A Geometric Optimal Control Approach for Imitation and Generalization of Manipulation Skills
ROBOTICS AND AUTONOMOUS SYSTEMS(2023)
Key words
Learning from demonstration,Riemannian geometry,Model-based optimization,Optimal control
AI Read Science
Must-Reading Tree
Example
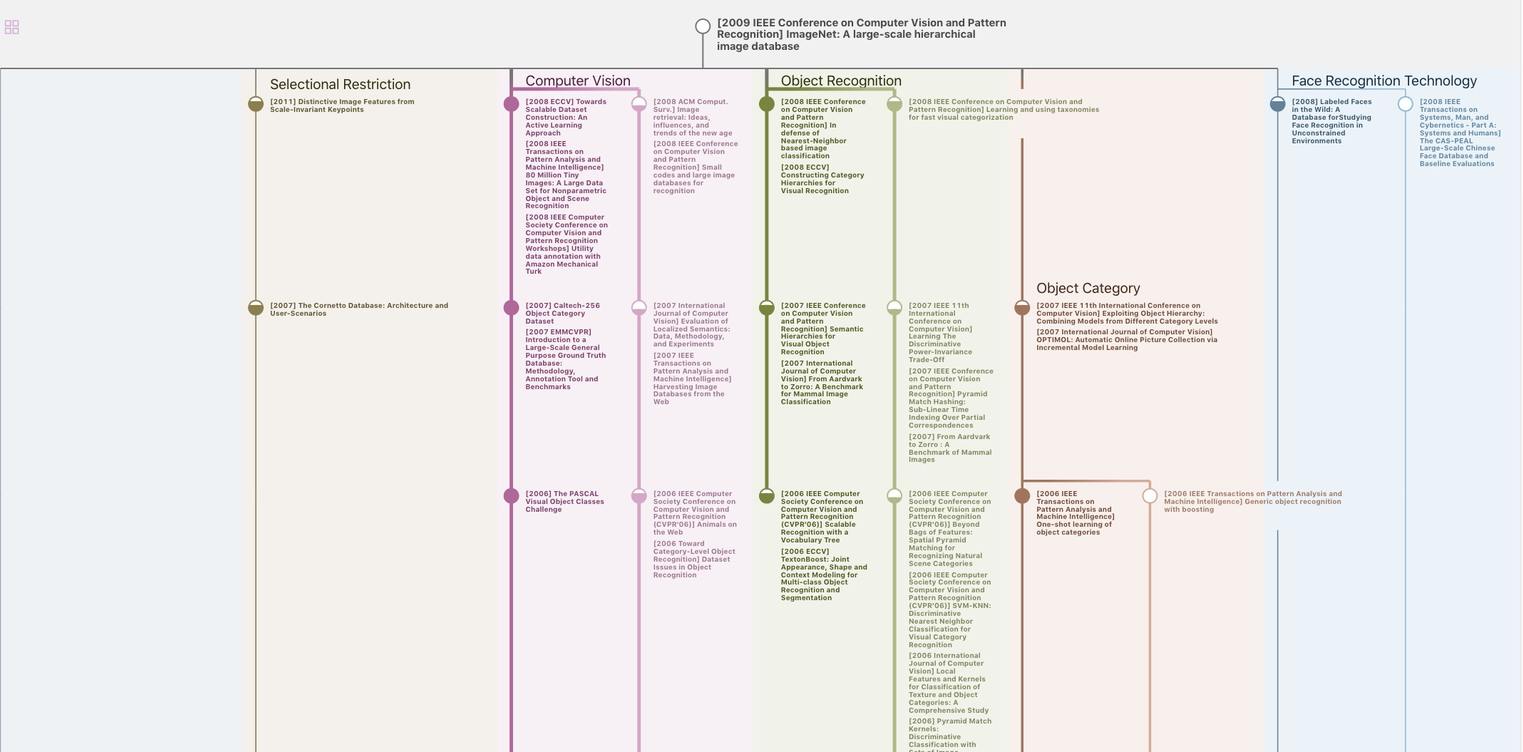
Generate MRT to find the research sequence of this paper
Chat Paper
Summary is being generated by the instructions you defined