Testing Sufficiency for Transfer Learning
arXiv (Cornell University)(2023)
摘要
Modern statistical analysis often encounters high dimensional models but with limited sample sizes. This makes the target data based statistical estimation very difficult. Then how to borrow information from another large sized source data for more accurate target model estimation becomes an interesting problem. This leads to the useful idea of transfer learning. Various estimation methods in this regard have been developed recently. In this work, we study transfer learning from a different perspective. Specifically, we consider here the problem of testing for transfer learning sufficiency. By transfer learning sufficiency (denoted as the null hypothesis), we mean that, with the help of the source data, the useful information contained in the feature vectors of the target data can be sufficiently extracted for predicting the interested target response. Therefore, the rejection of the null hypothesis implies that information useful for prediction remains in the feature vectors of the target data and thus calls for further exploration. To this end, we develop a novel testing procedure and a centralized and standardized test statistic, whose asymptotic null distribution is analytically derived. Simulation studies are presented to demonstrate the finite sample performance of the proposed method. A deep learning related real data example is presented for illustration purpose.
更多查看译文
关键词
testing sufficiency,transfer,learning
AI 理解论文
溯源树
样例
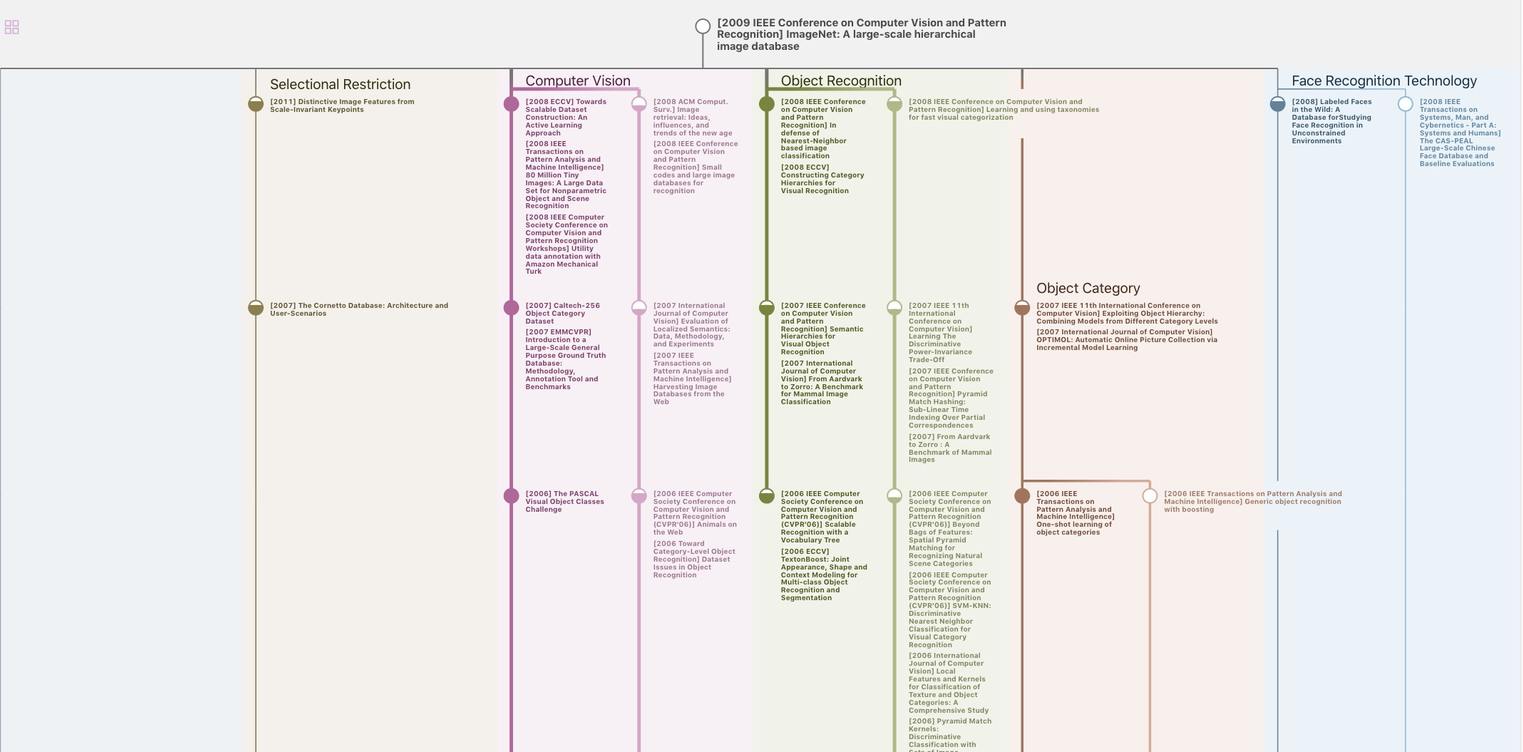
生成溯源树,研究论文发展脉络
Chat Paper
正在生成论文摘要