Bayesian Feature Selection for Radiomics Using Reliability Metrics
Frontiers in Genetics(2023)
关键词
Bayesian modeling,classification,quantitative imaging,probit prior,radiomics,variable selection
AI 理解论文
溯源树
样例
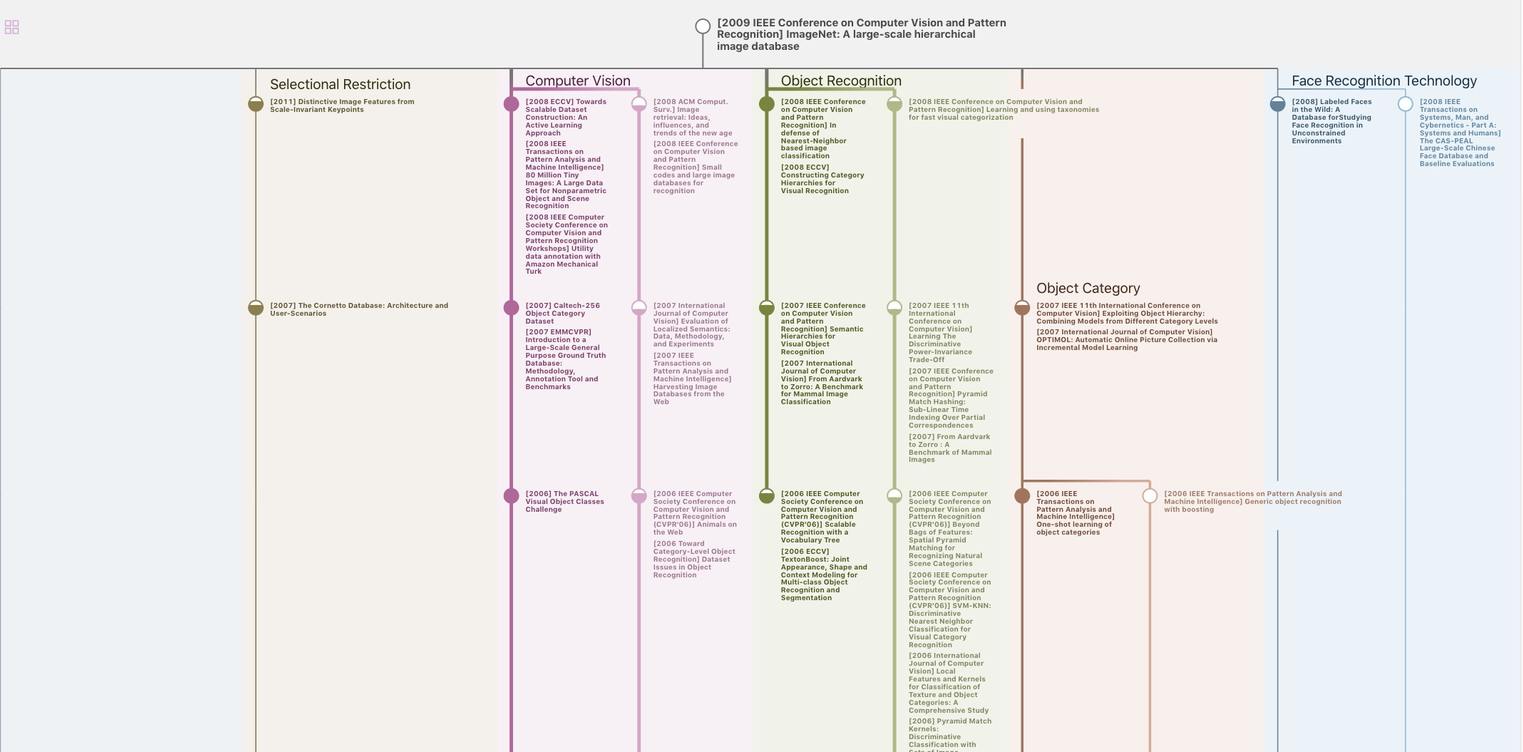
生成溯源树,研究论文发展脉络
Chat Paper
正在生成论文摘要
Frontiers in Genetics(2023)