Design of peptide inhibitors targeting β-catenin using generative deep learning and molecular dynamics simulations
Research Square (Research Square)(2022)
关键词
peptide inhibitors,molecular dynamics simulations,generative deep learning
AI 理解论文
溯源树
样例
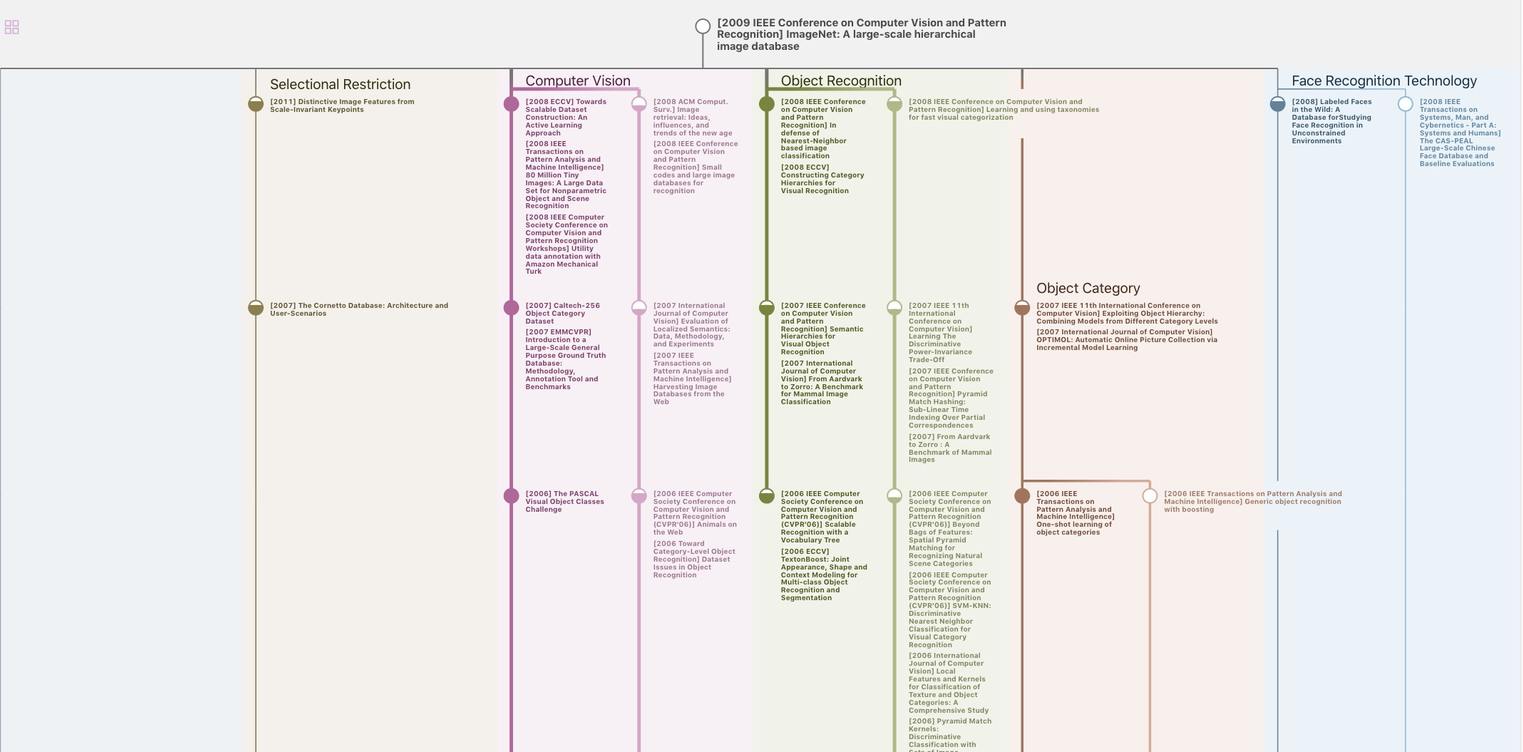
生成溯源树,研究论文发展脉络
Chat Paper
正在生成论文摘要