AfriNames: Most ASR models "butcher" African Names
CoRR(2023)
摘要
Useful conversational agents must accurately capture named entities to minimize error for downstream tasks, for example, asking a voice assistant to play a track from a certain artist, initiating navigation to a specific location, or documenting a laboratory result for a patient. However, where named entities such as ``Ukachukwu`` (Igbo), ``Lakicia`` (Swahili), or ``Ingabire`` (Rwandan) are spoken, automatic speech recognition (ASR) models' performance degrades significantly, propagating errors to downstream systems. We model this problem as a distribution shift and demonstrate that such model bias can be mitigated through multilingual pre-training, intelligent data augmentation strategies to increase the representation of African-named entities, and fine-tuning multilingual ASR models on multiple African accents. The resulting fine-tuned models show an 81.5\% relative WER improvement compared with the baseline on samples with African-named entities.
更多查看译文
关键词
butcher,most asr models,afrinames
AI 理解论文
溯源树
样例
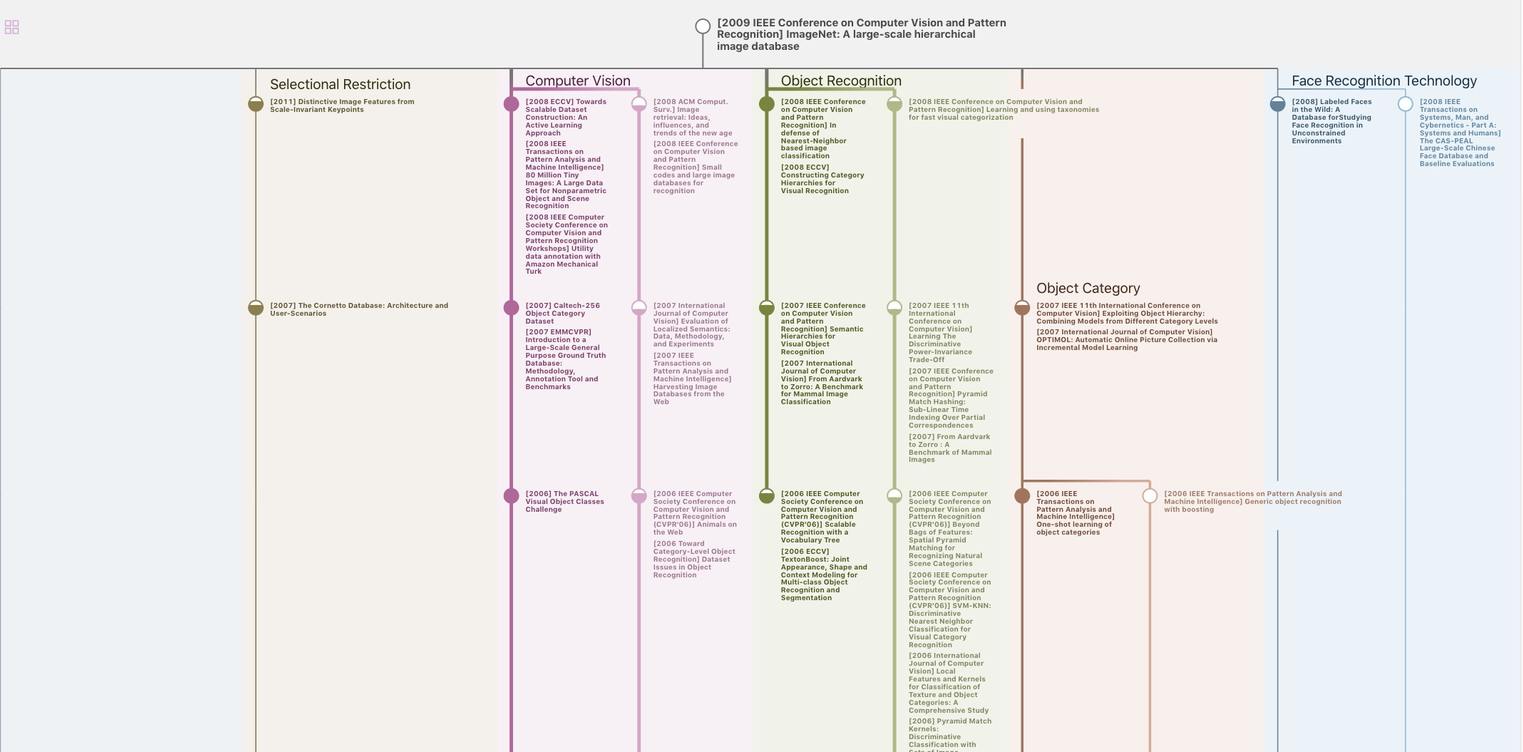
生成溯源树,研究论文发展脉络
Chat Paper
正在生成论文摘要