Beyond Link Prediction: on Pre-Training Knowledge Graph Embeddings
ICLR 2023(2023)
AI Read Science
Must-Reading Tree
Example
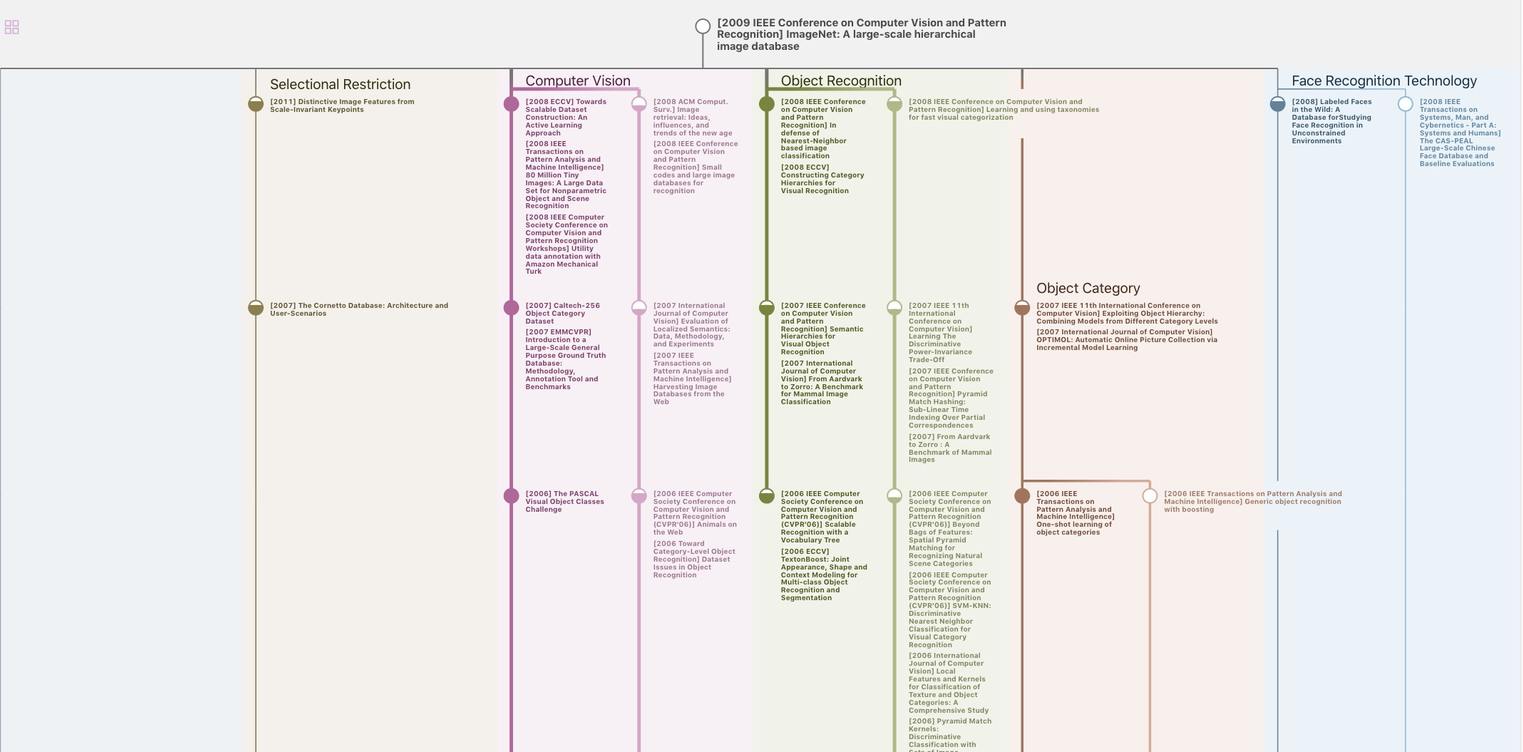
Generate MRT to find the research sequence of this paper
Chat Paper
Summary is being generated by the instructions you defined