1657. Real-life Implementation of a Machine Learning Based Algorithm for Urinary Tract Infection Antibiotic Resistance Management in the Community Setting
Open Forum Infectious Diseases(2022)
AI 理解论文
溯源树
样例
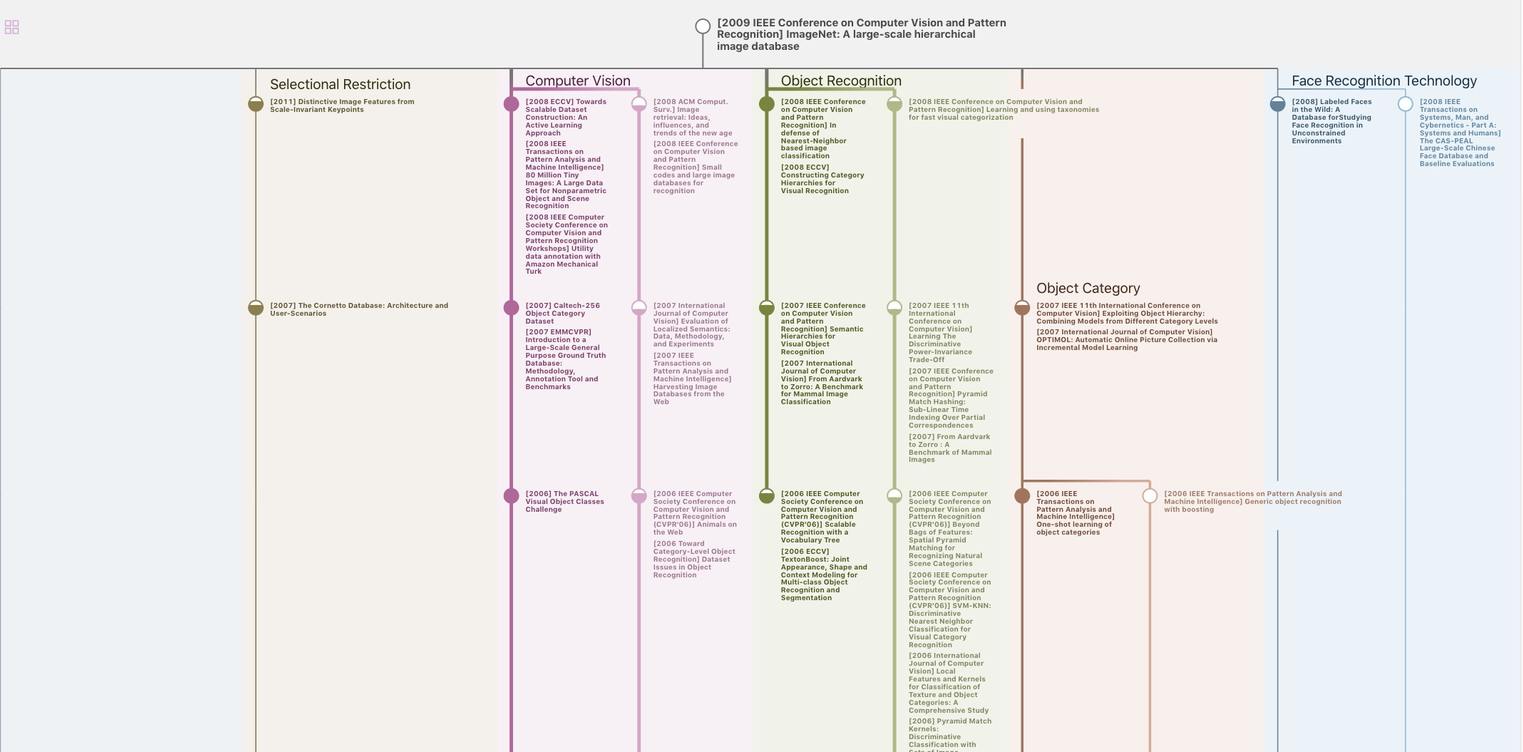
生成溯源树,研究论文发展脉络
Chat Paper
正在生成论文摘要
Open Forum Infectious Diseases(2022)