Actor-multi-scale context bidirectional higher order interactive relation Network for spatial-temporal action localization
IJCAI 2023(2023)
摘要
The key to video action detection lies in the understanding of interaction between persons and background objects in a video. Current methods usually employ object detectors to extract objects directly or use grid features to represent objects in the environment, which underestimate the great potential of multi-scale context information (e.g., objects and scenes of different sizes). How to exactly represent the multi-scale context and make full utilization of it still remains an unresolved challenge for spatial-temporal action localization. In this paper, we propose a novel Actor-Multi-Scale Context Bidirectional Higher Order Interactive Relation Network (AMCRNet) that extracts multi-scale context through multiple pooling layers with different sizes. Specifically, we develop an Interactive Relation Extraction Module to model the higher-order relation between the target person and the context (e.g., other persons and objects). Along this line, we further propose a History Feature Bank and Interaction Module to achieve better performance by modeling such relation across continuing video clips. Extensive experimental results on AVA2.2 and UCF101-24 demonstrate the superiority and rationality of our proposed AMCRNet.
更多查看译文
AI 理解论文
溯源树
样例
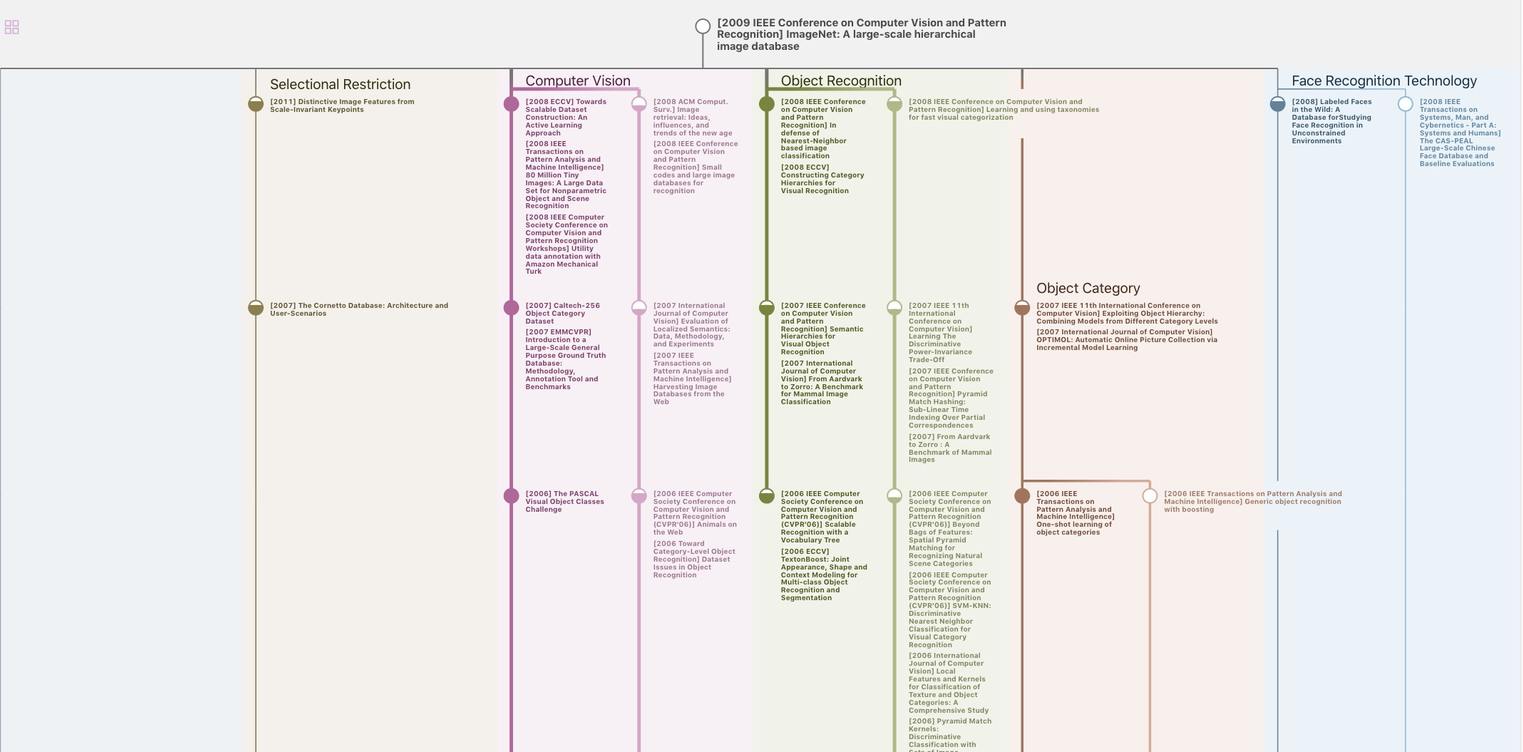
生成溯源树,研究论文发展脉络
Chat Paper
正在生成论文摘要