Data-driven Distributionally Robust Optimization over Time
INFORMS journal on optimization(2023)
摘要
Stochastic Optimization (SO) is a classical approach for optimization under uncertainty that typically requires knowledge about the probability distribution of uncertain parameters. As the latter is often unknown, Distributionally Robust Optimization (DRO) provides a strong alternative that determines the best guaranteed solution over a set of distributions (ambiguity set). In this work, we present an approach for DRO over time that uses online learning and scenario observations arriving as a data stream to learn more about the uncertainty. Our robust solutions adapt over time and reduce the cost of protection with shrinking ambiguity. For various kinds of ambiguity sets, the robust solutions converge to the SO solution. Our algorithm achieves the optimization and learning goals without solving the DRO problem exactly at any step. We also provide a regret bound for the quality of the online strategy which converges at a rate of $\mathcal{O}(\log T / \sqrt{T})$, where $T$ is the number of iterations. Furthermore, we illustrate the effectiveness of our procedure by numerical experiments on mixed-integer optimization instances from popular benchmark libraries and give practical examples stemming from telecommunications and routing. Our algorithm is able to solve the DRO over time problem significantly faster than standard reformulations.
更多查看译文
关键词
distributionally robust optimization,data-driven
AI 理解论文
溯源树
样例
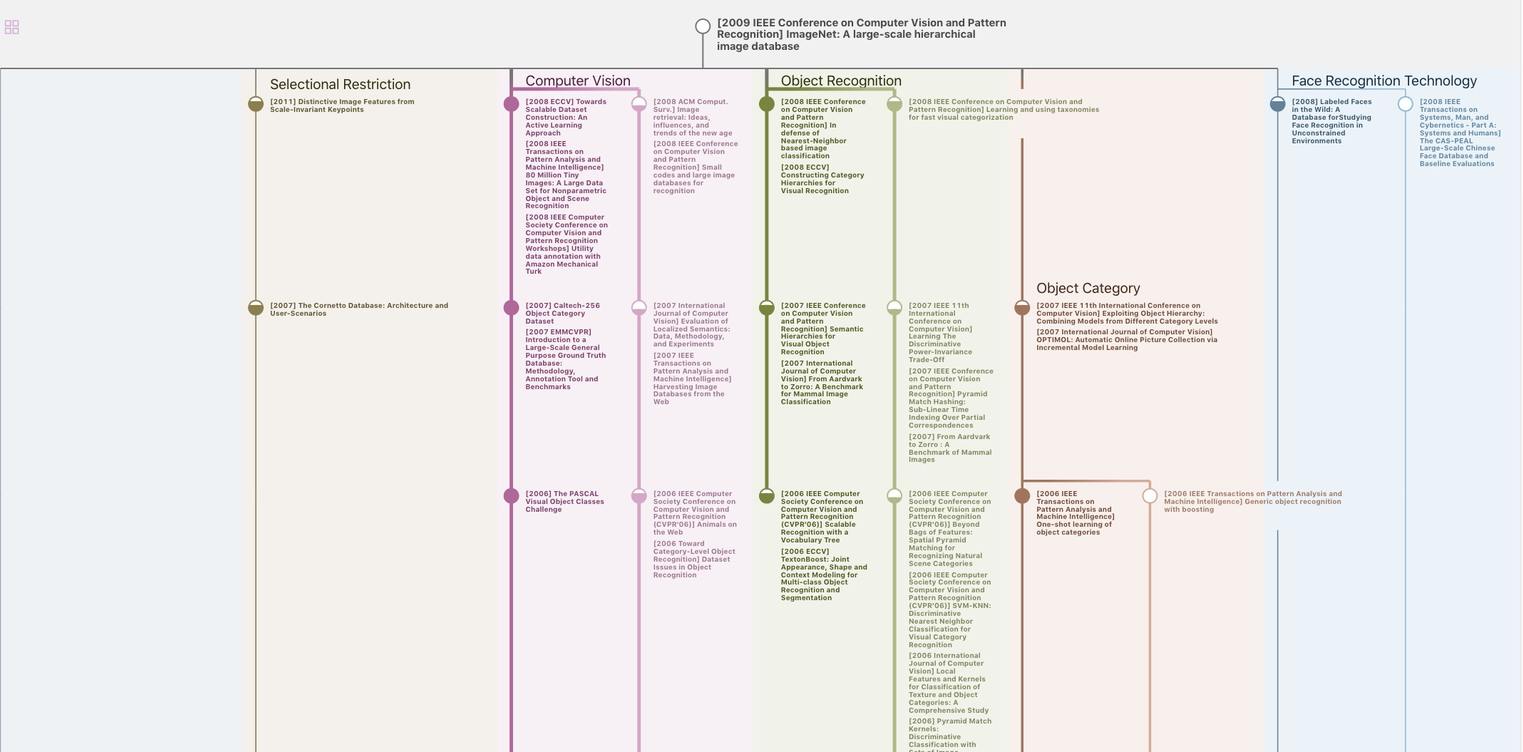
生成溯源树,研究论文发展脉络
Chat Paper
正在生成论文摘要