Short-term probabilistic prediction of significant wave height using bayesian model averaging: Case study of chabahar port, Iran
Ocean Engineering(2023)
摘要
Accurate predictions of significant wave heights are important for a number of maritime applications, such as design of coastal and offshore structures. In the present study, an ensemble approach of Bayesian model averaging (BMA) is used for the prediction of significant wave heights. The BMA is used in conjunction with three machine learning methods: Multivariate Adaptive Regression Spline (MARS), Random Forest (RF) and Gradient Boosted Regression Trees (GBRT). Daily data from three wave monitoring stations located near the Chabahar Bay in the Gulf of Oman are used to evaluate performance of the methods in predicting the significant wave height for the lead times of one, two, three and six days. The machine learning methods are also compared with auto-regressive method. The obtained results indicate that BMA model performs slightly better than the GBRT, MARS, RF and AR methods in some cases especially for the six-day ahead time horizon and all the methods are generally competitive in predicting significant wave heights.
更多查看译文
关键词
Significant wave height,Multivariate adaptive regression spline (MARS),Random forest (RF),Gradient boosted regression trees (GBRT),Bayesian model averaging (BMA)
AI 理解论文
溯源树
样例
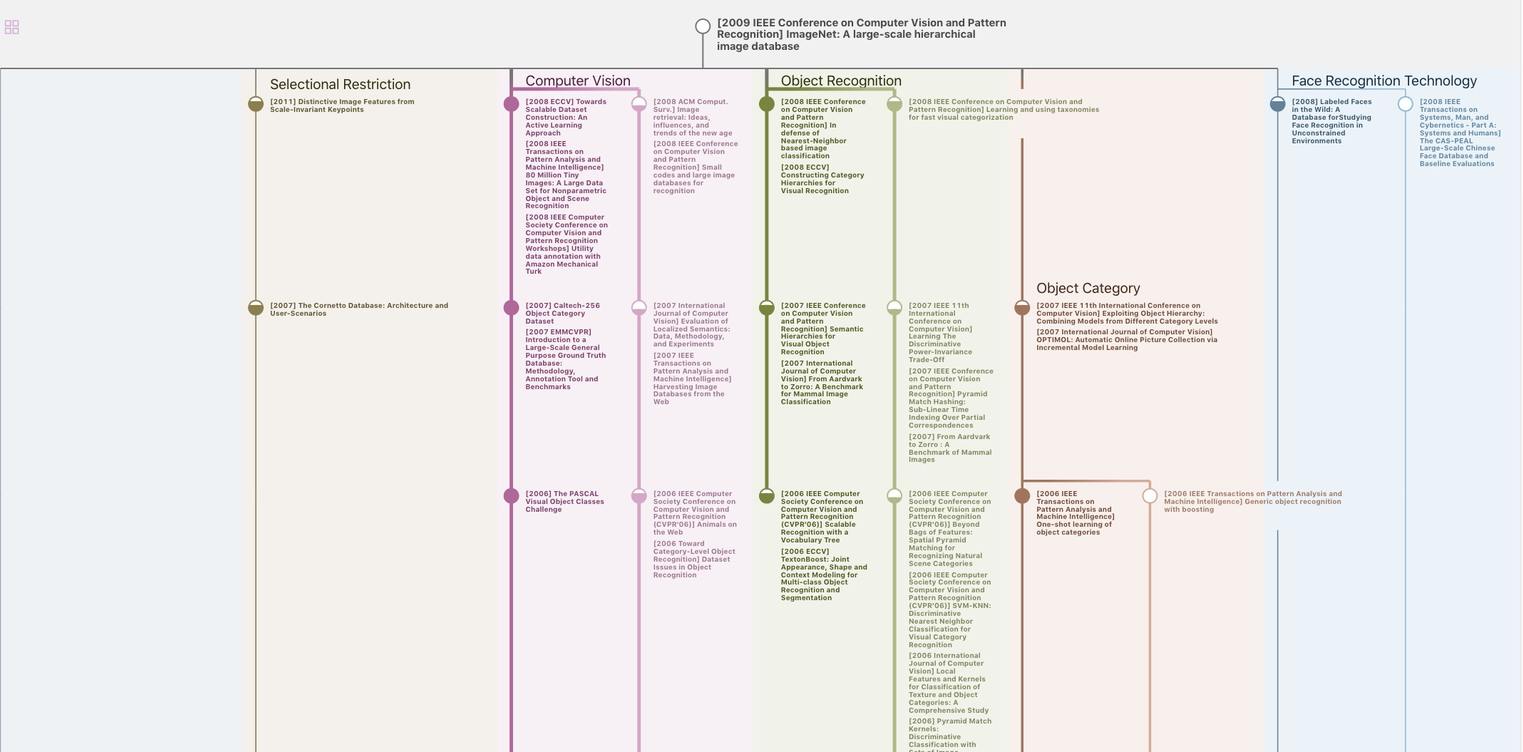
生成溯源树,研究论文发展脉络
Chat Paper
正在生成论文摘要