Targeting Tumor Heterogeneity: Multiplex-Detection-based Multiple Instance Learning for Whole Slide Image Classification.
Bioinformatics(2023)
AI Read Science
Must-Reading Tree
Example
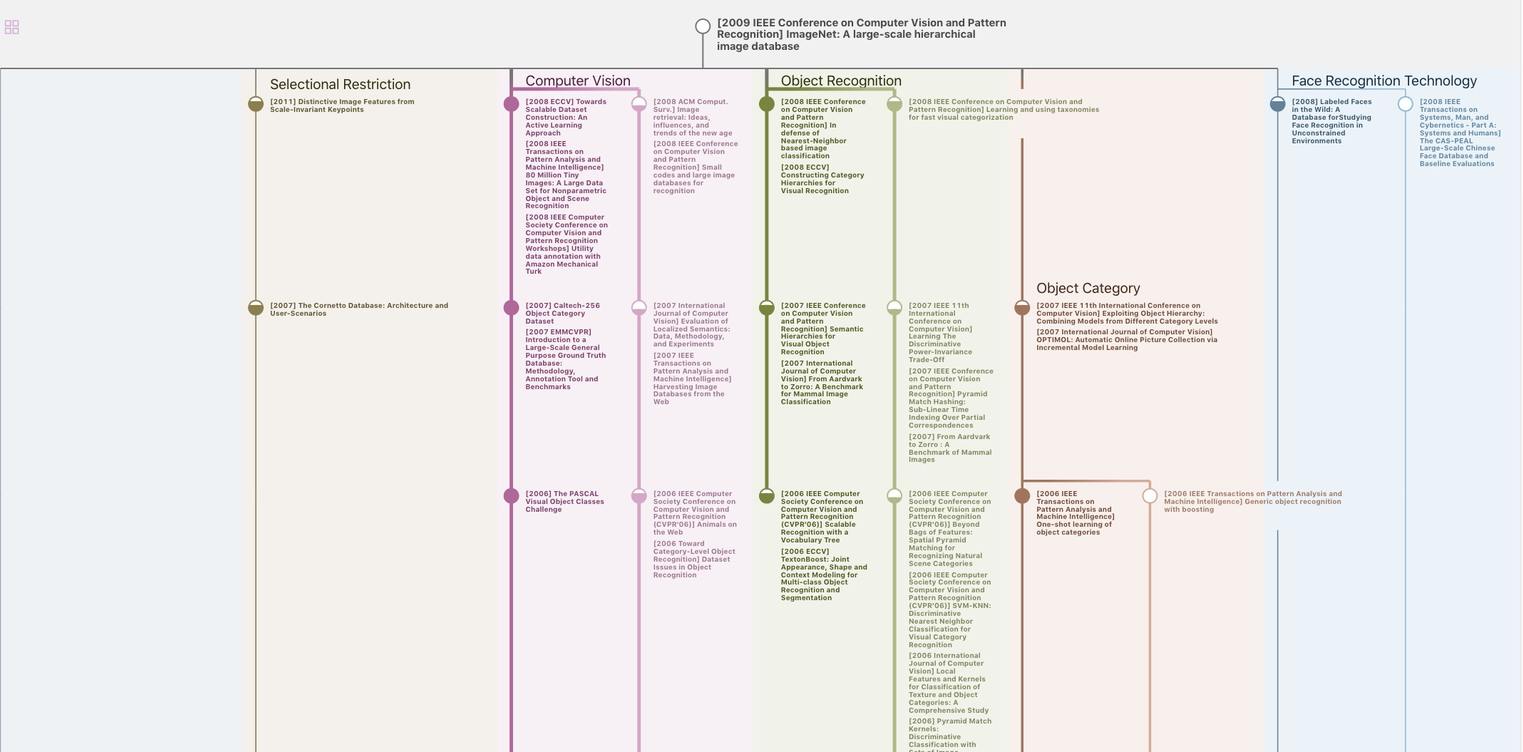
Generate MRT to find the research sequence of this paper
Chat Paper
Summary is being generated by the instructions you defined