Learned Interpolation for Better Streaming Quantile Approximation with Worst-Case Guarantees.
Society for Industrial and Applied Mathematics eBooks(2023)
Key words
Ensemble Learning,Semi-Supervised Learning
AI Read Science
Must-Reading Tree
Example
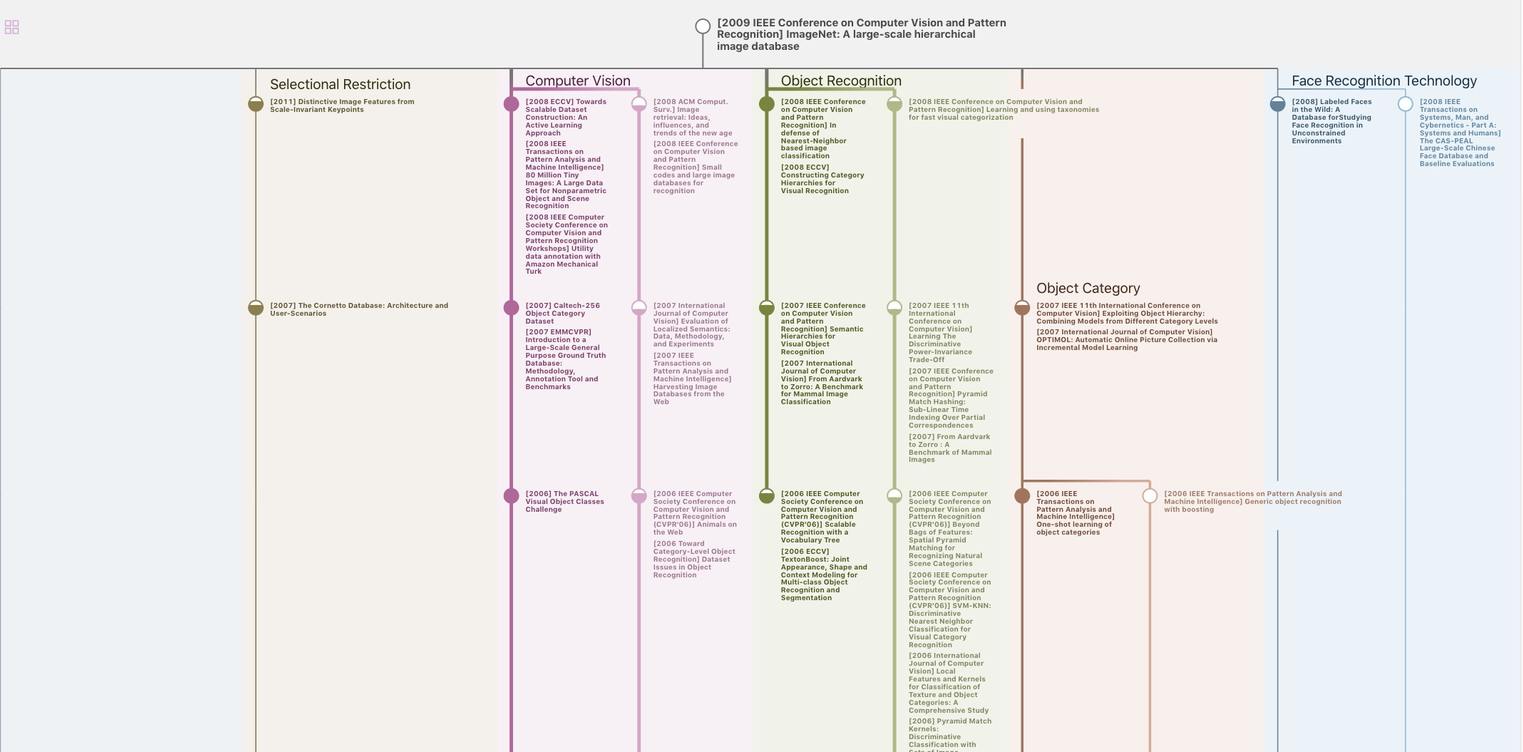
Generate MRT to find the research sequence of this paper
Chat Paper
Summary is being generated by the instructions you defined