High probability and risk-averse guarantees for stochastic saddle point problems
arxiv(2023)
摘要
We consider strongly-convex-strongly-concave (SCSC) saddle point (SP) problems which frequently arise in many applications from distributionally robust learning to game theory and fairness in machine learning. We focus on the recently developed stochastic accelerated primal-dual algorithm (SAPD), which admits optimal complexity in several settings as an accelerated algorithm. We provide high probability guarantees for convergence to a neighborhood of the saddle point that reflects accelerated convergence behavior. We also provide an analytical formula for the limiting covariance matrix of the iterates for a class of SCSC quadratic problems where the gradient noise is additive and Gaussian. This allows us to develop lower bounds for this class of quadratic problems which show that our analysis is tight in terms of the high probability bound dependency to the parameters. We also provide a risk-averse convergence analysis characterizing "Conditional Value at Risk" and the "Entropic Value at Risk" of the distance to the saddle point, highlighting the trade-offs between the bias and the risk associated with an approximate solution.
更多查看译文
AI 理解论文
溯源树
样例
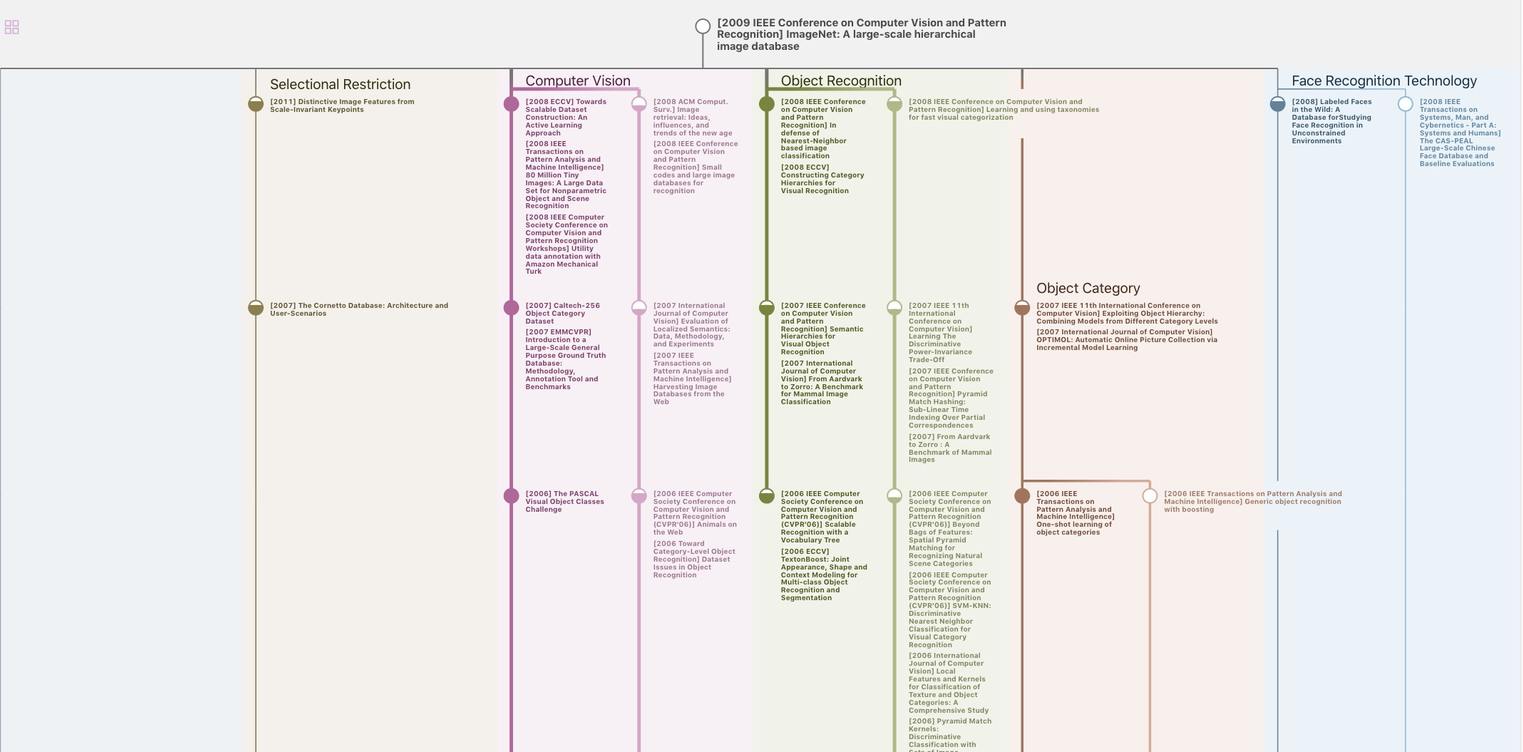
生成溯源树,研究论文发展脉络
Chat Paper
正在生成论文摘要