Scalability improvement of simplified, secure distributed processing with decomposition data
Nonlinear Theory and Its Applications, IEICE(2023)
摘要
Much research on secure and safe AI analysis methods for users has been conducted. They are 1) secure multiparty computation (SMC), 2) quasi-homomorphic encryption, 3) federated learning (FL), and so on. It is known that both utility and confidentiality are essential for machine learning using confidential data. However, there is a trade-off between them. In the previous paper, we proposed a learning method for secure distributed processing using decomposition data and parameters. The characteristic feature of this method is to achieve high confidentiality through distributed processing with decomposed data and parameters. On the other hand, the realization of machine learning through the distribution and integration of decomposition data leads to an increase in computational complexity and a deterioration of computational accuracy. In particular, while increasing the number of servers improves confidentiality, this problem becomes more pronounced. In this paper, we propose scalability improvement of simplified, secure distributed processing methods of BP and NG methods to suppress the computational complexity associated with an increase in the number of servers and demonstrate its effectiveness through a numerical simulation.
更多查看译文
关键词
scalability improvement,decomposition,processing
AI 理解论文
溯源树
样例
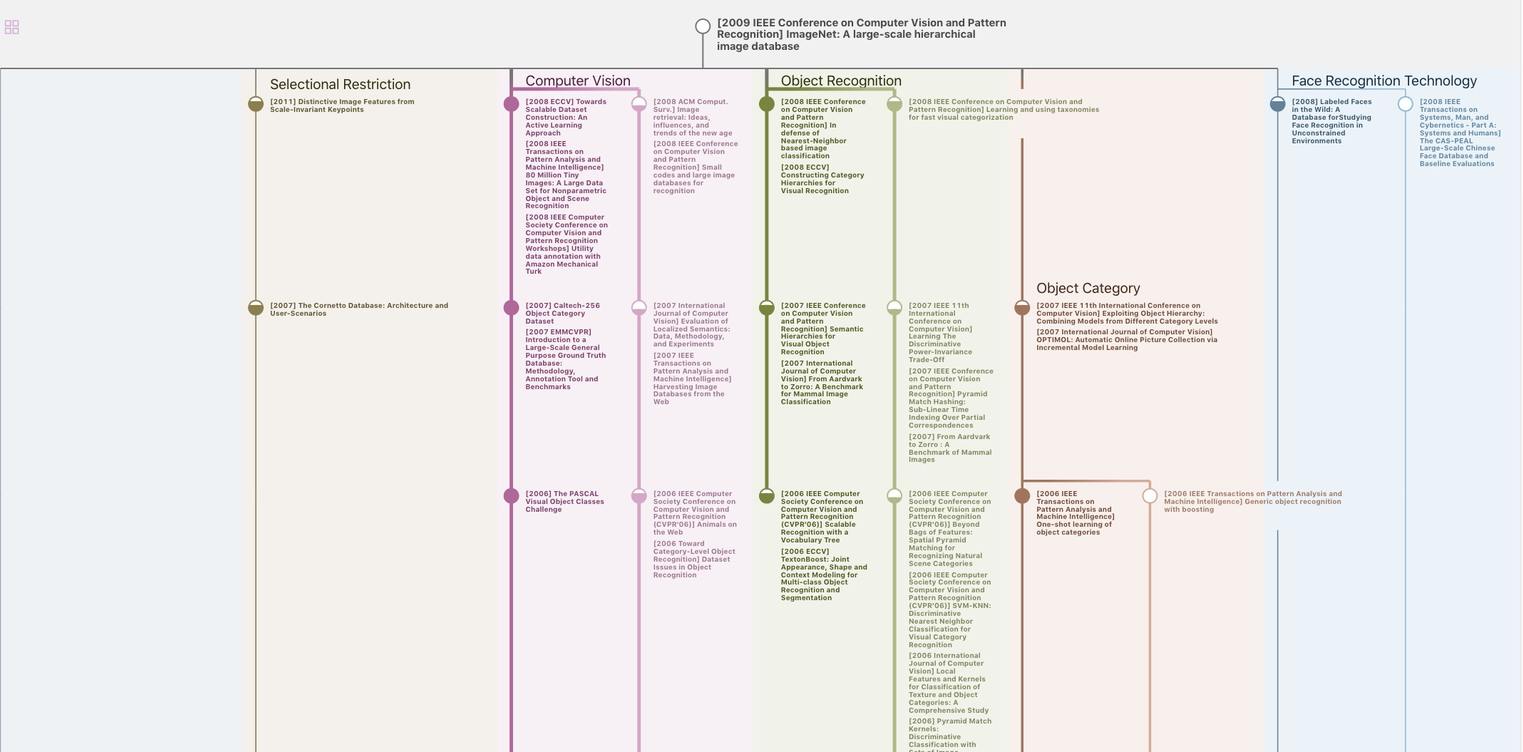
生成溯源树,研究论文发展脉络
Chat Paper
正在生成论文摘要