Predicting the Causative Pathogen among Children with Pneumonia Using a Causal Bayesian Network.
PLOS COMPUTATIONAL BIOLOGY(2023)
Key words
Childhood Pneumonia,Causal Discovery
AI Read Science
Must-Reading Tree
Example
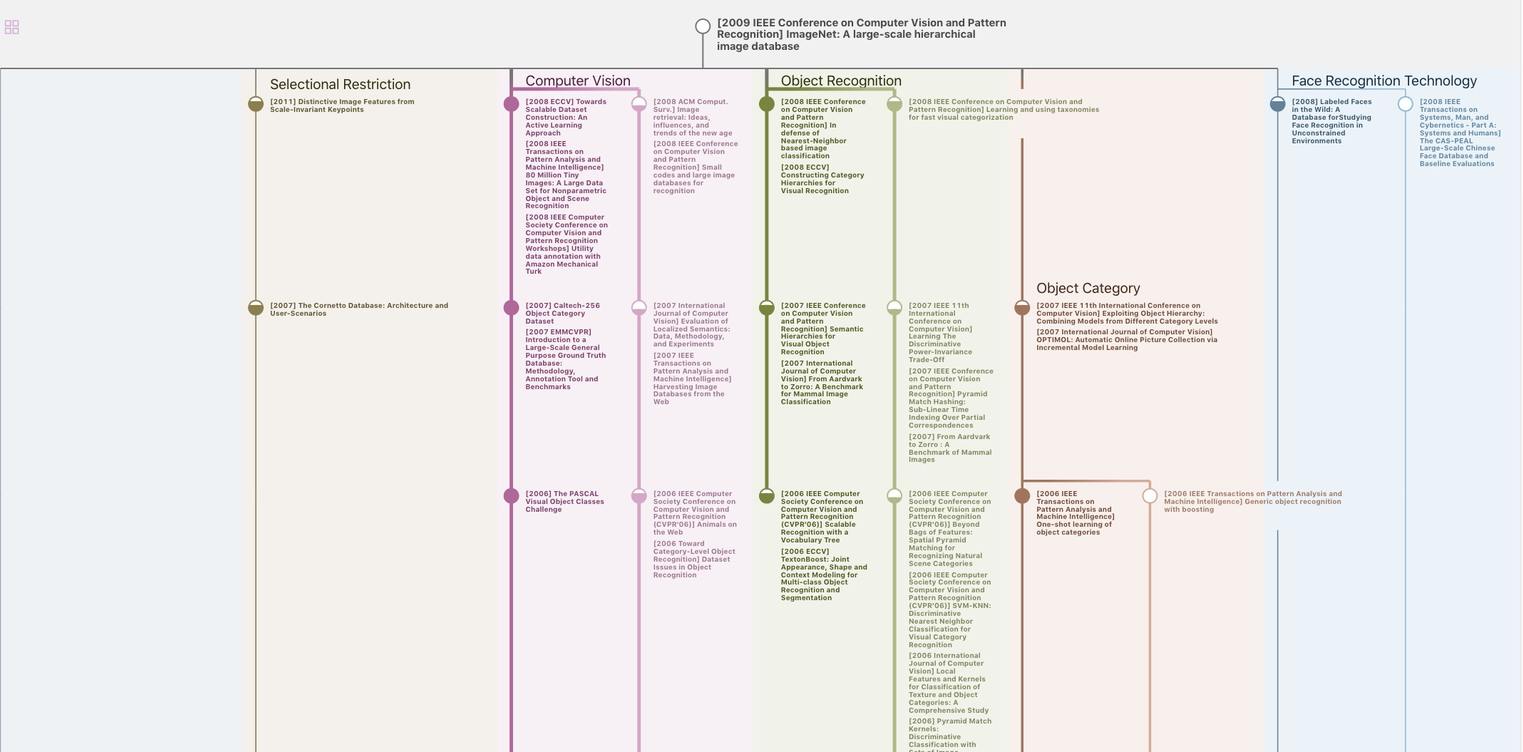
Generate MRT to find the research sequence of this paper
Chat Paper
Summary is being generated by the instructions you defined