Online black-box adaptation to label-shift in the presence of conditional-shift
ICLR 2023(2023)
摘要
We consider an out-of-distribution setting where trained predictive models are deployed online in new locations (inducing conditional-shift), such that these locations are also associated with differently skewed target distributions (label-shift). While approaches for online adaptation to label-shift have recently been discussed by Wu et al. (2021), the potential presence of concurrent conditional-shift has not been considered in the literature, although one might anticipate such distributional shifts in realistic deployments. In this paper, we empirically explore the effectiveness of online adaptation methods in such situations on three synthetic and two realistic datasets, comprising both classification and regression problems. We show that it is possible to improve performance in these settings by learning additional hyper-parameters to account for the presence of conditional-shift by using appropriate validation sets.
更多查看译文
关键词
label-shift,online,black-box,adaptation,Bayesian
AI 理解论文
溯源树
样例
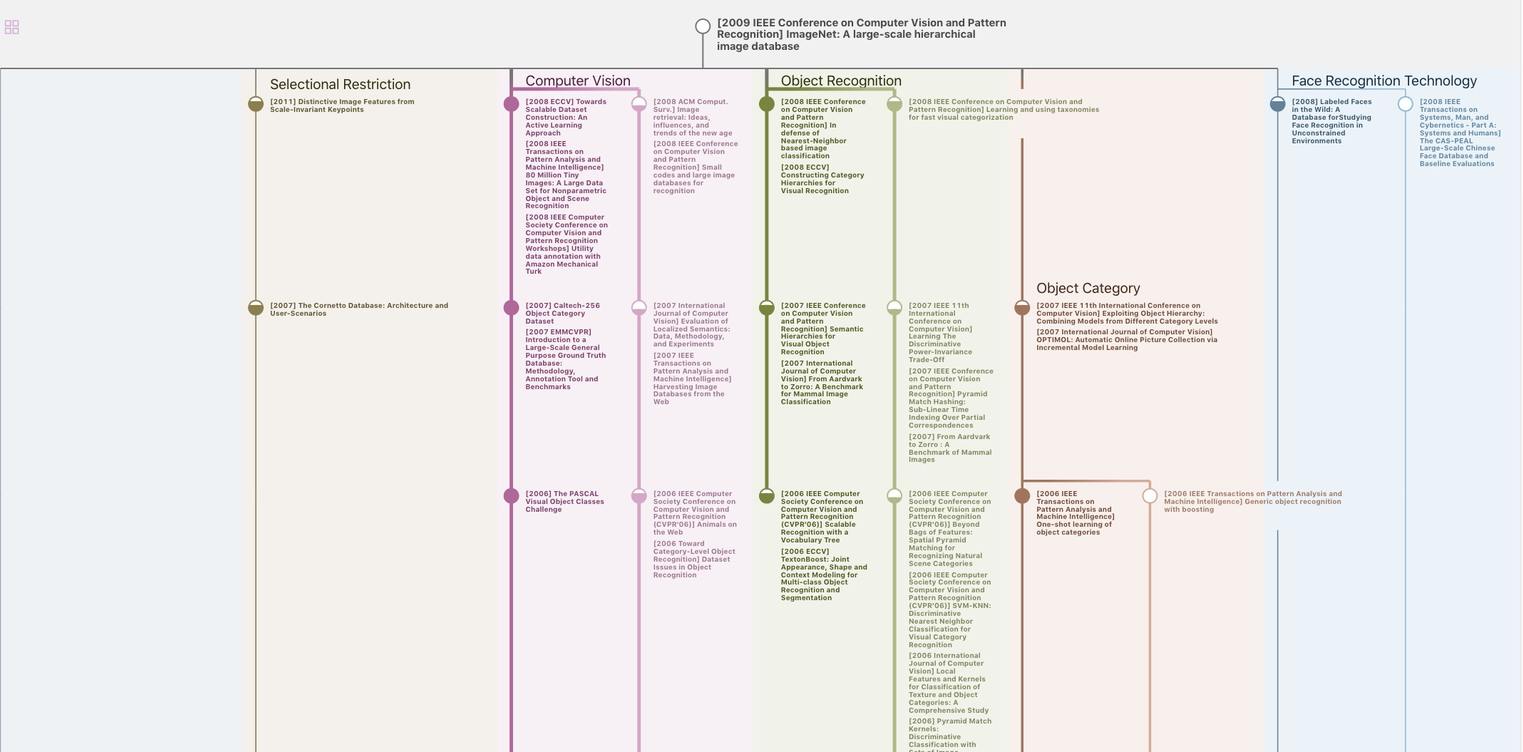
生成溯源树,研究论文发展脉络
Chat Paper
正在生成论文摘要