Prediction-based Variable Selection for Component-wise Gradient Boosting
INTERNATIONAL JOURNAL OF BIOSTATISTICS(2024)
Univ Goettingen
Abstract
Model-based component-wise gradient boosting is a popular tool for data-driven variable selection. In order to improve its prediction and selection qualities even further, several modifications of the original algorithm have been developed, that mainly focus on different stopping criteria, leaving the actual variable selection mechanism untouched. We investigate different prediction-based mechanisms for the variable selection step in model-based component-wise gradient boosting. These approaches include Akaikes Information Criterion (AIC) as well as a selection rule relying on the component-wise test error computed via cross-validation. We implemented the AIC and cross-validation routines for Generalized Linear Models and evaluated them regarding their variable selection properties and predictive performance. An extensive simulation study revealed improved selection properties whereas the prediction error could be lowered in a real world application with age-standardized COVID-19 incidence rates.
MoreTranslated text
Key words
gradient boosting,variable selection,prediction analysis,high-dimensional data,sparse models
PDF
View via Publisher
AI Read Science
Must-Reading Tree
Example
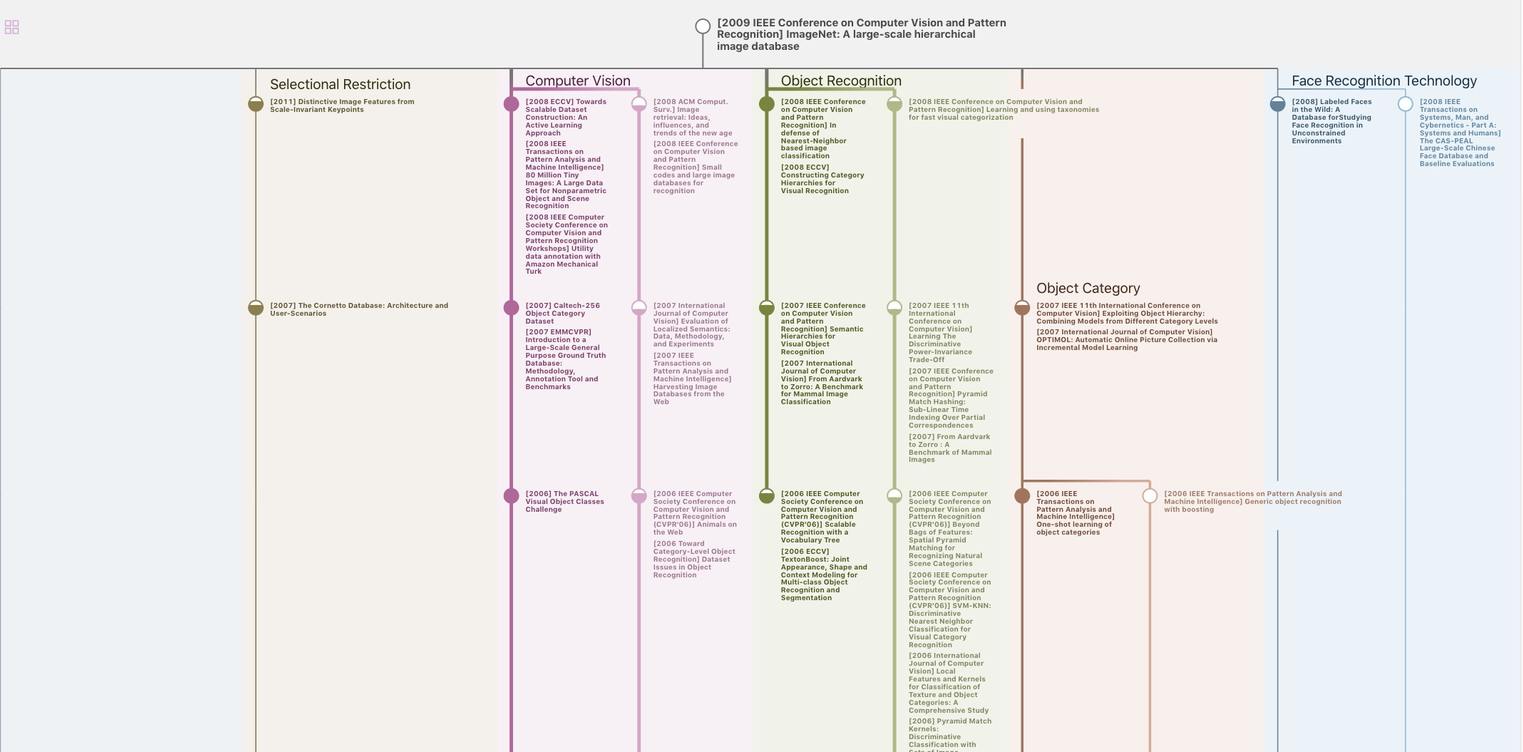
Generate MRT to find the research sequence of this paper
Data Disclaimer
The page data are from open Internet sources, cooperative publishers and automatic analysis results through AI technology. We do not make any commitments and guarantees for the validity, accuracy, correctness, reliability, completeness and timeliness of the page data. If you have any questions, please contact us by email: report@aminer.cn
Chat Paper
Summary is being generated by the instructions you defined