Landslide Susceptibility Evaluation and Interpretability Analysis of Typical Loess Areas Based on Deep Learning
Natural Hazards Research(2023)
关键词
TabNet,Landslide susceptibility evaluation,Frequency ratio,Self-supervised TabNet,Interpretability analysis
AI 理解论文
溯源树
样例
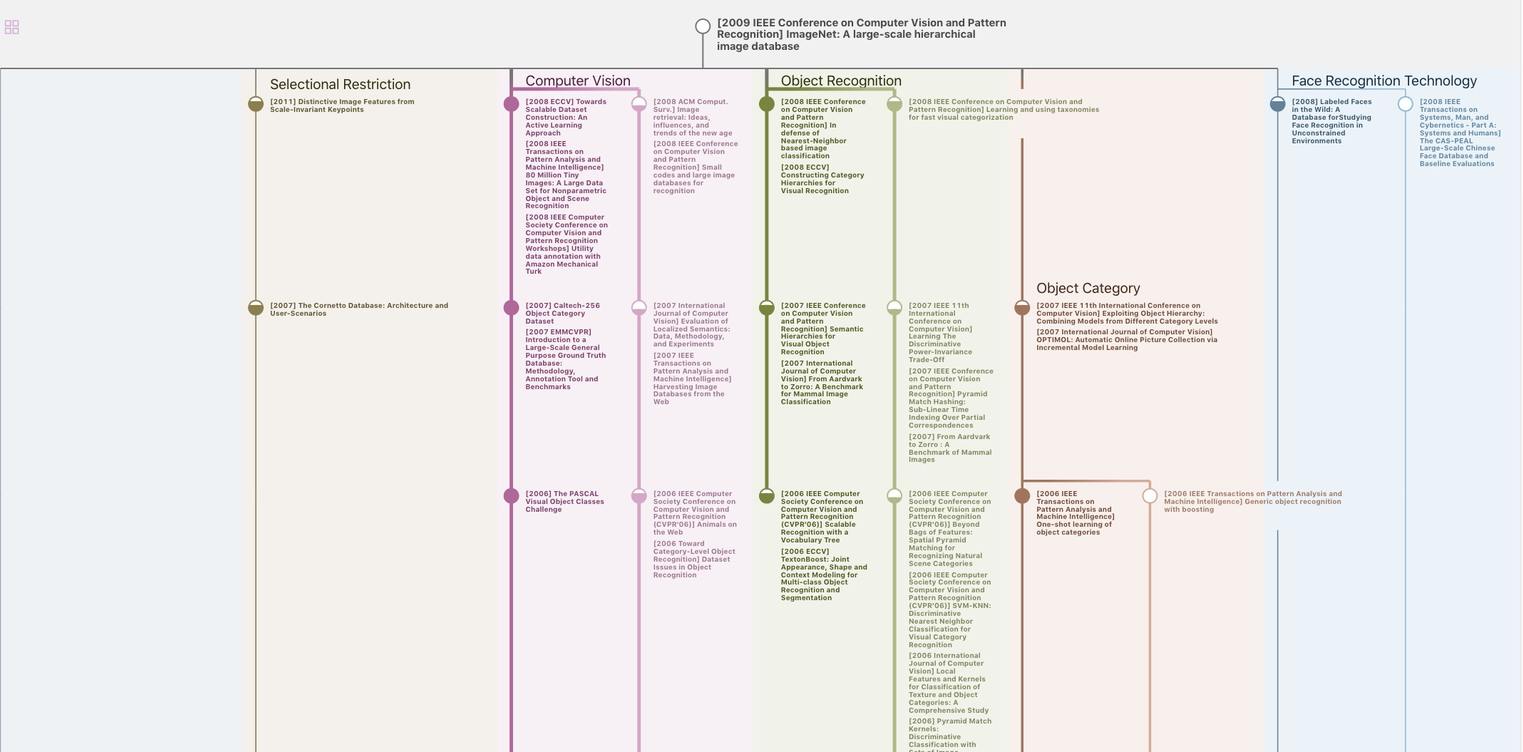
生成溯源树,研究论文发展脉络
Chat Paper
正在生成论文摘要