Federated Variational Inference: Towards Improved Personalization and Generalization
TMLR 2024(2024)
Key words
Federated Learning,Predictive Modeling,Machine Learning
AI Read Science
Must-Reading Tree
Example
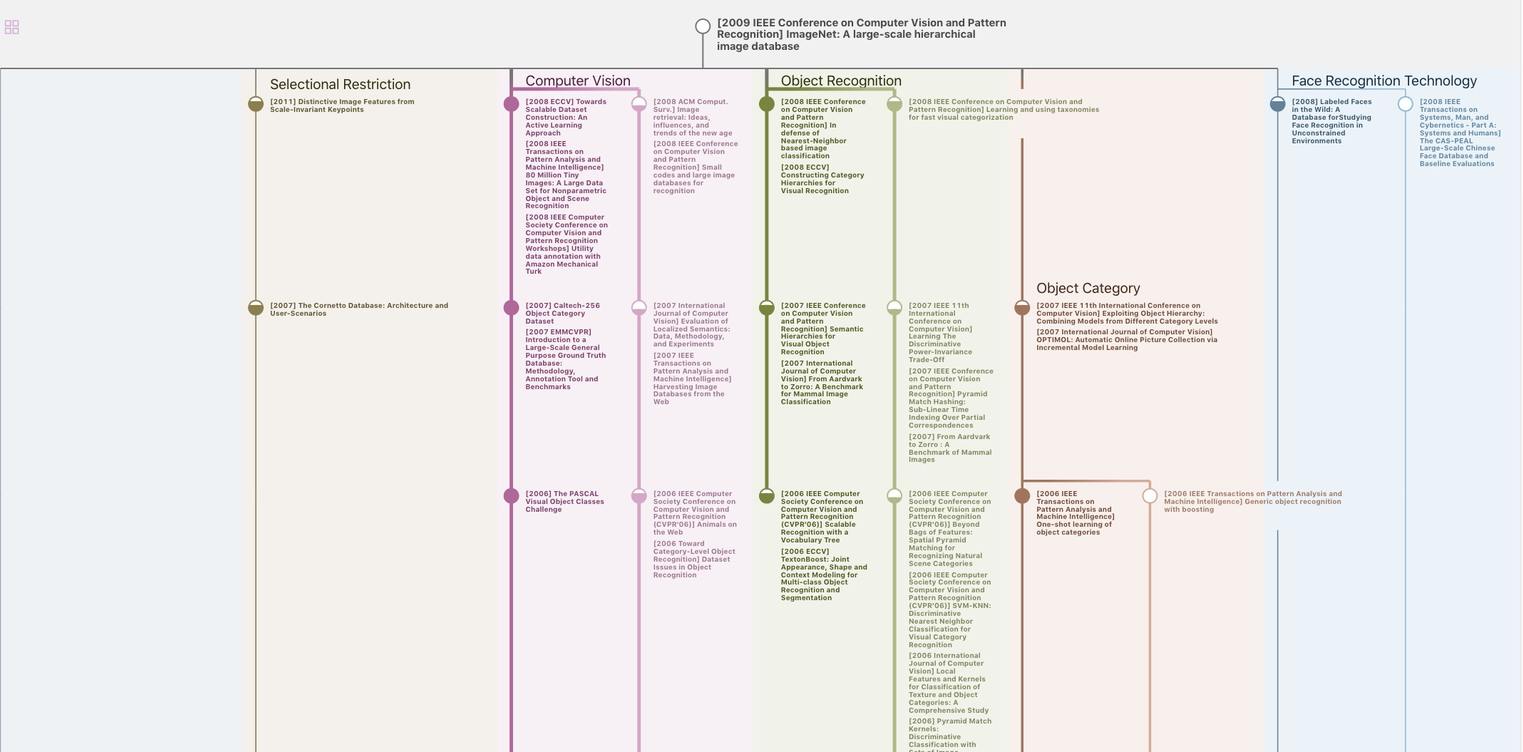
Generate MRT to find the research sequence of this paper
Chat Paper
Summary is being generated by the instructions you defined