A surprisingly simple technique to control the pretraining bias for better transfer: Expand or Narrow your representation
CoRR(2023)
摘要
Self-Supervised Learning (SSL) models rely on a pretext task to learn representations. Because this pretext task differs from the downstream tasks used to evaluate the performance of these models, there is an inherent misalignment or pretraining bias. A commonly used trick in SSL, shown to make deep networks more robust to such bias, is the addition of a small projector (usually a 2 or 3 layer multi-layer perceptron) on top of a backbone network during training. In contrast to previous work that studied the impact of the projector architecture, we here focus on a simpler, yet overlooked lever to control the information in the backbone representation. We show that merely changing its dimensionality -- by changing only the size of the backbone's very last block -- is a remarkably effective technique to mitigate the pretraining bias. It significantly improves downstream transfer performance for both Self-Supervised and Supervised pretrained models.
更多查看译文
关键词
better transfer,bias,representation,technique
AI 理解论文
溯源树
样例
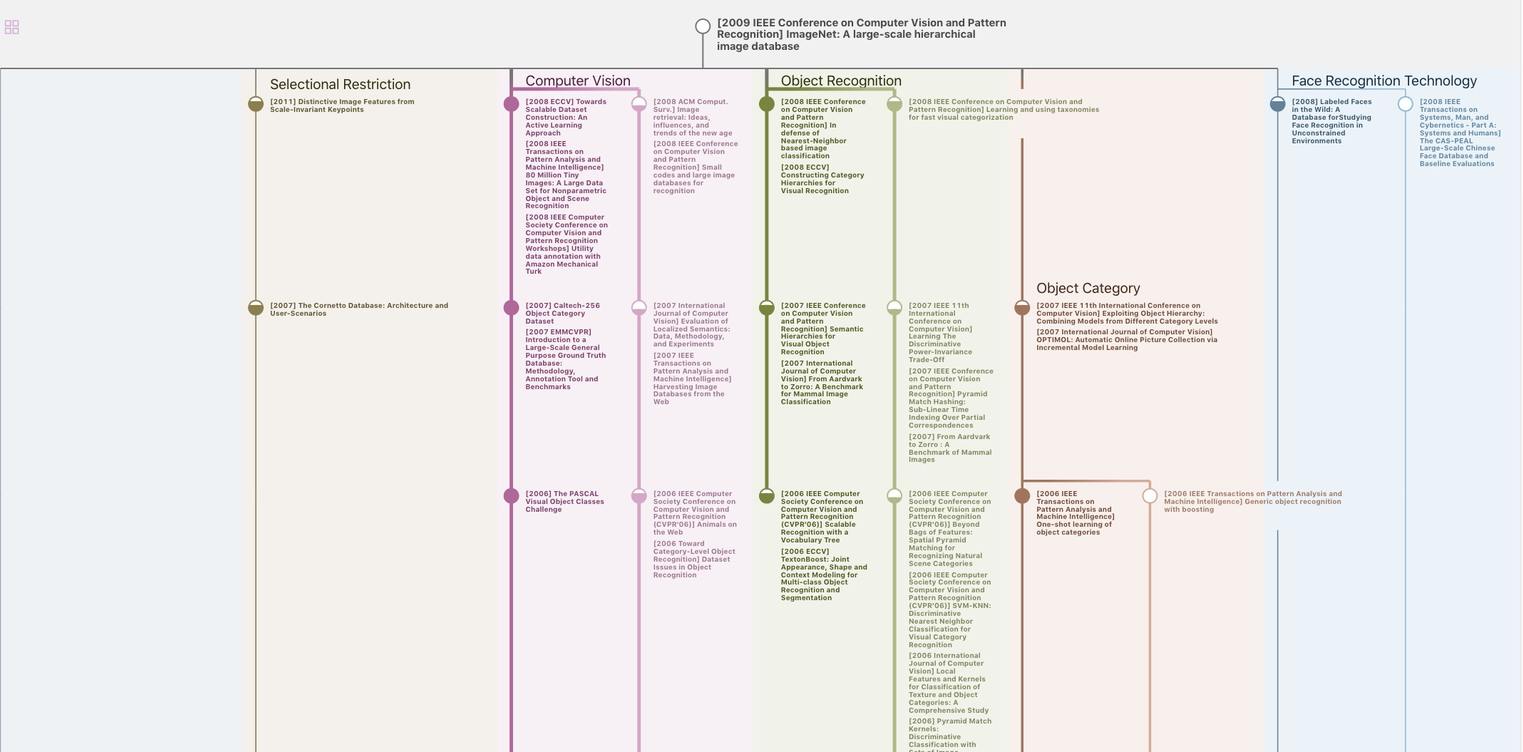
生成溯源树,研究论文发展脉络
Chat Paper
正在生成论文摘要