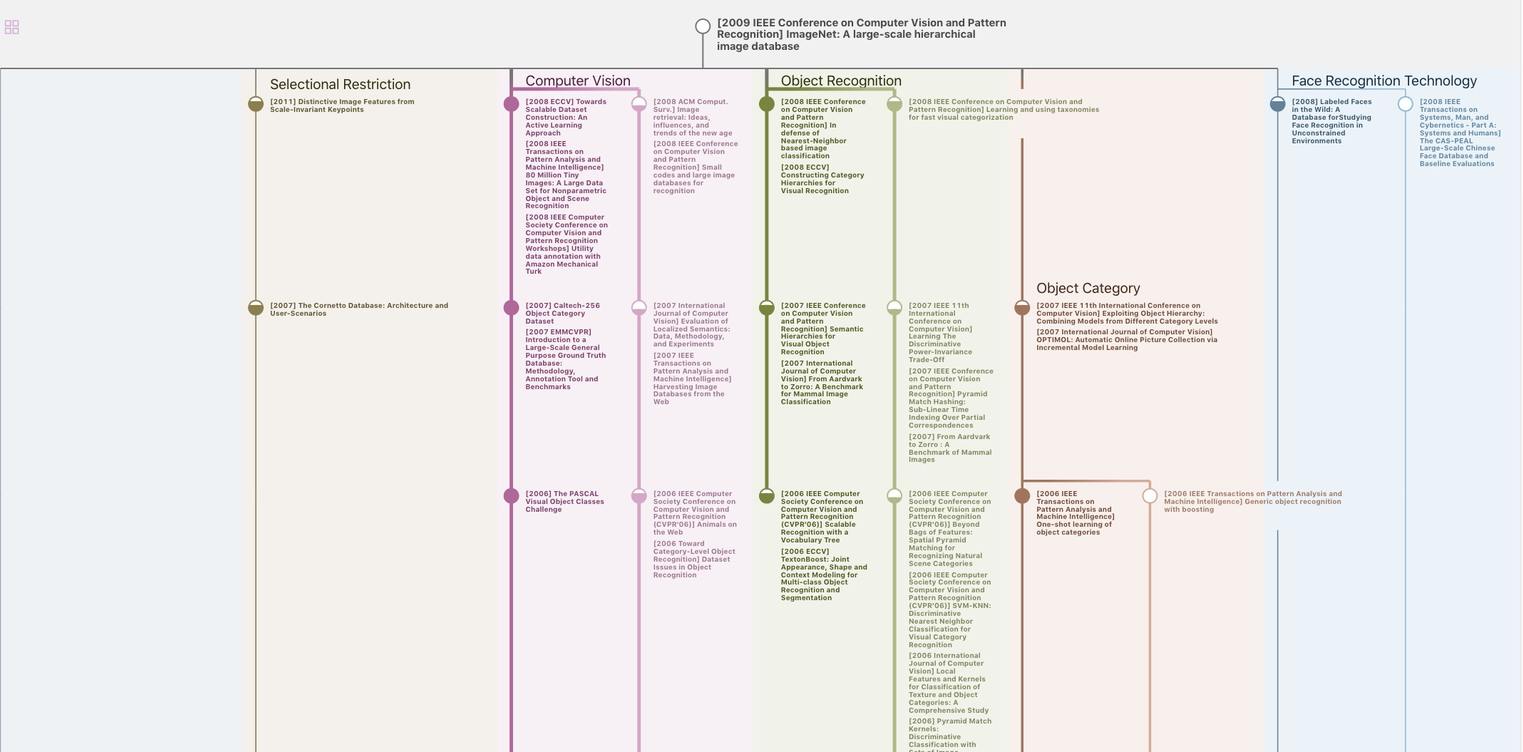
生成溯源树,研究论文发展脉络
Chat Paper
正在生成论文摘要