Visually-Situated Natural Language Understanding with Contrastive Reading Model and Frozen Large Language Models.
CoRR(2023)
摘要
Advances in Large Language Models (LLMs) have inspired a surge of research exploring their expansion into the visual domain. While recent models exhibit promise in generating abstract captions for images and conducting natural conversations, their performance on text-rich images leaves room for improvement. In this paper, we propose the Contrastive Reading Model (Cream), a novel neural architecture designed to enhance the language-image understanding capability of LLMs by capturing intricate details typically overlooked by existing methods. Cream integrates vision and auxiliary encoders, complemented by a contrastive feature alignment technique, resulting in a more effective understanding of textual information within document images. Our approach, thus, seeks to bridge the gap between vision and language understanding, paving the way for more sophisticated Document Intelligence Assistants. Rigorous evaluations across diverse tasks, such as visual question answering on document images, demonstrate the efficacy of Cream as a state-of-the-art model in the field of visual document understanding. We provide our codebase and newly-generated datasets at https://github.com/naver-ai/cream
更多查看译文
关键词
reading model,natural language understanding,models,visually-situated
AI 理解论文
溯源树
样例
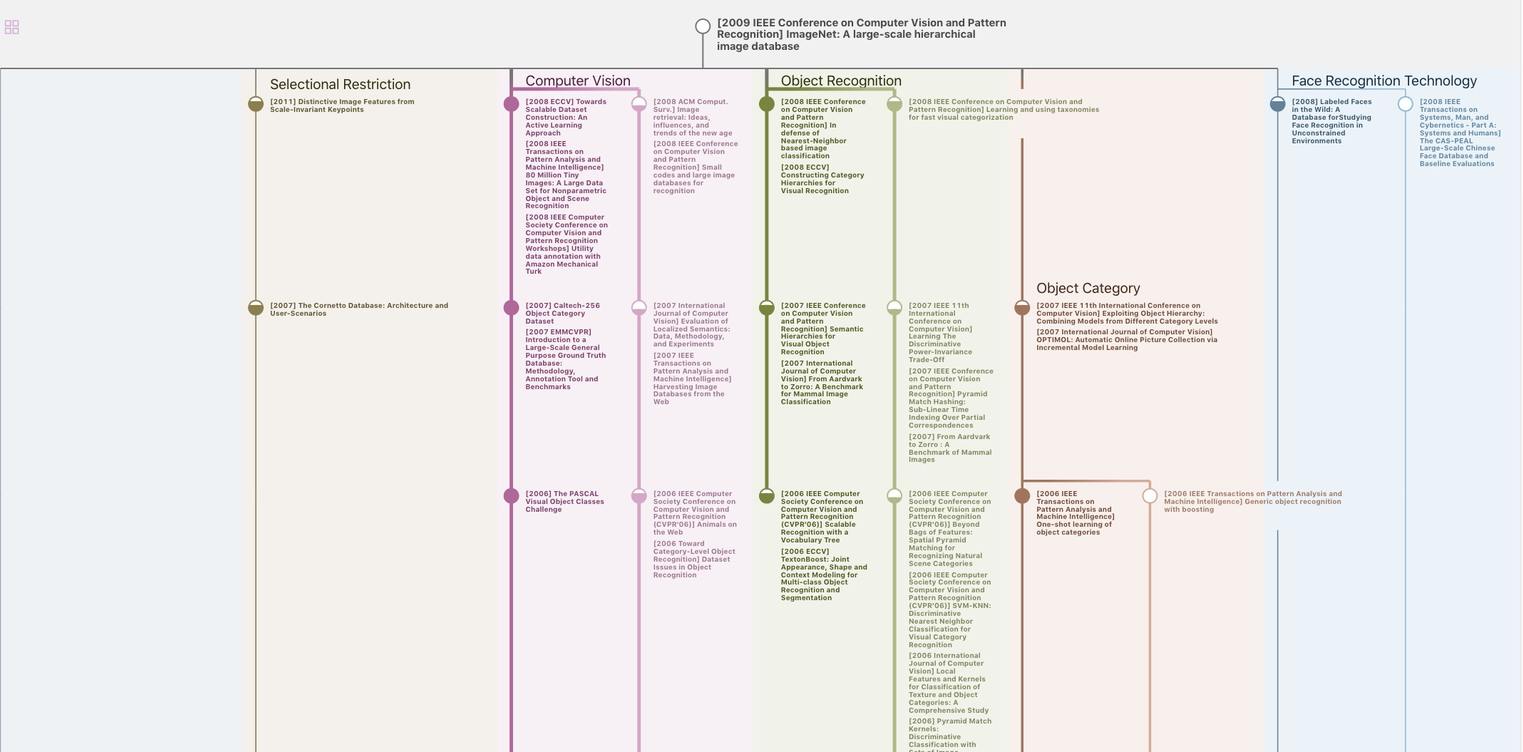
生成溯源树,研究论文发展脉络
Chat Paper
正在生成论文摘要