Image-based Features in Machine Learning to Identify Delivery Errors and Predict Error Magnitude for Patient-Specific IMRT Quality Assurance.
STRAHLENTHERAPIE UND ONKOLOGIE(2023)
关键词
Thoracic cancer,Error prediction,Dose distribuction,Artificial intelligence,Random forest
AI 理解论文
溯源树
样例
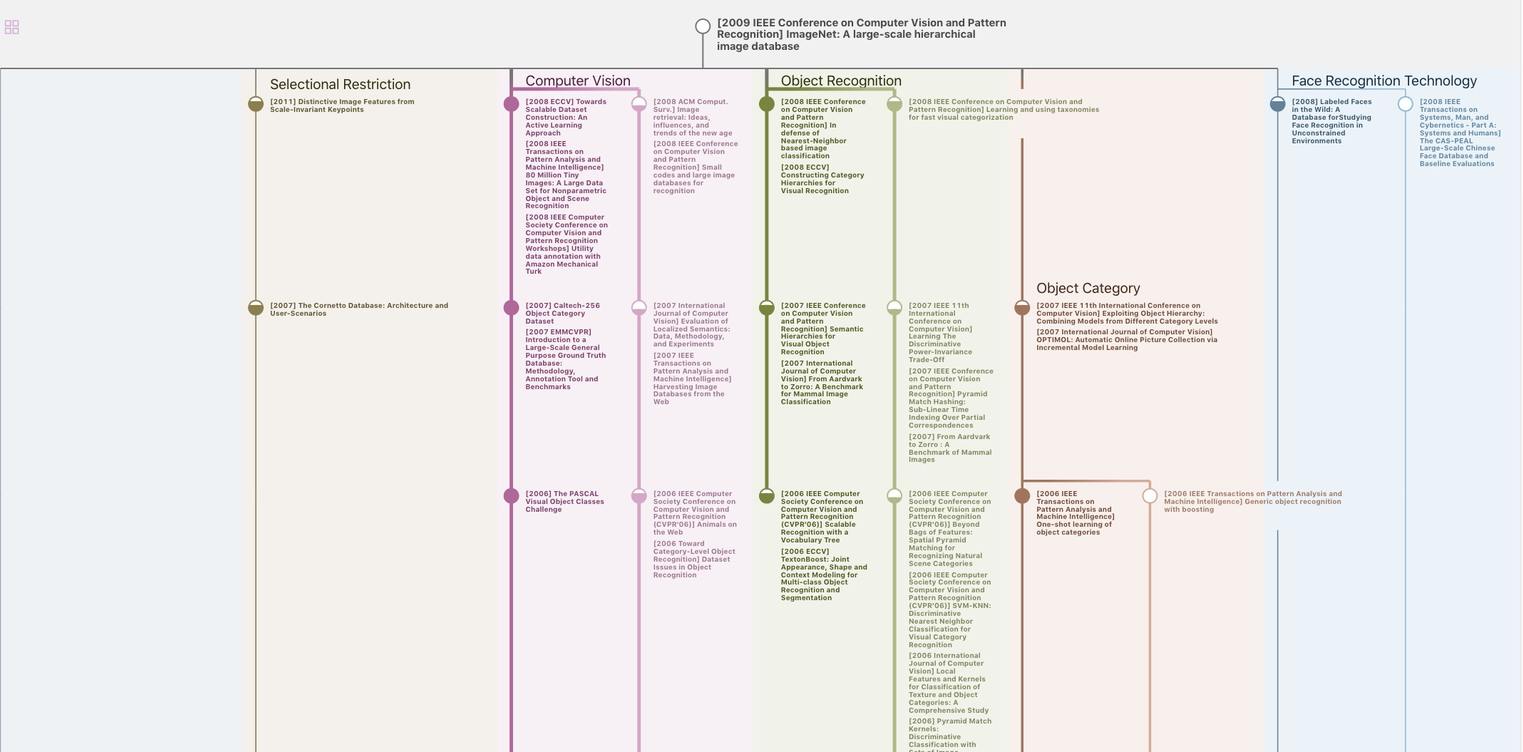
生成溯源树,研究论文发展脉络
Chat Paper
正在生成论文摘要