GANAD:A GAN-based Method for Network Anomaly Detection
WORLD WIDE WEB-INTERNET AND WEB INFORMATION SYSTEMS(2023)
关键词
Network anomaly detection,WGAN,Gradient penalty,Spectral normalization
AI 理解论文
溯源树
样例
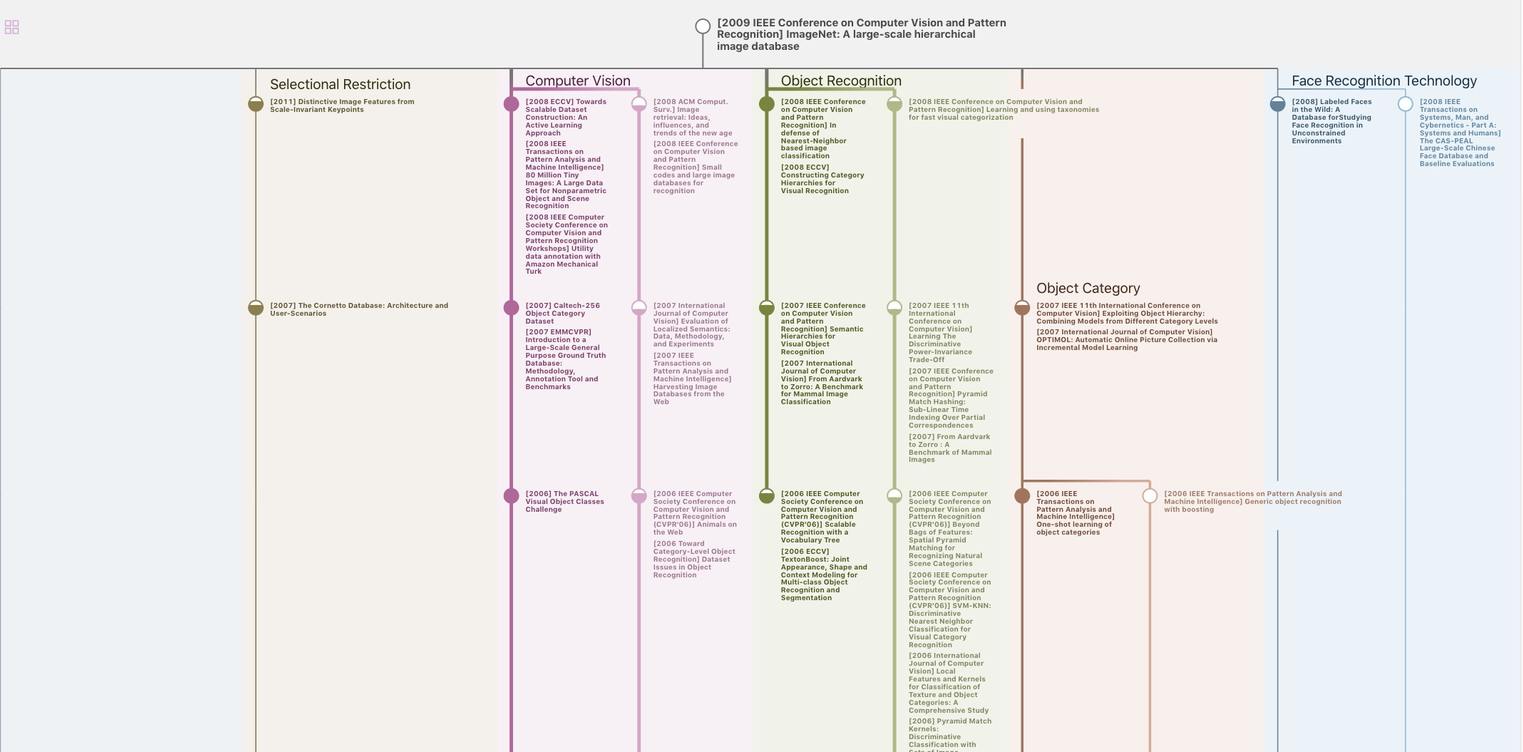
生成溯源树,研究论文发展脉络
Chat Paper
正在生成论文摘要
WORLD WIDE WEB-INTERNET AND WEB INFORMATION SYSTEMS(2023)