Attention-Based Cross-Modal CNN Using Non-Disassembled Files for Malware Classification.
IEEE Access(2023)
摘要
The role of malware classification is crucial in addressing the explosive increase in malware variants. By classifying malware instances into malware families, malware analysts can apply appropriate techniques and tools to handle malware variants in each family. Using high-level representations of malware, such as disassembled codes, yields meaningful classification performance. However, malware classification based on disassembled codes depends on the practically implausible assumption that every malware is correctly reversed by disassemblers. Unfortunately, sophisticated malware, which has anti-disassembly capabilities, seeks to confuse disassemblers, yielding incorrectly disassembled codes. In this study, we focus on malware family classification, which requires no disassembly, and propose a new CNN-based malware classification model using non-disassembled malware files (i.e., binary files). Our model associates two modalities: "malware images " and "structural entropies, " which are converted and extracted from binary files. Both modalities have different granularities of bytes and chunks that complement each other. The model adopts a cross-modal attention mechanism to combine the features of the two modalities by moderating their expressive limitations. We validate our model using three popular datasets from the Kaggle Microsoft Malware Classification, Malimg, and BODMAS datasets. The experimental results show that our model identifies malware families with a higher degree of accuracy than previous methods and does not require the burden of disassembling.
更多查看译文
关键词
Malware,Feature extraction,Entropy,Codes,Behavioral sciences,Runtime,Visualization,Malware classification,structural entropy,malware image,deep learning,convolutional neural network,attention mechanism
AI 理解论文
溯源树
样例
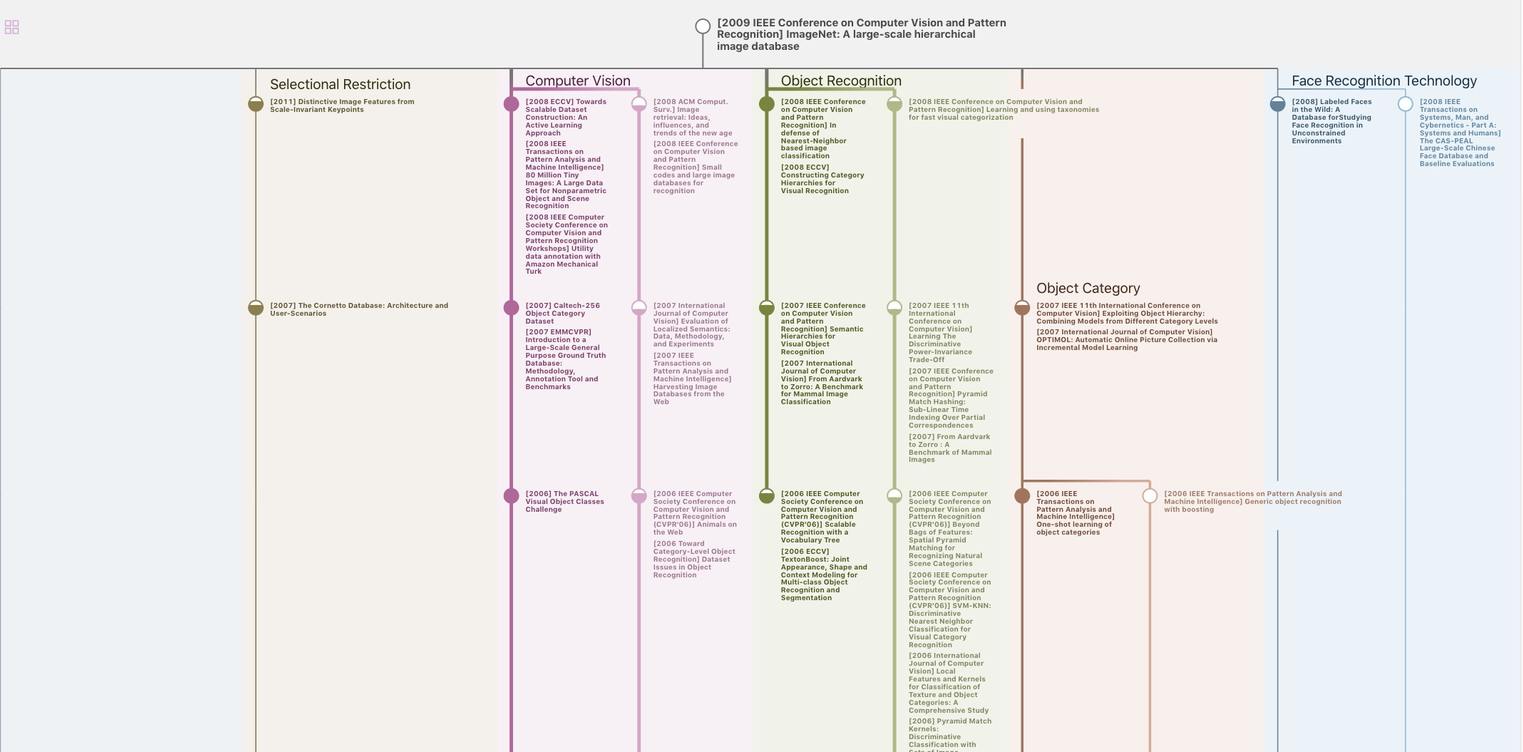
生成溯源树,研究论文发展脉络
Chat Paper
正在生成论文摘要