Vistaar: Diverse Benchmarks and Training Sets for Indian Language ASR.
INTERSPEECH 2023(2023)
Key words
Language Modeling
AI Read Science
Must-Reading Tree
Example
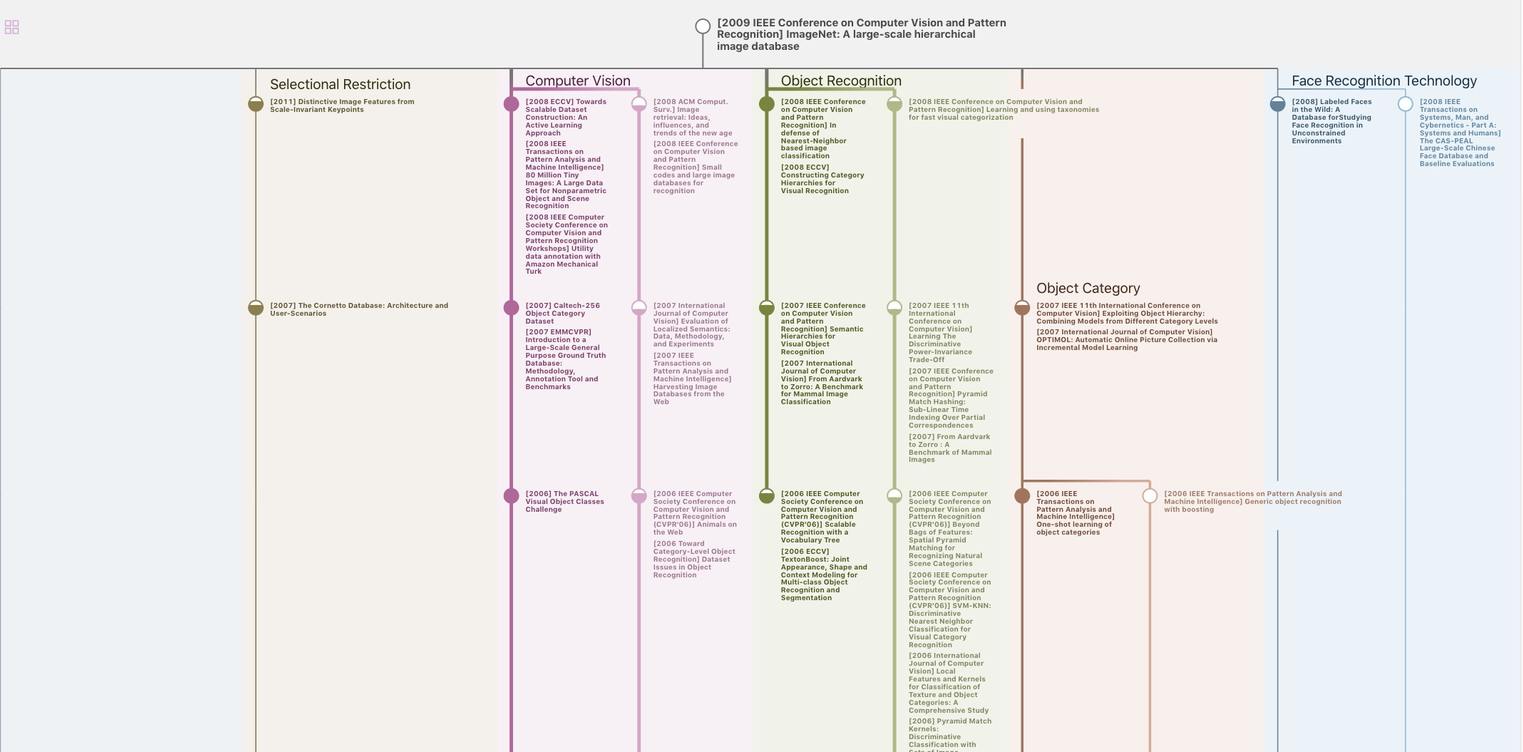
Generate MRT to find the research sequence of this paper
Chat Paper
Summary is being generated by the instructions you defined