Combining multiple spectral enhancement features for improving spectroscopic asymptomatic detection and symptomatic severity classification of southern corn leaf blight
PRECISION AGRICULTURE(2023)
摘要
Southern corn leaf blight (SCLB) seriously threatens corn production. The timely and accurate monitoring of SCLB conditions (e.g., detection during the asymptomatic stage and severity classification during the symptomatic stage) is valuable for precision agriculture, because the application of pesticides depends on disease conditions. Compared with time-consuming and laborious field surveys, spectroscopy is a promising tool for plant disease monitoring. The unique advantages of combining multiple spectral enhancement features for monitoring rice and wheat diseases have been recognized. However, physiological and biochemical differences between maize leaves and rice and wheat leaves, along with the specific spectral response of SCLB, are likely to affect the performance of combining multiple spectral enhancement features. In addition, similar previous studies have not combined spectral slope features, i.e., first-order spectral derivatives (FSDs), with spectral bands (SBs) and spectral indices (SIs) and wavelet features (WFs) to improve plant disease detection. Thus, the performance of a method that combines FSDs, WFs, SBs, and SIs for SCLB asymptomatic detection, symptomatic detection, and symptomatic severity classification should be evaluated further. Here, the utility of combining SBs, SIs, WFs, and FSDs was quantified and evaluated in the asymptomatic detection, symptomatic detection, and symptomatic severity classification of SCLB. Various forms of spectral enhancement features that were sensitive to SCLB infection from the asymptomatic stage to the severe stage were first identified and combined using the RELIEF-F and sequential floating forward selection algorithms on the basis of two independent inoculation experiments. Finally, SCLB asymptomatic detection, symptomatic detection, and symptomatic severity classification models were developed and evaluated using the support vector machine algorithm. Results showed that combining FSDs with SBs, SIs, and WFs achieved the best performance in SCLB spectroscopic monitoring. (1) SCLB asymptomatic detection and symptomatic detection were moderately improved, i.e., overall accuracy (OA) and macro F1 (MF1) improved by ~ 1% to 2%. The OA of SCLB asymptomatic detection was 87.1% with an MF1 of 0.87, and that of symptomatic detection was 93.1% with an MF1 of 0.93. (2) SCLB symptomatic severity classification was significantly improved, i.e., OA and MF1 improved by ~ 7%. The OA of severity classification was 81.8% with am MF1 of 0.82. This study demonstrated that the complementary relationships among SBs, SIs, WFs, and FSDs could effectively improve SCLB spectroscopic monitoring. The proposed method provides a novel tool for large-scale SCLB spectroscopic monitoring. It has broad implications for assisting management decisions (i.e., when and where to apply pesticides and how much to apply) in precision agriculture.
更多查看译文
关键词
Southern corn leaf blight,Asymptomatic detection,RELIEF-F algorithm,Continuous wavelet transform,Spectral derivatives
AI 理解论文
溯源树
样例
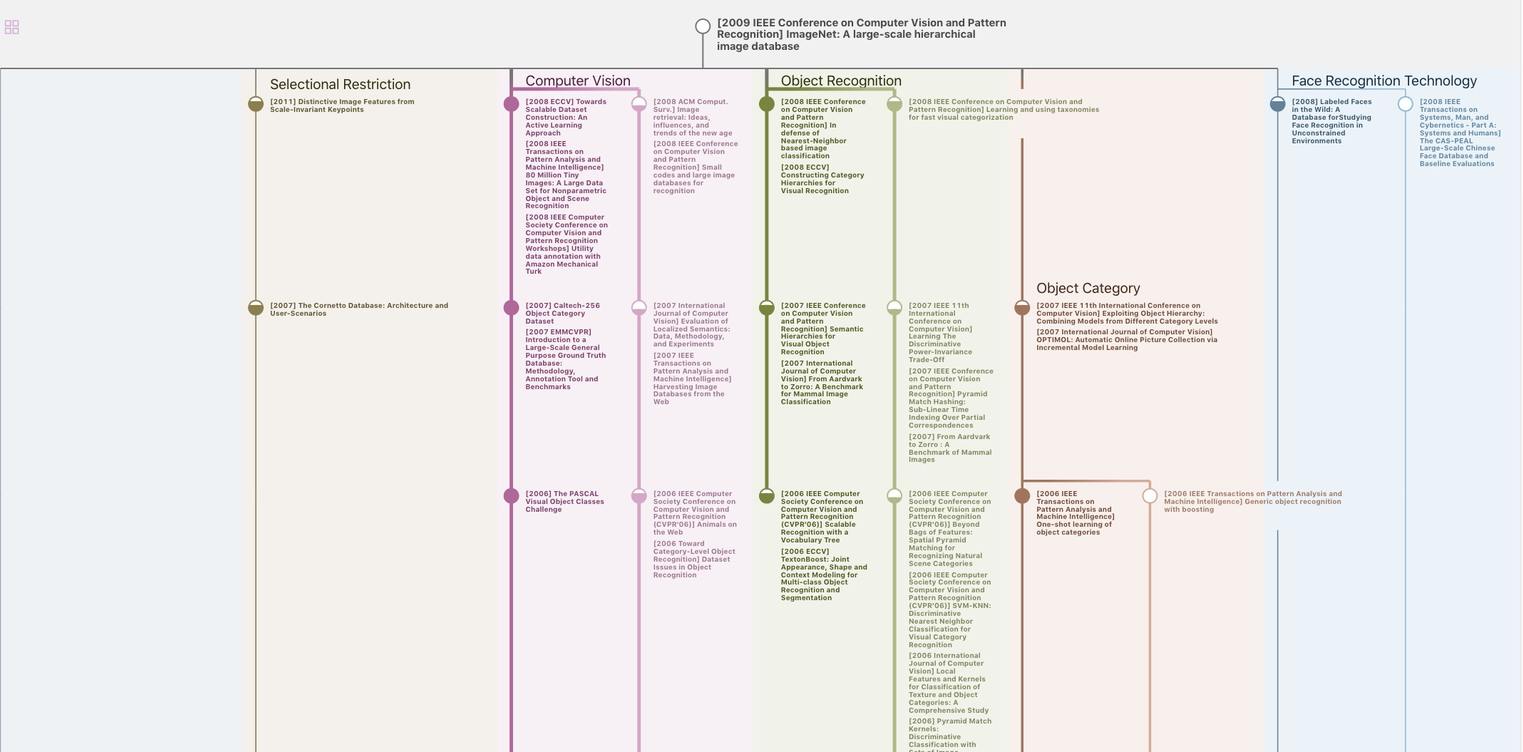
生成溯源树,研究论文发展脉络
Chat Paper
正在生成论文摘要