Proximal Curriculum for Reinforcement Learning Agents
Trans. Mach. Learn. Res.(2023)
摘要
We consider the problem of curriculum design for reinforcement learning (RL) agents in contextual multi-task settings. Existing techniques on automatic curriculum design typically require domain-specific hyperparameter tuning or have limited theoretical underpinnings. To tackle these limitations, we design our curriculum strategy, ProCuRL, inspired by the pedagogical concept of Zone of Proximal Development (ZPD). ProCuRL captures the intuition that learning progress is maximized when picking tasks that are neither too hard nor too easy for the learner. We mathematically derive ProCuRL by analyzing two simple learning settings. We also present a practical variant of ProCuRL that can be directly integrated with deep RL frameworks with minimal hyperparameter tuning. Experimental results on a variety of domains demonstrate the effectiveness of our curriculum strategy over state-of-the-art baselines in accelerating the training process of deep RL agents.
更多查看译文
关键词
reinforcement learning,agents,proximal
AI 理解论文
溯源树
样例
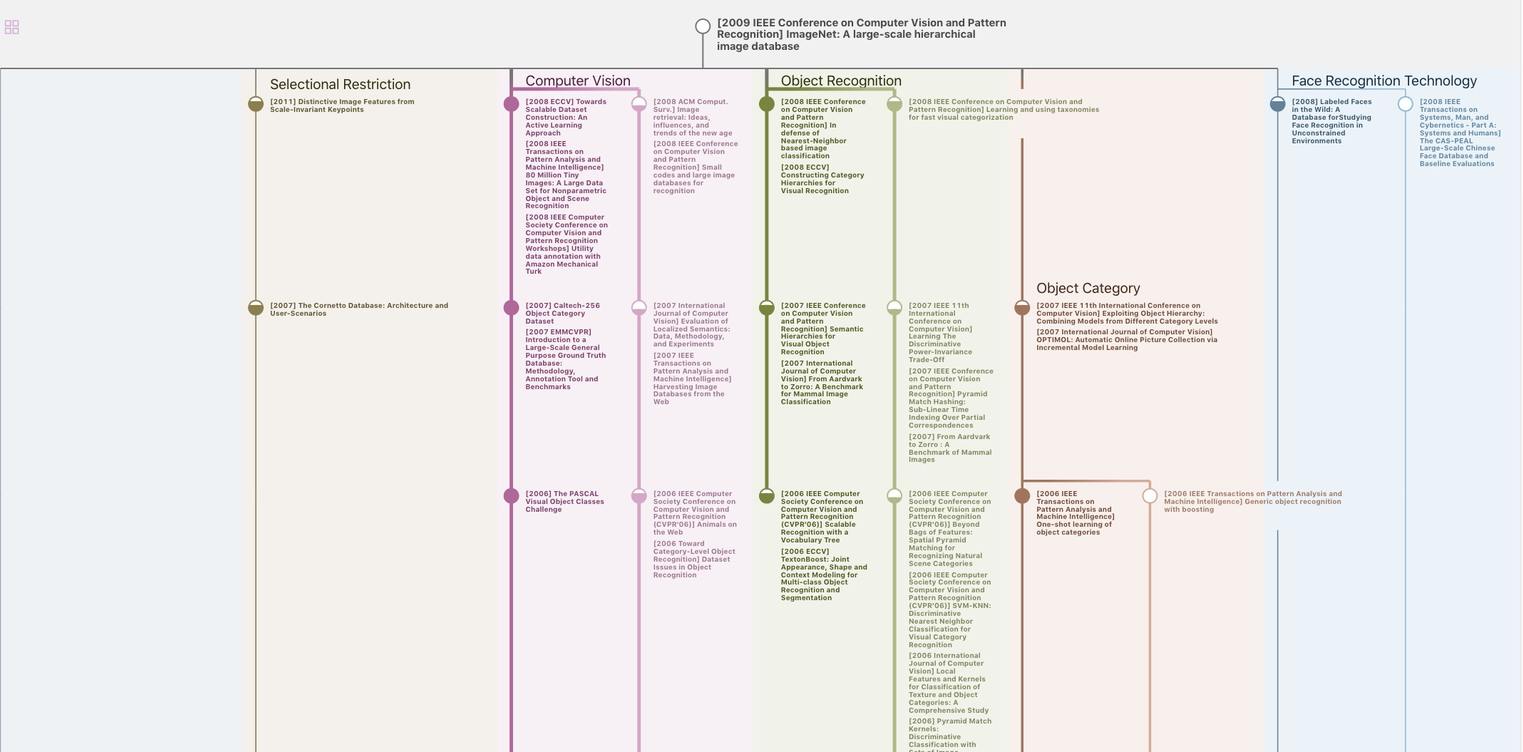
生成溯源树,研究论文发展脉络
Chat Paper
正在生成论文摘要