Preserve Your Own Correlation: A Noise Prior for Video Diffusion Models
Proceedings of the IEEE/CVF International Conference on Computer Vision (ICCV)(2023)
Key words
Image Synthesis,Texture Synthesis,Image Inpainting
AI Read Science
Must-Reading Tree
Example
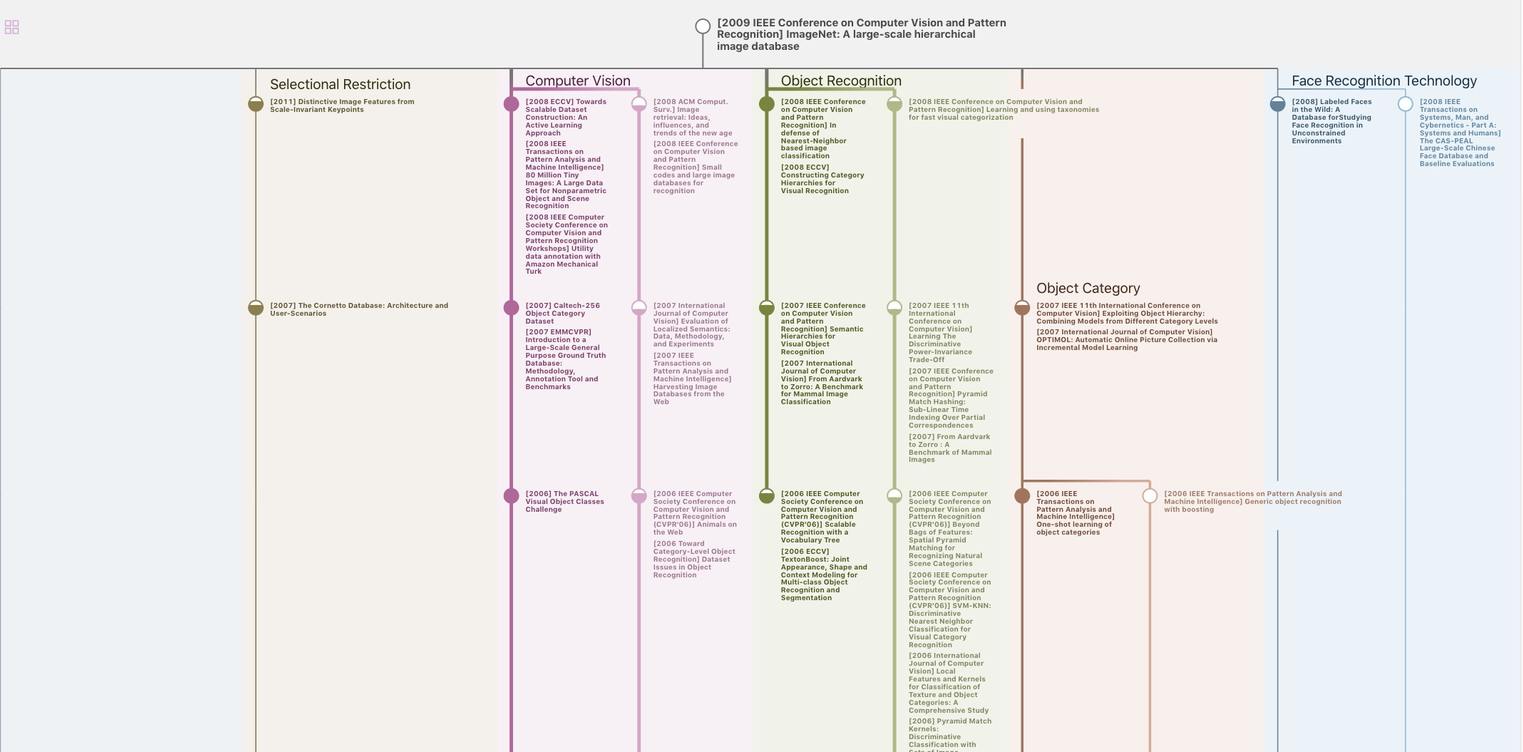
Generate MRT to find the research sequence of this paper
Chat Paper
Summary is being generated by the instructions you defined