AMSCN: A Novel Dual-Task Model for Automatic Modulation Classification and Specific Emitter Identification.
SENSORS(2023)
Key words
specific emitter identification (SEI),automatic modulation classification (AMC),deep learning,multitask learning
AI Read Science
Must-Reading Tree
Example
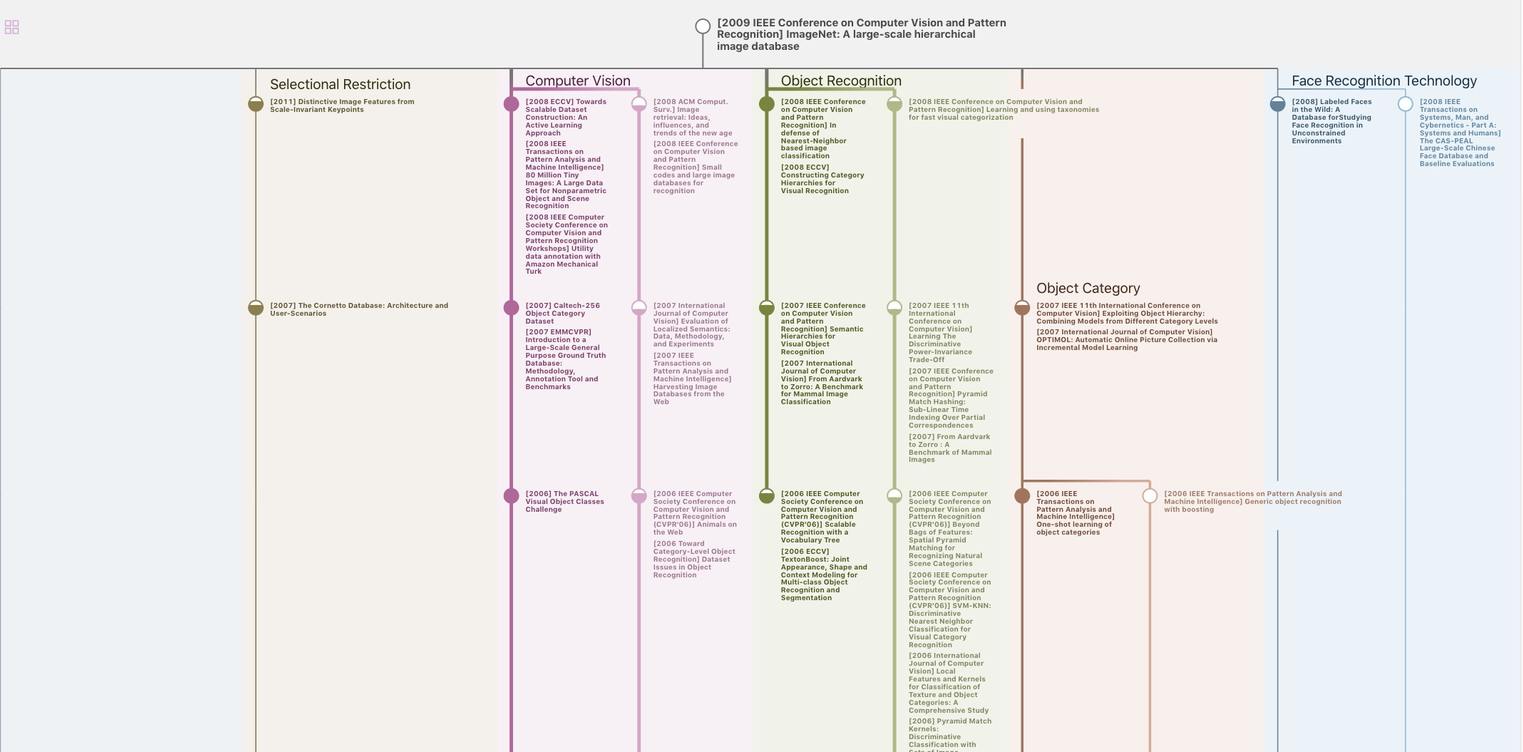
Generate MRT to find the research sequence of this paper
Chat Paper
Summary is being generated by the instructions you defined