Application of Deep Learning in Microseismic Waveform Classification: A Case Study of the Yebatan Hydropower Station Project
2022 8th International Conference on Hydraulic and Civil Engineering Deep Space Intelligent Development and Utilization Forum (ICHCE)(2022)
Key words
Rock engineering,Microseismic monitoring,Deep learning,waveform classification,ACGAN
AI Read Science
Must-Reading Tree
Example
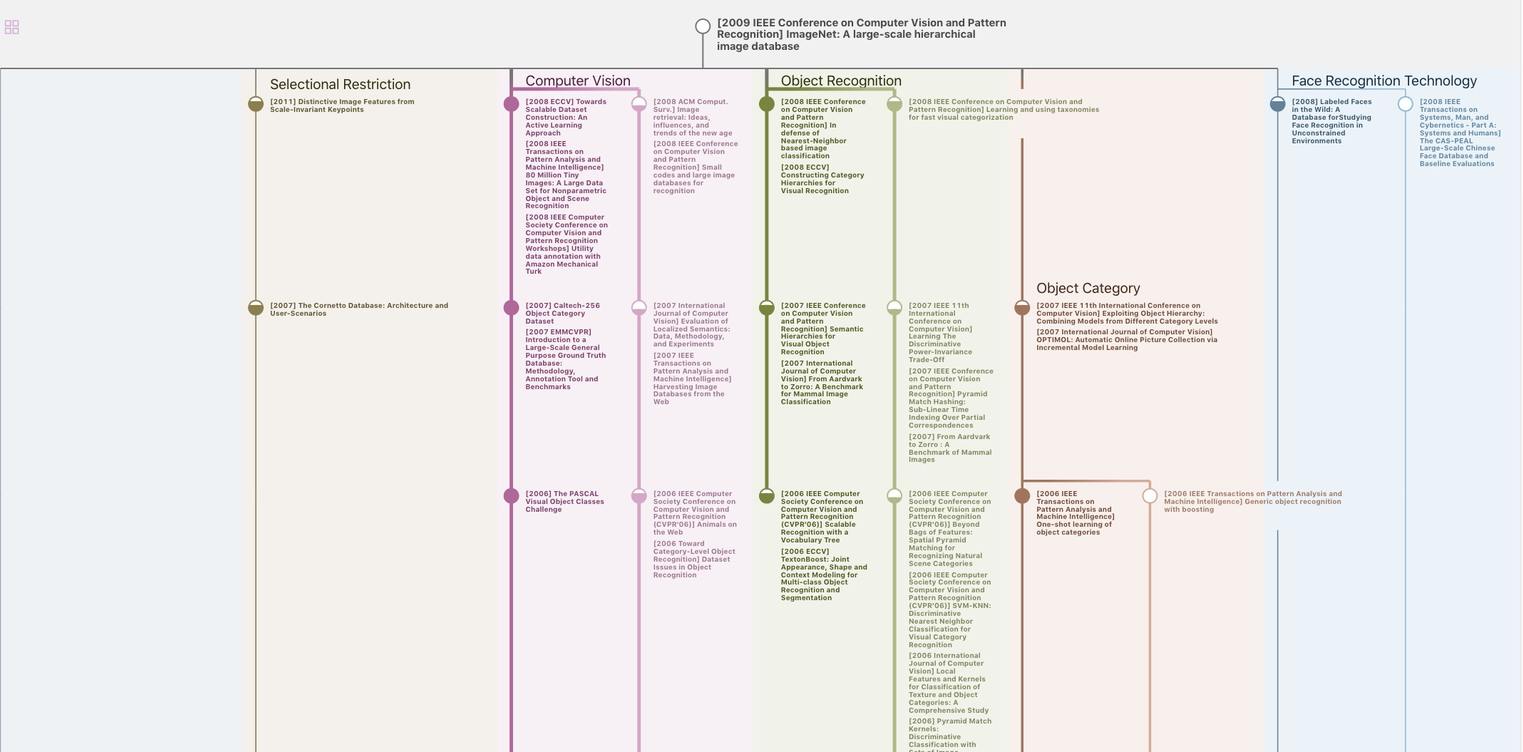
Generate MRT to find the research sequence of this paper
Chat Paper
Summary is being generated by the instructions you defined