TsSHAP: Robust model agnostic feature-based explainability for time series forecasting
arxiv(2023)
摘要
A trustworthy machine learning model should be accurate as well as explainable. Understanding why a model makes a certain decision defines the notion of explainability. While various flavors of explainability have been well-studied in supervised learning paradigms like classification and regression, literature on explainability for time series forecasting is relatively scarce. In this paper, we propose a feature-based explainability algorithm, TsSHAP, that can explain the forecast of any black-box forecasting model. The method is agnostic of the forecasting model and can provide explanations for a forecast in terms of interpretable features defined by the user a prior. The explanations are in terms of the SHAP values obtained by applying the TreeSHAP algorithm on a surrogate model that learns a mapping between the interpretable feature space and the forecast of the black-box model. Moreover, we formalize the notion of local, semi-local, and global explanations in the context of time series forecasting, which can be useful in several scenarios. We validate the efficacy and robustness of TsSHAP through extensive experiments on multiple datasets.
更多查看译文
关键词
explainability,robust model,feature-based
AI 理解论文
溯源树
样例
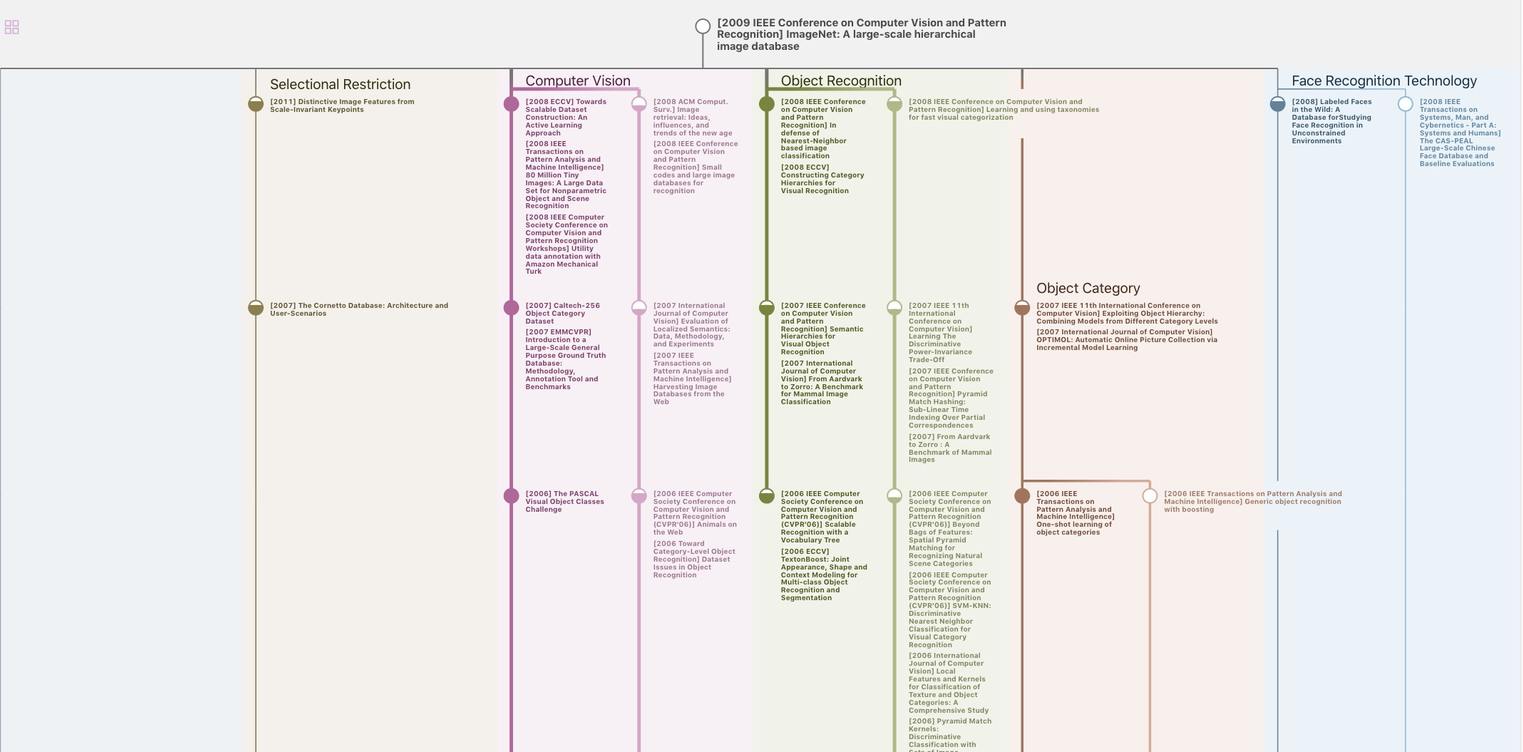
生成溯源树,研究论文发展脉络
Chat Paper
正在生成论文摘要