Crop Type Mapping with Temporal Sample Migration
INTERNATIONAL JOURNAL OF REMOTE SENSING(2024)
关键词
crop mapping,historical samples,RF,time migration,Sentinel-2
AI 理解论文
溯源树
样例
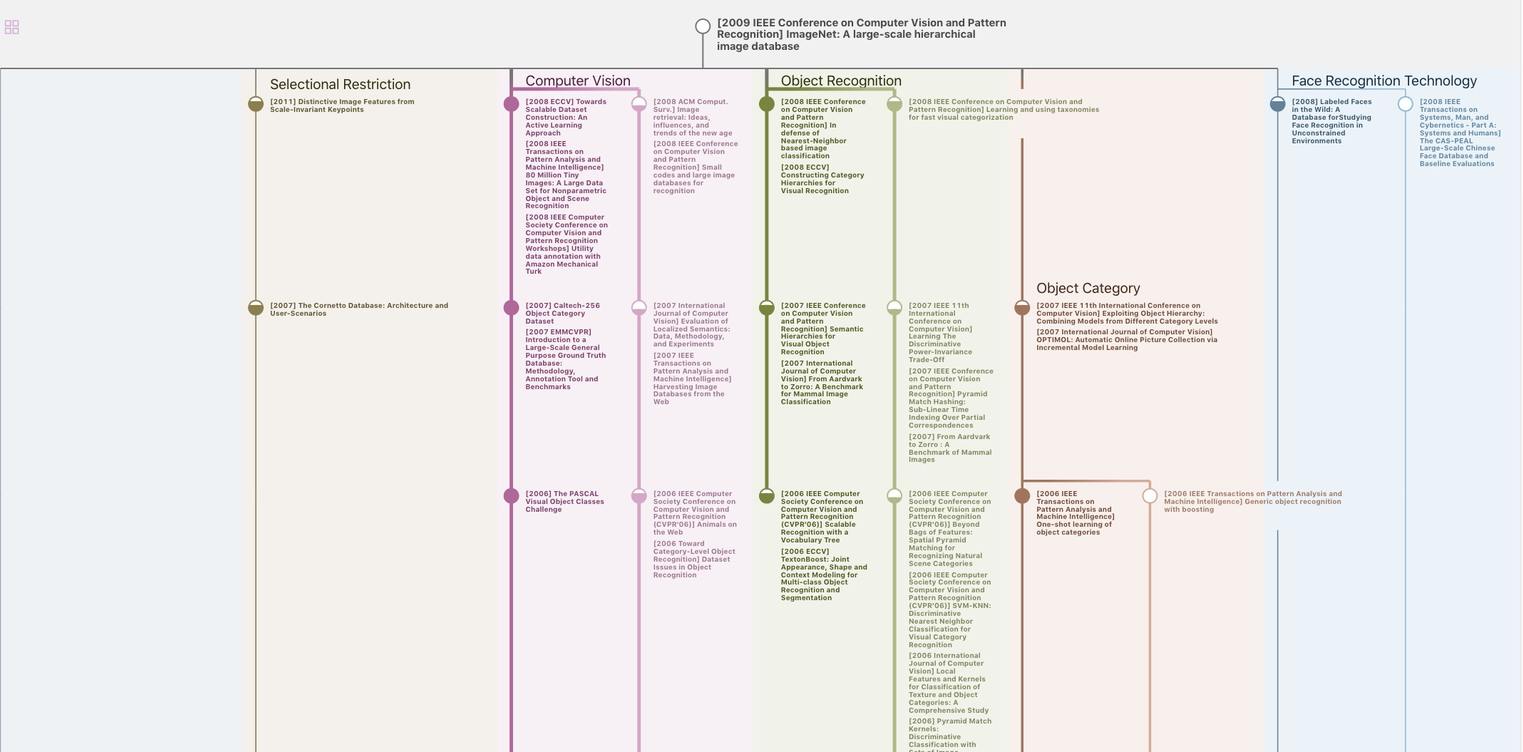
生成溯源树,研究论文发展脉络
Chat Paper
正在生成论文摘要
INTERNATIONAL JOURNAL OF REMOTE SENSING(2024)