Physics-empowered Molecular Representation Learning
ICLR 2023(2023)
摘要
Estimating the energetic properties of molecular systems is a critical task in material design. With the trade-off between accuracy and computational cost, various methods have been used to predict the energy of materials, including recent neural-net-based models. However, most existing neural-net models are context-free (physics-ignoring) black-box models, limiting their applications to predict energy only within the distribution of the training set and thus preventing from being applied to the real practice of molecular design. Inspired by the physical mechanism of the interatomic potential, we propose a physics-driven energy prediction model using a Transformer. Our model is trained not only on the energy regression in the training set, but also with conditions inspired by physical insights and self-supervision based on Masked Atomic Modeling, making it adaptable to the optimization of molecular structure beyond the range observed during training, taking a step towards realizable molecular structure optimization.
更多查看译文
关键词
Physics,Transformer,Molecular representation learning,ML potential
AI 理解论文
溯源树
样例
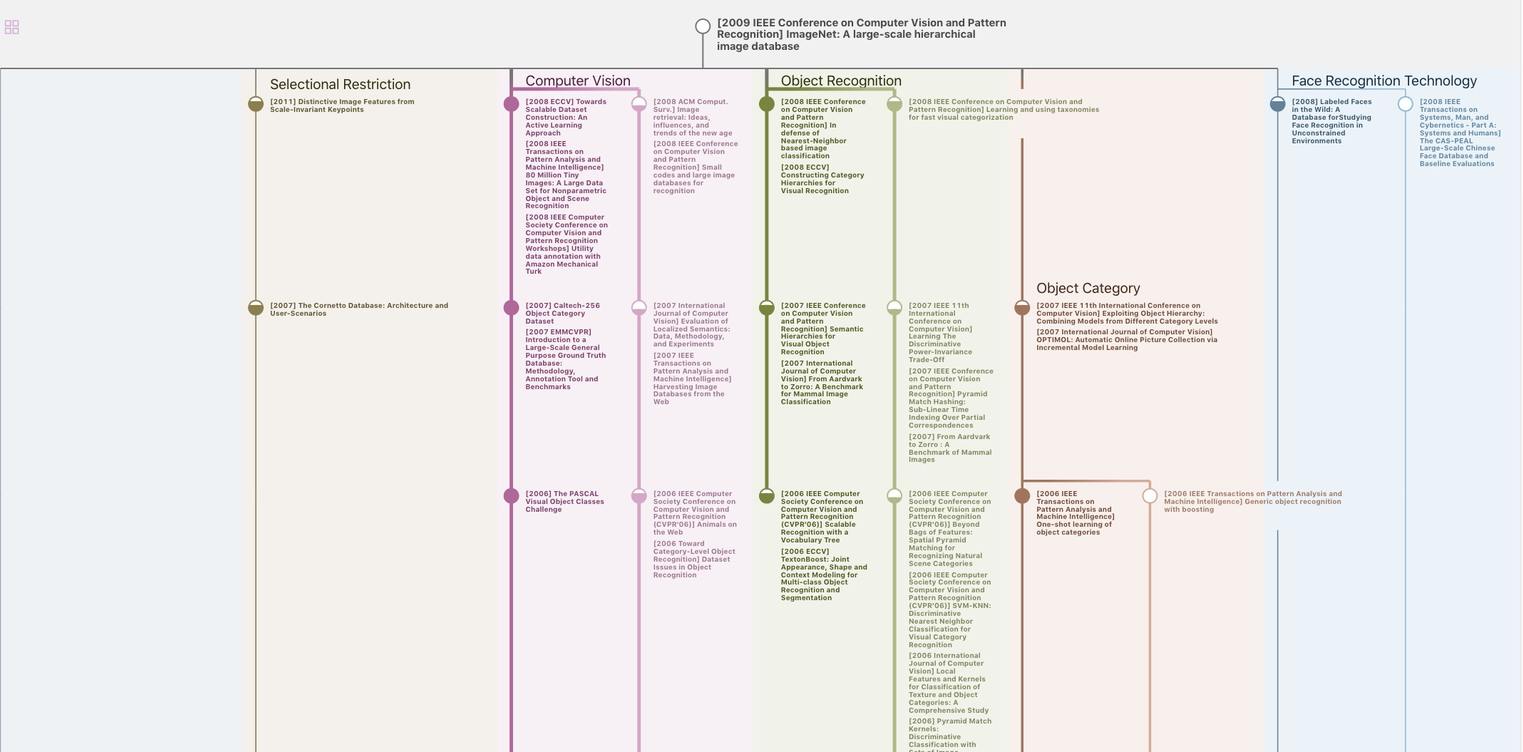
生成溯源树,研究论文发展脉络
Chat Paper
正在生成论文摘要