Discovery of Novel Multi-Functional Peptides by Using Protein Language Models and Graph-Based Deep Learning
biorxiv(2023)
Key words
Signal Peptides
AI Read Science
Must-Reading Tree
Example
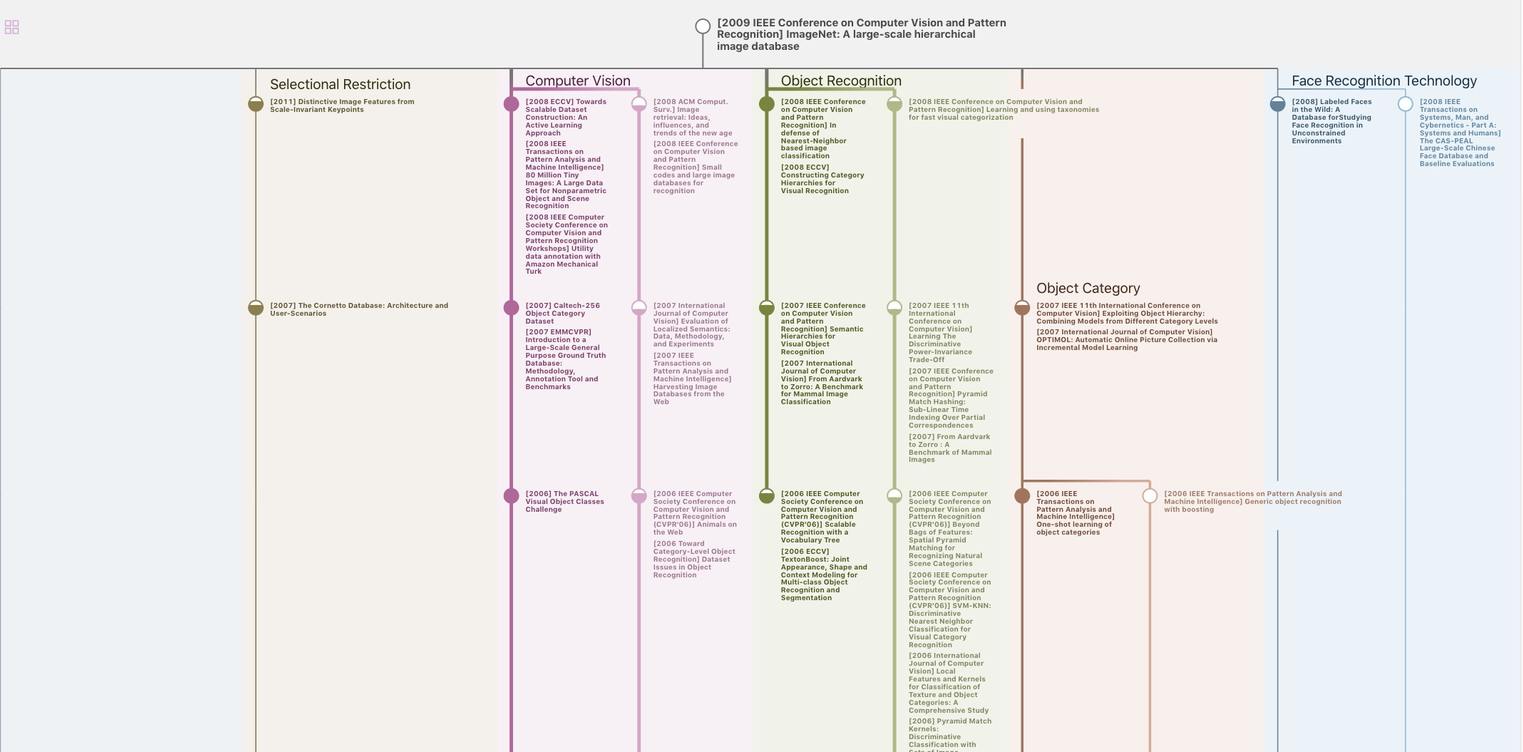
Generate MRT to find the research sequence of this paper
Chat Paper
Summary is being generated by the instructions you defined