Imitating Human Behaviour with Diffusion Models
ICLR 2023(2023)
摘要
Diffusion models have emerged as powerful generative models in the text-to-image domain. This paper studies their application as observation-to-action models for imitating human behaviour in sequential environments. Human behaviour is stochastic and multimodal, with structured correlations between action dimensions. Meanwhile, standard modelling choices in behaviour cloning are limited in their expressiveness and may introduce bias into the cloned policy. We begin by pointing out the limitations of these choices. We then propose that diffusion models are an excellent fit for imitating human behaviour, since they learn an expressive distribution over the joint action space. We introduce several innovations to make diffusion models suitable for sequential environments; designing suitable architectures, investigating the role of guidance, and developing reliable sampling strategies. Experimentally, diffusion models closely match human demonstrations in a simulated robotic control task and a modern 3D gaming environment.
更多查看译文
关键词
imitation learning,behavioral cloning,behavioral cloning,diffusion models,generative models
AI 理解论文
溯源树
样例
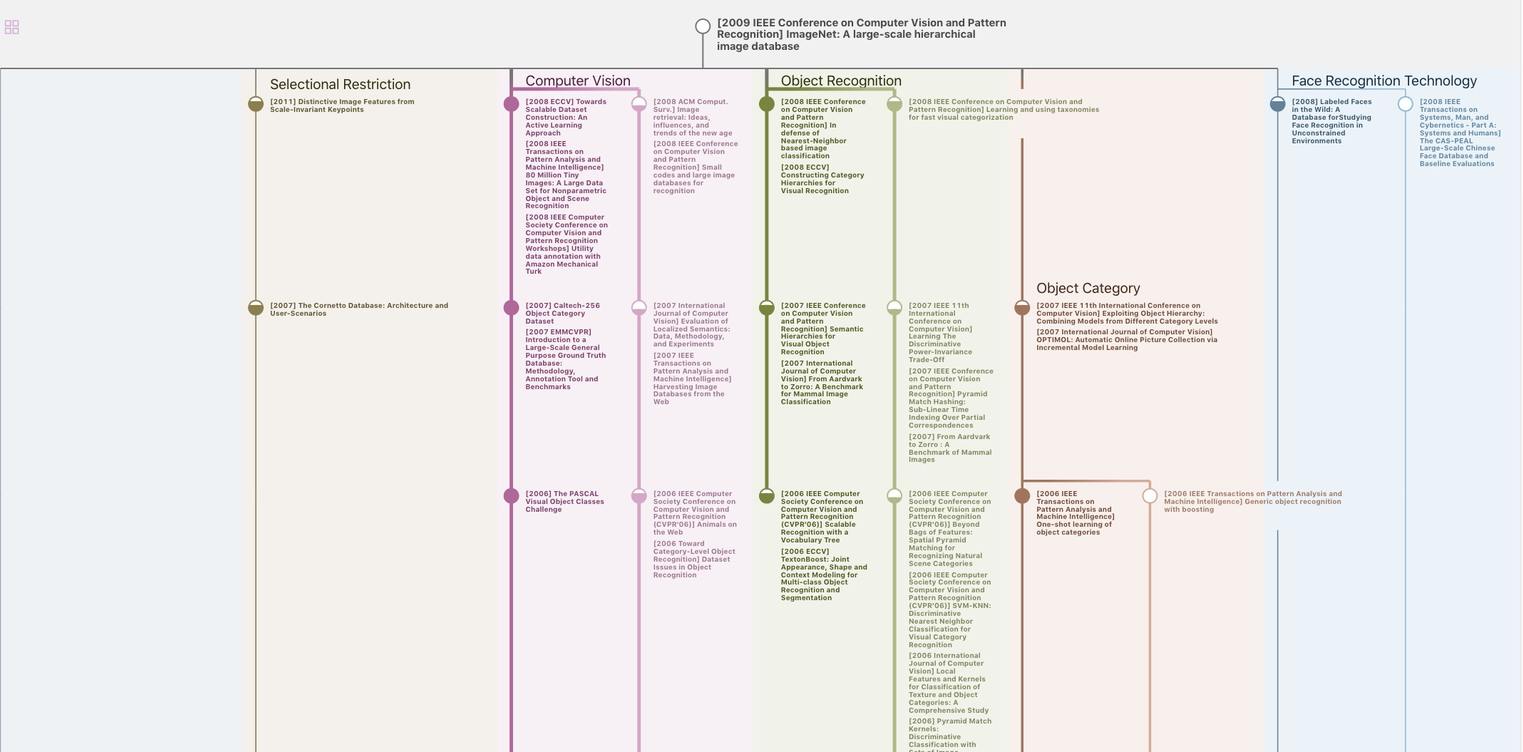
生成溯源树,研究论文发展脉络
Chat Paper
正在生成论文摘要