Knowledge-embedded meta-learning model for lift coefficient prediction of airfoils
arxiv(2023)
摘要
Aerodynamic performance evaluation is an important part of the aircraft aerodynamic design optimization process; however, traditional methods are costly and time-consuming. Despite the fact that various machine learning methods can achieve high accuracy, their application in engineering is still difficult due to their poor generalization performance and "black box" nature. In this paper, a knowledge-embedded meta learning model, which fully integrates data with the theoretical knowledge of the lift curve, is developed to obtain the lift coefficients of an arbitrary supercritical airfoil under various angle of attacks. In the proposed model, a primary network is responsible for representing the relationship between the lift and angle of attack, while the geometry information is encoded into a hyper network to predict the unknown parameters involved in the primary network. Specifically, three models with different architectures are trained to provide various interpretations. Compared to the ordinary neural network, our proposed model can exhibit better generalization capability with competitive prediction accuracy. Afterward, interpretable analysis is performed based on the Integrated Gradients and Saliency methods. Results show that the proposed model can tend to assess the influence of airfoil geometry to the physical characteristics. Furthermore, the exceptions and shortcomings caused by the proposed model are analysed and discussed in detail.
更多查看译文
关键词
Lift coefficient,Knowledge-embedding,Meta-learning,Interpretability
AI 理解论文
溯源树
样例
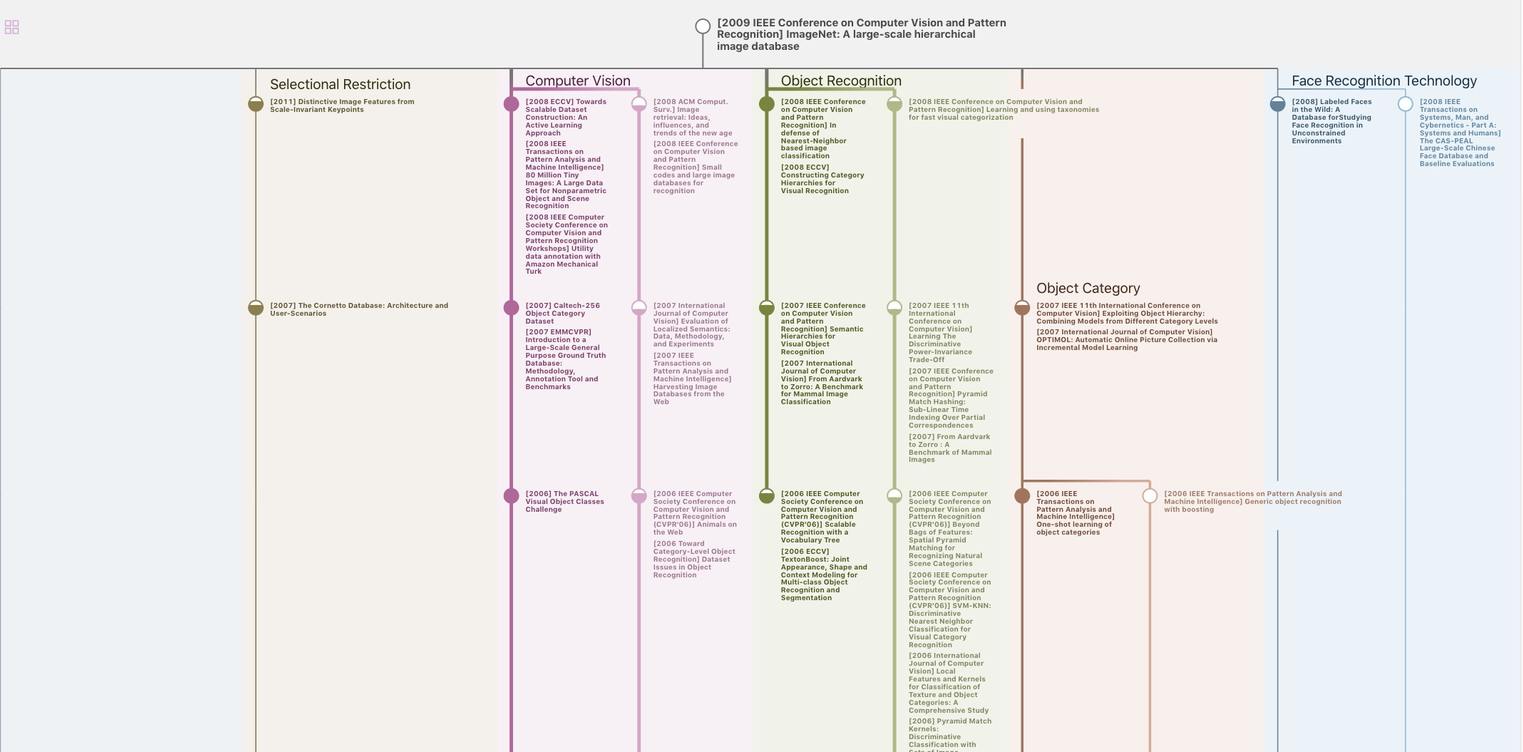
生成溯源树,研究论文发展脉络
Chat Paper
正在生成论文摘要