Modelling heterogeneity in the classification process in multi-species distribution models can improve predictive performance
ECOLOGY AND EVOLUTION(2024)
摘要
Species distribution models and maps from large-scale biodiversity data are necessary for conservation management. One current issue is that biodiversity data are prone to taxonomic misclassifications. Methods to account for these misclassifications in multi-species distribution models have assumed that the classification probabilities are constant throughout the study. In reality, classification probabilities are likely to vary with several covariates. Failure to account for such heterogeneity can lead to biased prediction of species distributions. Here, we present a general multi-species distribution model that accounts for heterogeneity in the classification process. The proposed model assumes a multinomial generalised linear model for the classification confusion matrix. We compare the performance of the heterogeneous classification model to that of the homogeneous classification model by assessing how well they estimate the parameters in the model and their predictive performance on hold-out samples. We applied the model to gull data from Norway, Denmark and Finland, obtained from the Global Biodiversity Information Facility. Our simulation study showed that accounting for heterogeneity in the classification process increased the precision of true species' identity predictions by 30% and accuracy and recall by 6%. Since all the models in this study accounted for misclassification of some sort, there was no significant effect of accounting for heterogeneity in the classification process on the inference about the ecological process. Applying the model framework to the gull dataset did not improve the predictive performance between the homogeneous and heterogeneous models (with parametric distributions) due to the smaller misclassified sample sizes. However, when machine learning predictive scores were used as weights to inform the species distribution models about the classification process, the precision increased by 70%. We recommend multiple multinomial regression to be used to model the variation in the classification process when the data contains relatively larger misclassified samples. Machine learning prediction scores should be used when the data contains relatively smaller misclassified samples. This study presents a multi-species distribution model that accounts for heterogeneity in the classification process. We found out that accounting for the heterogeneity improves the predictive performance of the species distribution models.image
更多查看译文
关键词
Bayesian models,citizen science,false positives,machine learning,misclassification,multi-species distribution models
AI 理解论文
溯源树
样例
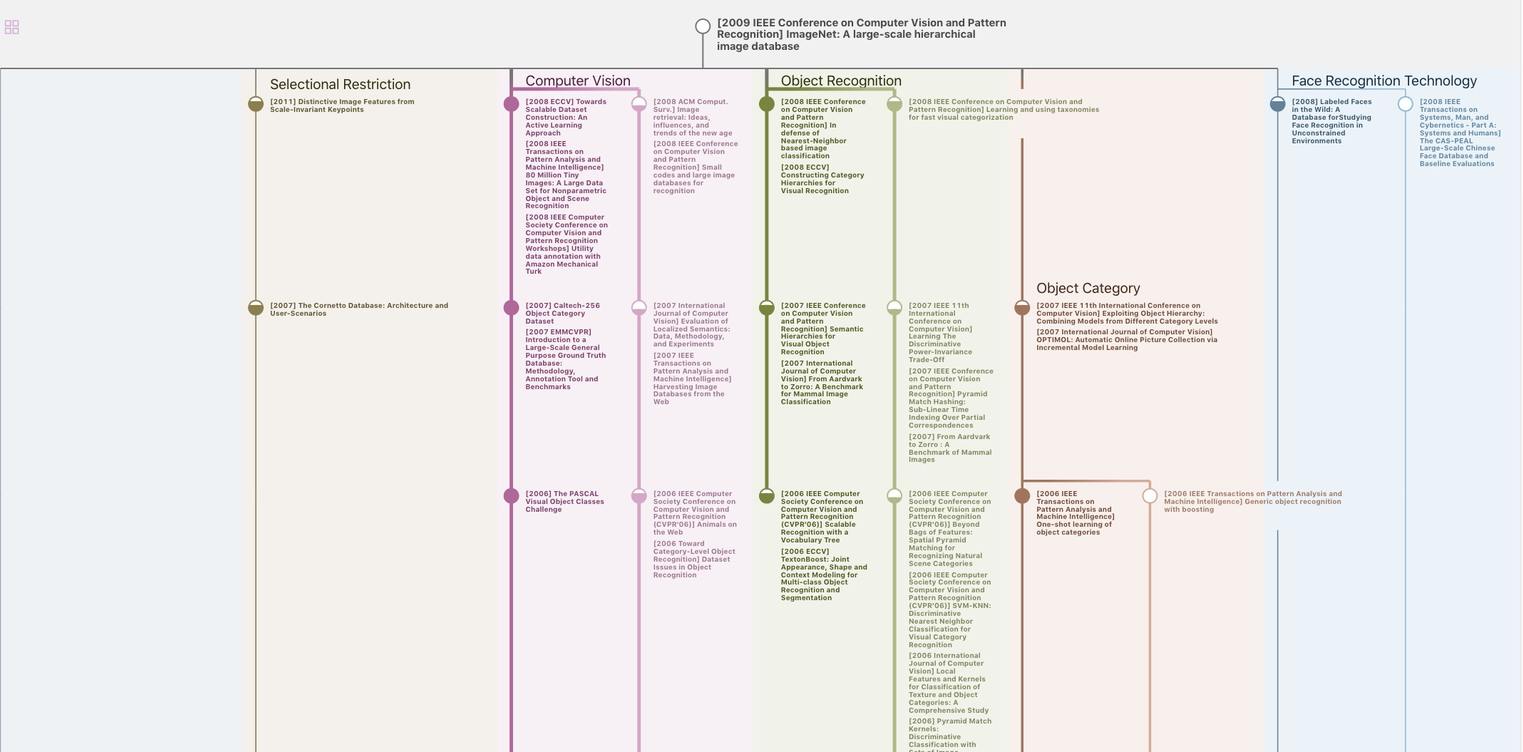
生成溯源树,研究论文发展脉络
Chat Paper
正在生成论文摘要