Improving the Timing Resolution of Positron Emission Tomography Detectors Using Boosted Learning-A Residual Physics Approach
IEEE TRANSACTIONS ON NEURAL NETWORKS AND LEARNING SYSTEMS(2023)
摘要
Artificial intelligence (AI) is entering medical imaging, mainly enhancing image reconstruction. Nevertheless, improvements throughout the entire processing, from signal detection to computation, potentially offer significant benefits. This work presents a novel and versatile approach to detector optimization using machine learning (ML) and residual physics. We apply the concept to positron emission tomography (PET), intending to improve the coincidence time resolution (CTR). PET visualizes metabolic processes in the body by detecting photons with scintillation detectors. Improved CTR performance offers the advantage of reducing radioactive dose exposure for patients. Modern PET detectors with sophisticated concepts and read-out topologies represent complex physical and electronic systems requiring dedicated calibration techniques. Traditional methods primarily depend on analytical formulations successfully describing the main detector characteristics. However, when accounting for higher-order effects, additional complexities arise matching theoretical models to experimental reality. Our work addresses this challenge by combining traditional calibration with AI and residual physics, presenting a highly promising approach. We present a residual physics-based strategy using gradient tree boosting and physics-guided data generation. The explainable AI framework SHapley Additive exPlanations (SHAPs) was used to identify known physical effects with learned patterns. In addition, the models were tested against basic physical laws. We were able to improve the CTR significantly (more than 20%) for clinically relevant detectors of 19 mm height, reaching CTRs of 185 ps (450-550 keV).
更多查看译文
关键词
Coincidence time resolution (CTR),explainable artificial intelligence (XAI),gradient boosted decision tree (GBDT),positron emission tomography (PET),residual physics,time-of-flight (TOF)
AI 理解论文
溯源树
样例
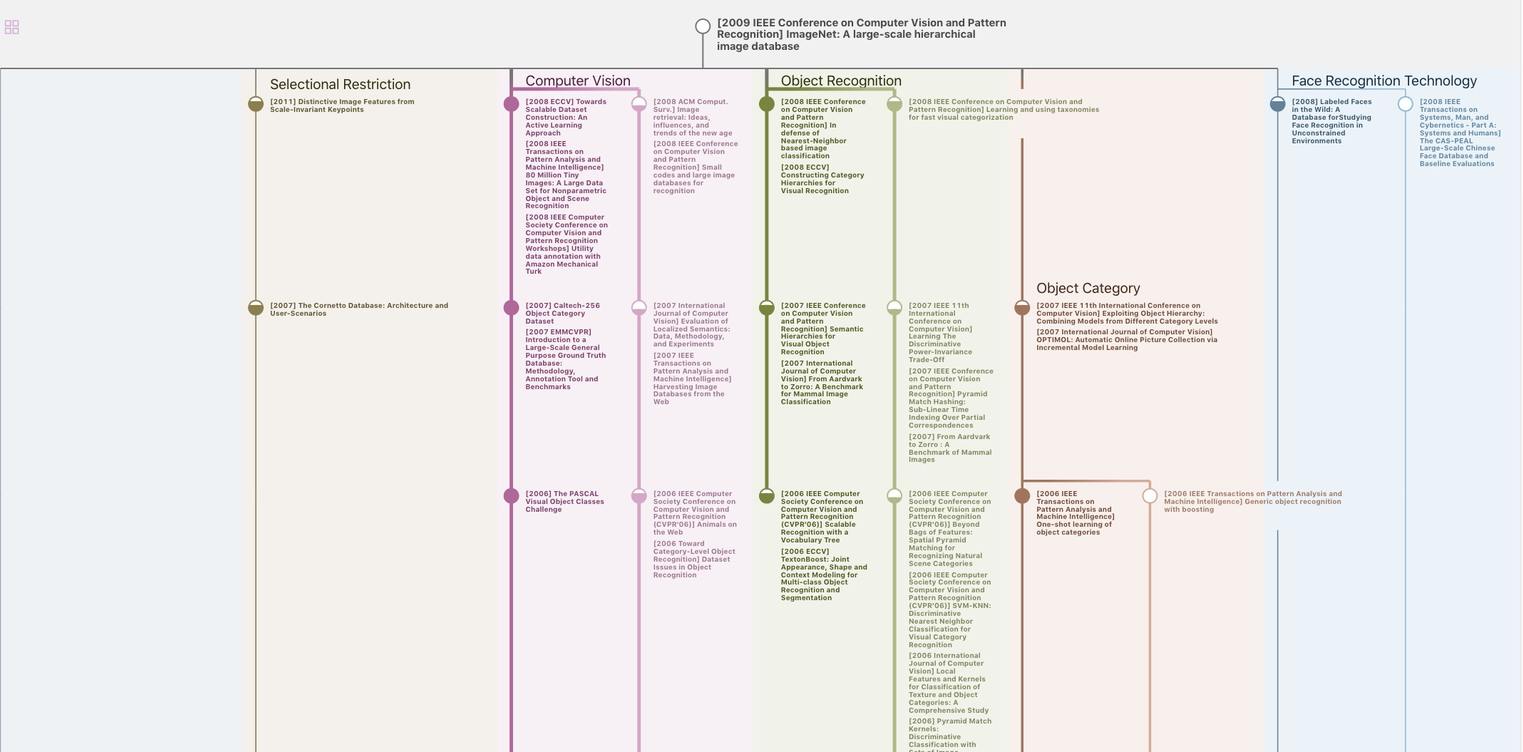
生成溯源树,研究论文发展脉络
Chat Paper
正在生成论文摘要