Deep Learning for High-Resolution Wildfire Modeling
Advances in Forest Fire Research 2022(2022)
AI Read Science
Must-Reading Tree
Example
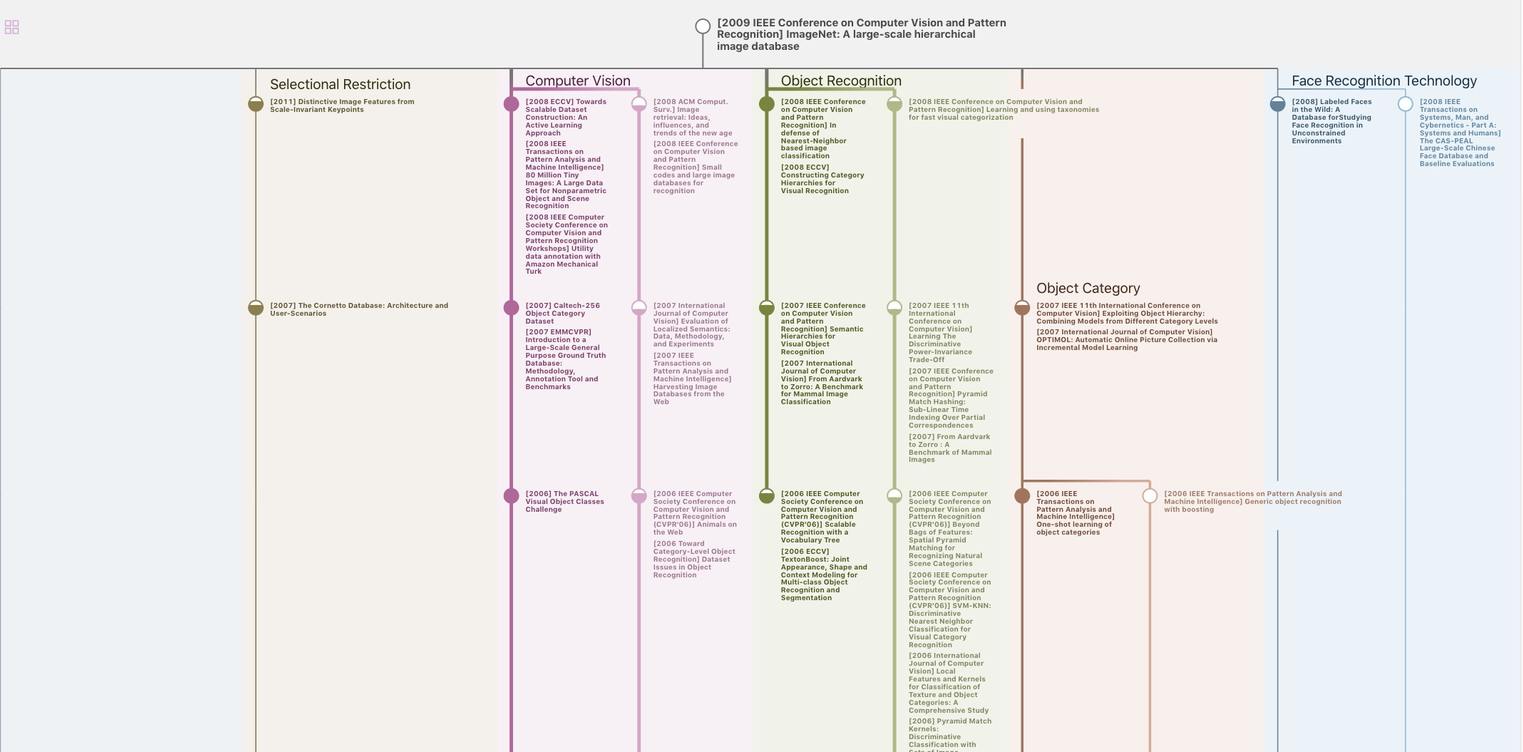
Generate MRT to find the research sequence of this paper
Chat Paper
Summary is being generated by the instructions you defined