Active Reward Learning From Critiques
2018 IEEE INTERNATIONAL CONFERENCE ON ROBOTICS AND AUTOMATION (ICRA)(2018)
摘要
Learning from demonstration algorithms, such as Inverse Reinforcement Learning, aim to provide a natural mechanism for programming robots, but can often require a prohibitive number of demonstrations to capture important subtleties of a task. Rather than requesting additional demonstrations blindly, active learning methods leverage uncertainty to query the user for action labels at states with high expected information gain. However, this approach can still require a large number of labels to adequately reduce uncertainty and may also be unintuitive, as users are not accustomed to determining optimal actions in a single out-of-context state. To address these shortcomings, we propose a novel trajectory-based active Bayesian inverse reinforcement learning algorithm that 1) queries the user for critiques of automatically generated trajectories, rather than asking for demonstrations or action labels, 2) utilizes trajectory segmentation to expedite the critique / labeling process, and 3) predicts the user's critiques to generate the most highly informative trajectory queries. We evaluated our algorithm in simulated domains, finding it to compare favorably to prior work and a randomized baseline.
更多查看译文
关键词
critiques,active reward Learning,programming robots,active Bayesian inverse reinforcement learning,trajectory queries,labeling process,active learning
AI 理解论文
溯源树
样例
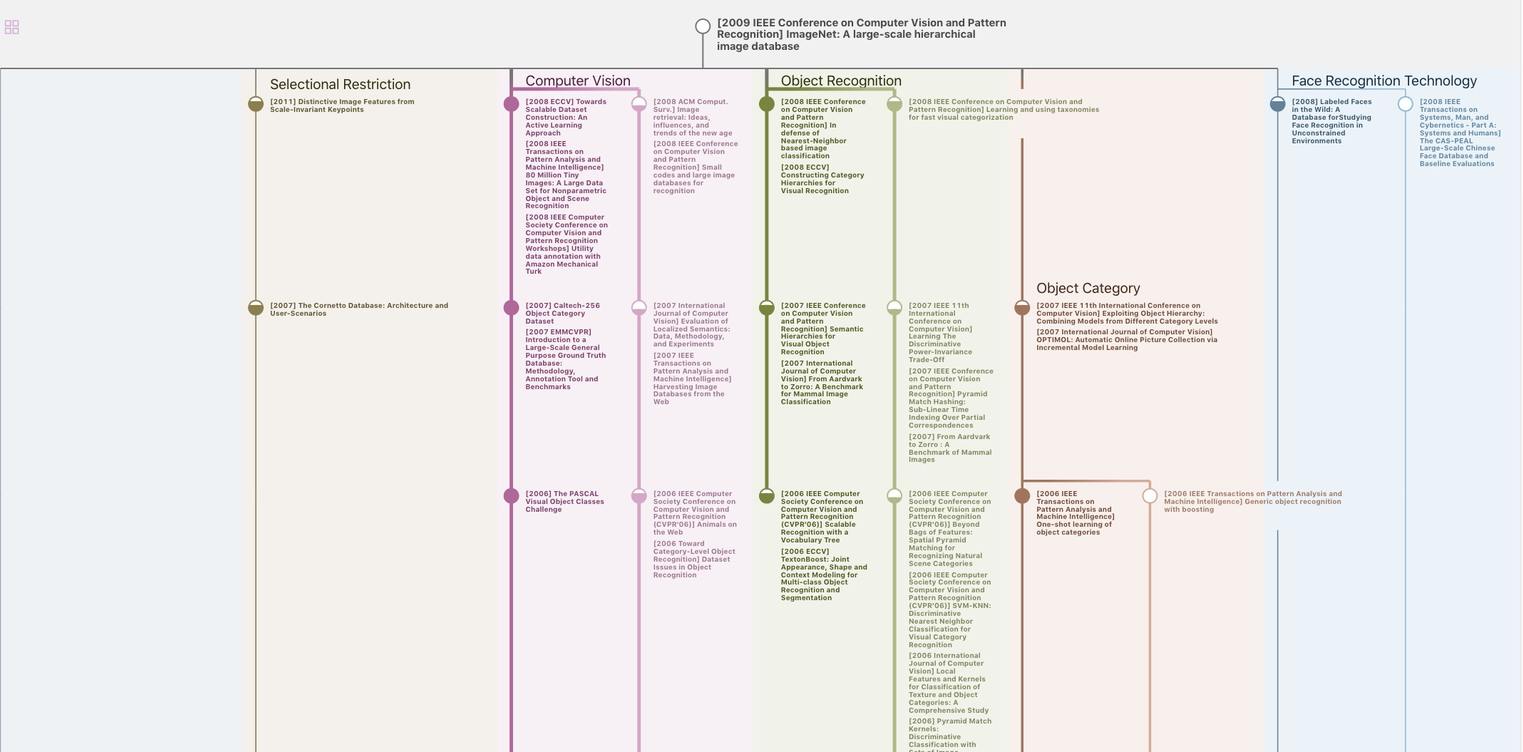
生成溯源树,研究论文发展脉络
Chat Paper
正在生成论文摘要