On the Importance of the Policy Structure in Offline Reinforcement Learning
ICLR 2023(2023)
Key words
offline reinforcement learning,discrete latent representations
AI Read Science
Must-Reading Tree
Example
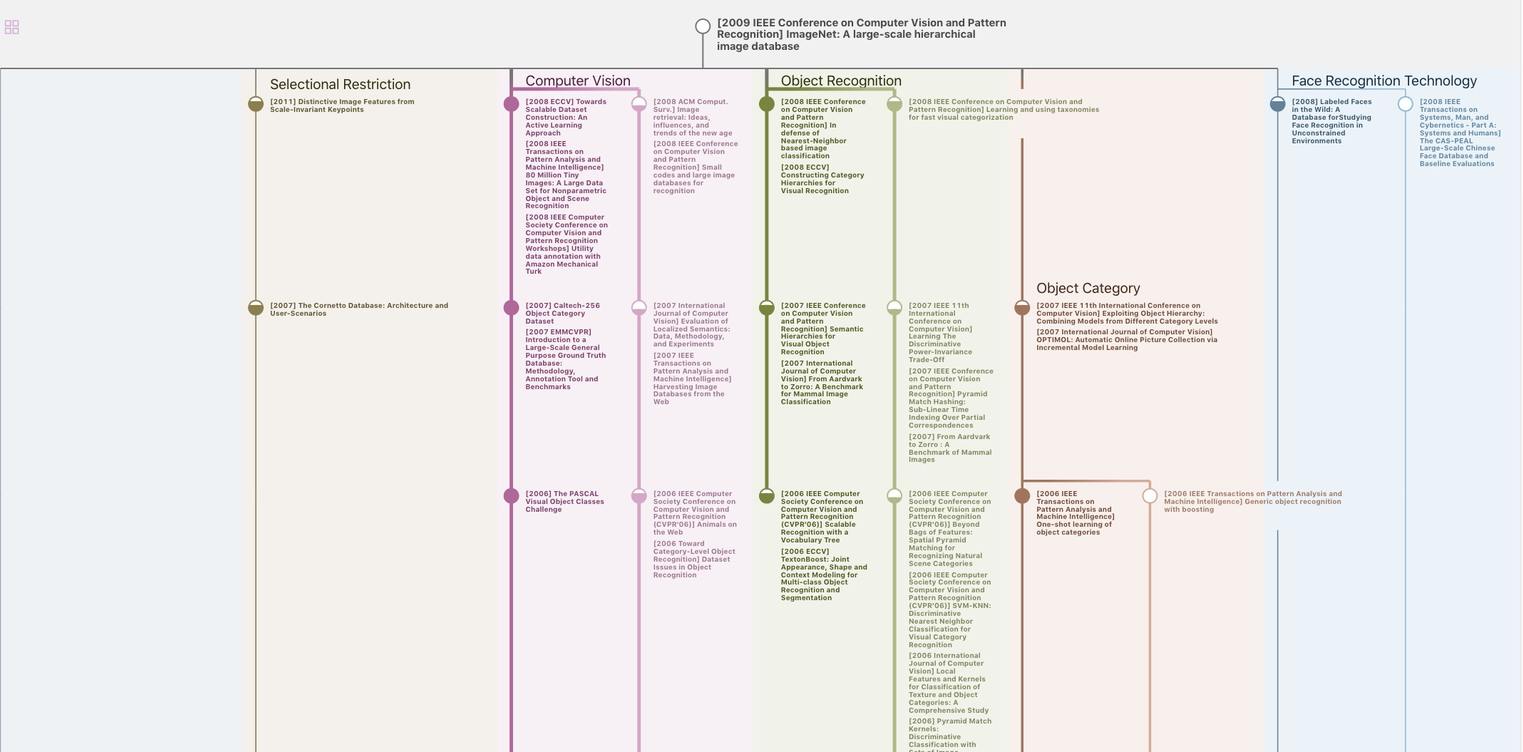
Generate MRT to find the research sequence of this paper
Chat Paper
Summary is being generated by the instructions you defined