Improving the Accuracy of the Water Detect Algorithm Using Sentinel-2, Planetscope and Sharpened Imagery: a Case Study in an Intermittent River
GISCIENCE & REMOTE SENSING(2023)
关键词
Remote sensing,water detection,sensitivity analysis,sharpening,hydrology
AI 理解论文
溯源树
样例
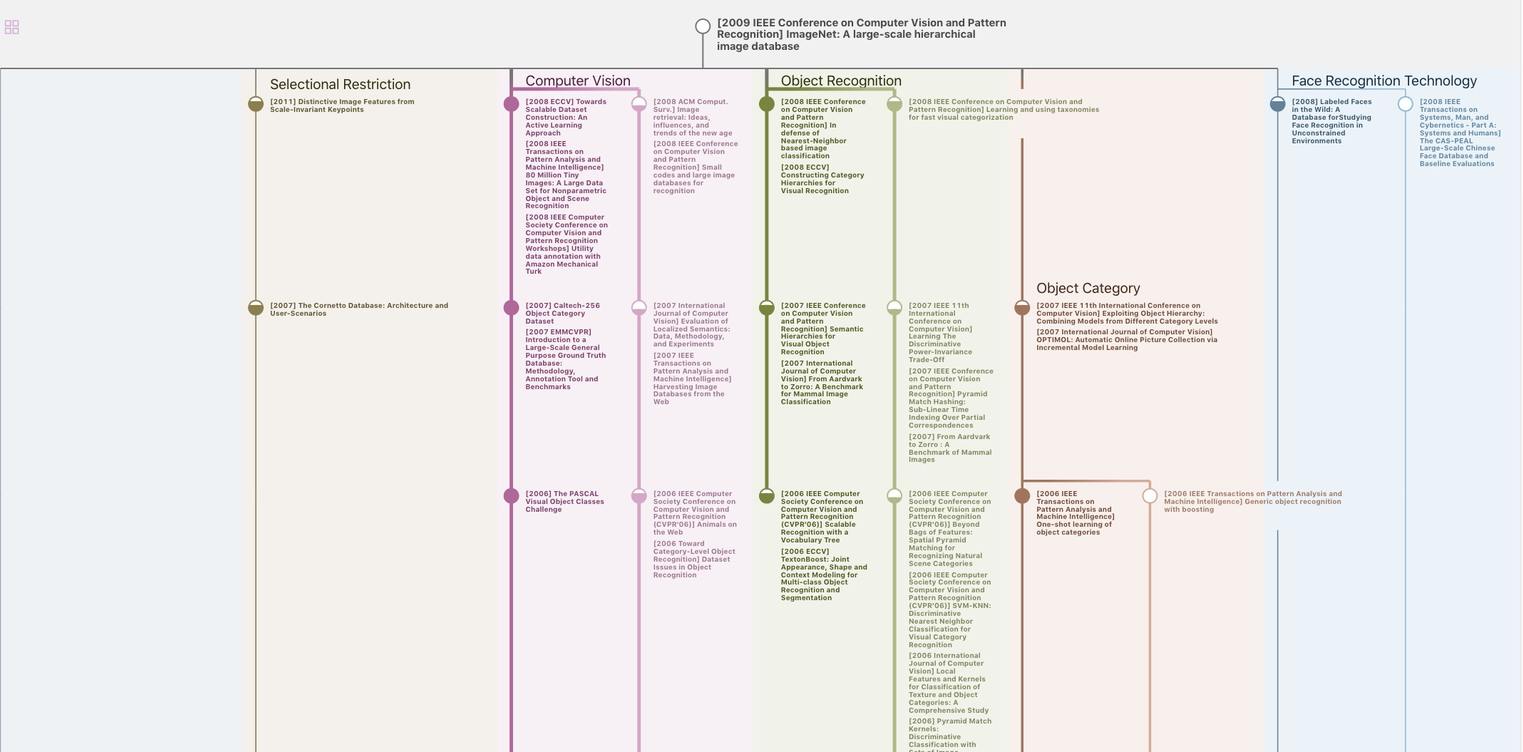
生成溯源树,研究论文发展脉络
Chat Paper
正在生成论文摘要