Learning heterogeneous delays in a layer of spiking neurons for fast motion detection
crossref(2022)
摘要
Abstract The response of a biological neuron depends on the precise timing of afferent spikes. This temporal aspect of the neuronal code is essential in understanding information processing in neurobiology and applies particularly well to the output of neuromorphic hardware such as event-based cameras. However, most artificial neuronal models do not take advantage of this minute temporal dimension. Inspired by this neuroscientific observation, we develop a model for the efficient detection of temporal spiking motifs based on a layer of neurons with heterogeneous delays which we apply to the computer vision task of motion detection. Indeed, the variety of synaptic delays on the dendritic tree allows to synchronize synaptic inputs as they reach the basal dendritic tree. We show this can be formalized as a time-invariant logistic regression which can be trained using labeled data. We apply this model to solve the specific computer vision problem of motion detection, and demonstrate its application to synthetic naturalistic videos transformed into event streams similar to the output of event-based cameras. In particular, we quantify how the accuracy of the model can vary with the total computational load. This end-to-end event-driven computational brick could help improve the performance of future Spiking Neural Network (SNN) algorithms and their prospective use in neuromorphic chips.
更多查看译文
AI 理解论文
溯源树
样例
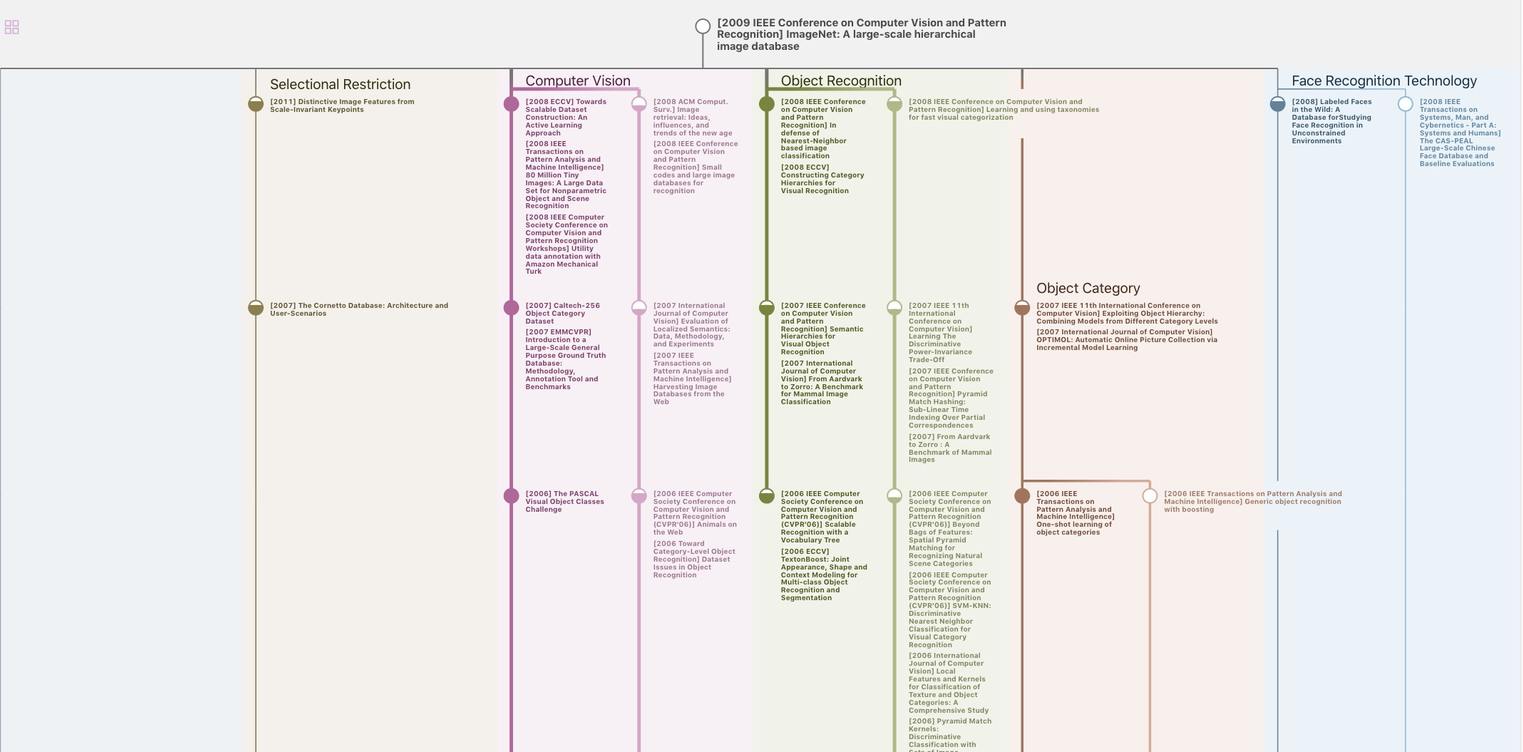
生成溯源树,研究论文发展脉络
Chat Paper
正在生成论文摘要